Fault Diagnosis of Oil‐Immersed Power Transformers Using SVM and Logarithmic Arctangent Transform
IEEJ transactions on electrical and electronic engineering(2022)
Abstract
A new method of dissolved gas analysis is proposed to improve the accuracy of transformer fault diagnosis. The slime mold optimized support vector machine (SMA‐SVM), and logarithmic arctangent transform (LOG‐ACT) are combined. On the one hand, the better global optimization performance of SMA is used to optimize SVM parameters to solve the difficulty of SVM parameter selection. On the other hand, corresponding transformations are carried out for different features: the logarithmic(LOG) transformation is carried out for the original DGA data to retain the order of magnitude information. The arctangent (ACT) transformation is carried out for the ratio features to improve the data structure. Therefore, the combination of data transformation and optimization model can improve the accuracy of diagnosis from two aspects of data structure and classification algorithm. The performance of the proposed method was compared with IEC three ratio method, artificial neural network, optimized artificial neural network, GA‐SVM, and PSO‐SVM. Experimental results using published data show that the proposed method can significantly improve the accuracy of transformer fault diagnosis. © 2022 Institute of Electrical Engineers of Japan. Published by Wiley Periodicals LLC.
MoreTranslated text
Key words
dissolved gas analysis (DGA),support vector machine,slime molds algorithm,logarithmic transform,arctangent transform
AI Read Science
Must-Reading Tree
Example
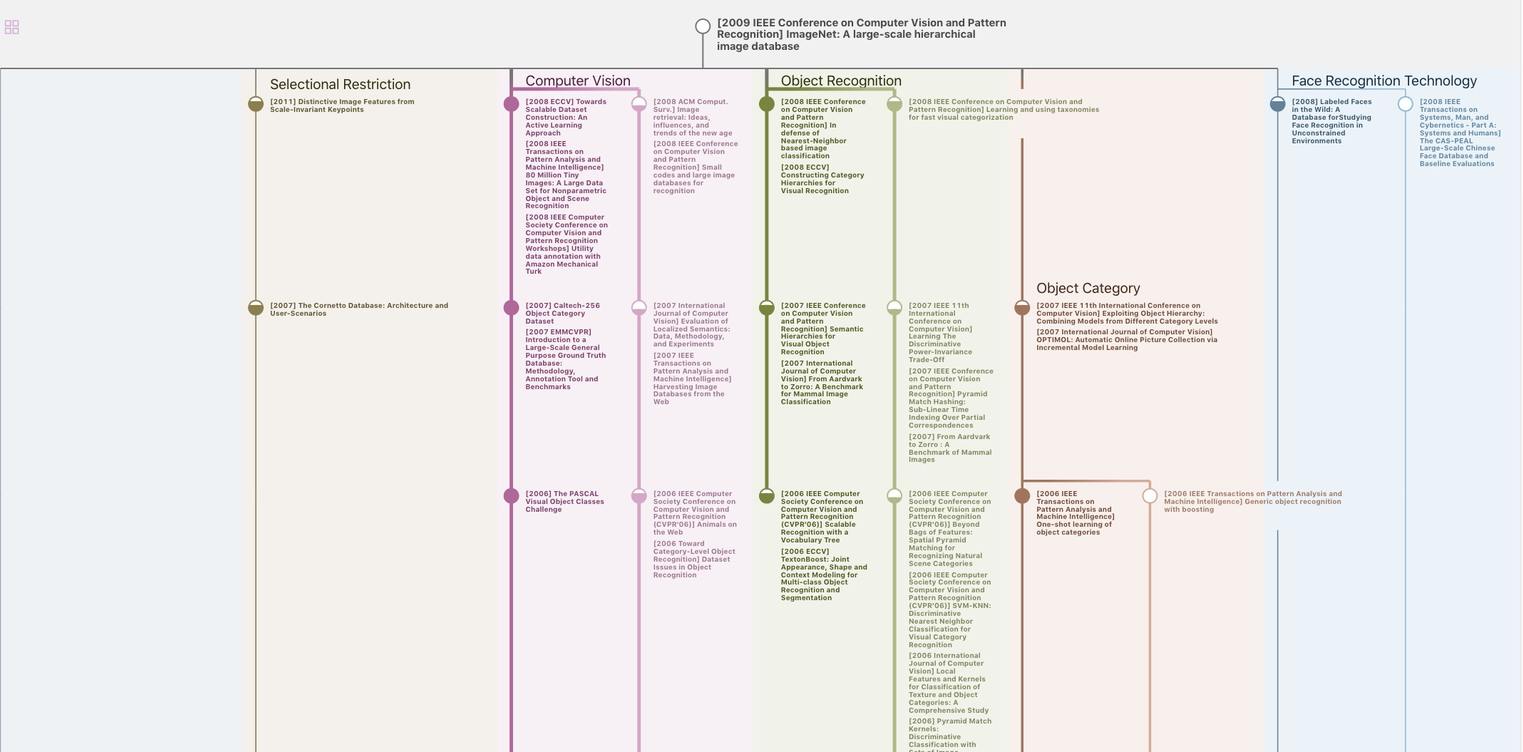
Generate MRT to find the research sequence of this paper
Chat Paper
Summary is being generated by the instructions you defined