The Implementation of Multiobjective Flexible Workshop Scheduling Based on Genetic Simulated Annealing-Inspired Clustering Algorithm
WIRELESS COMMUNICATIONS & MOBILE COMPUTING(2022)
摘要
Multiobjective flexible workshop scheduling is an important subject to improve resource utilization and production efficiency and enhance the competitiveness of enterprises. As the situation of resource constraints becomes more and more severe, the problem of companies rationally allocating limited resources in production is becoming more and more serious. Today, the manufacturing industry widely adopts advanced manufacturing modes such as computer-integrated manufacturing and intelligent manufacturing, but in these semi-intelligent manufacturing modes with a high degree of uncertainty and a high degree of personnel dependence, it is difficult to adapt to the work of large-scale production. Therefore, suitable clustering algorithms are urgently needed to help solve these problems, and this paper selects a clustering algorithm based on the genetic simulation annealing algorithm. This article is aimed at studying the problem of efficiency improvement in the production process of large-scale manufacturing and at finding a stronger and more effective production mode for the manufacturing industry. Firstly, this paper introduces the basic principles of simulated annealing genetic algorithm and regularized clustering algorithm. These algorithms have excellent performance in searching for global optimal solutions. They can be constantly tested and computed to keep the calculation results close to the global optimal solution. In this paper, the K-means clustering algorithm is used to select the shortest completion time to represent the clustering target. According to the minimum distance principle, the machine, workpiece, and other objects are input into the clustering of the algorithm, and the K-means algorithm will send out the sorting plan. Therefore, a multiobjective flexible job shop scheduling model based on genetic simulated annealing algorithm and clustering algorithm is established. Then, by using hypothetical production data to simulate the operation of the workshop, the scheduling model was applied to conduct a deduction and empirical comparative study. The experimental results showed that the model shortened the completion time of the workpiece by 4.4% and increased the average load rate of the machine by 10%.
更多查看译文
AI 理解论文
溯源树
样例
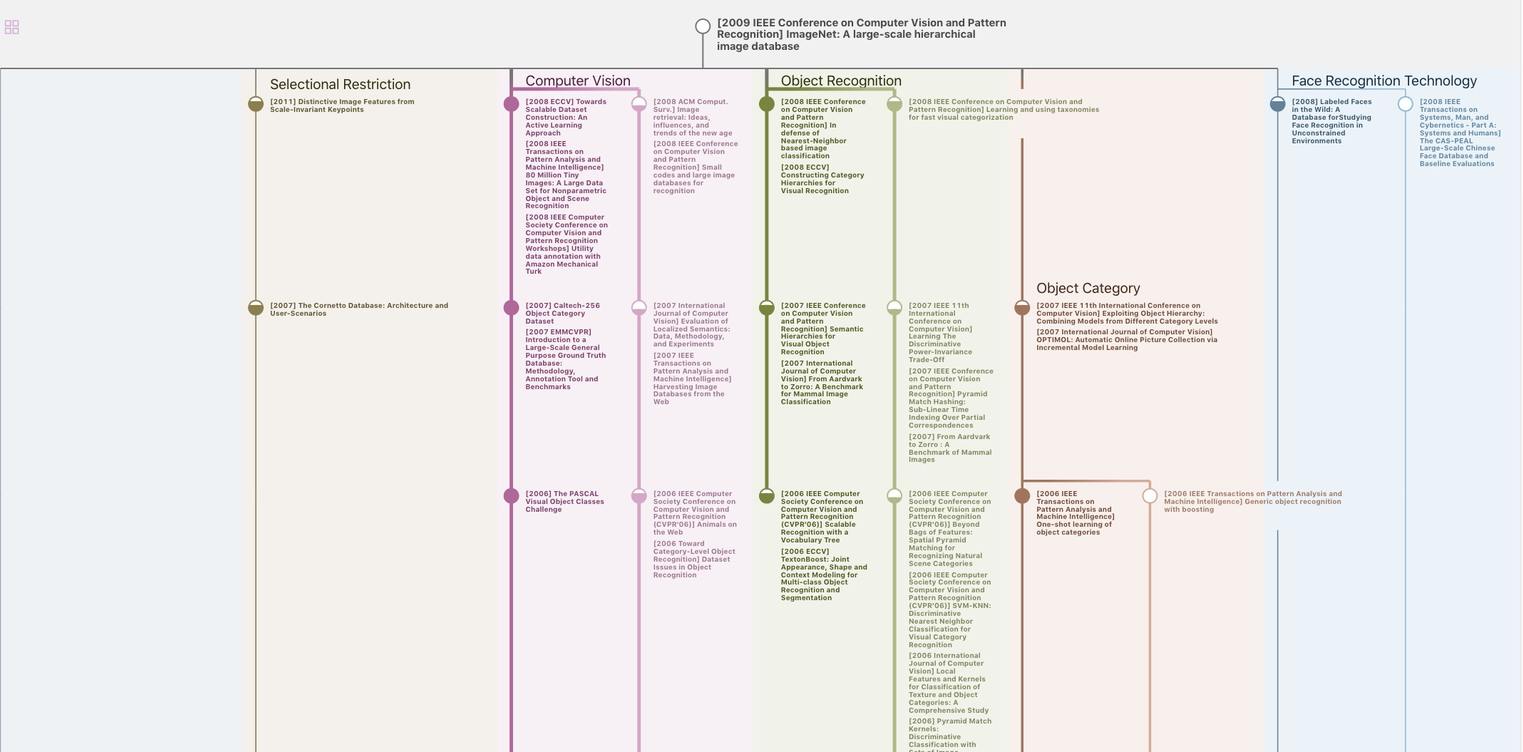
生成溯源树,研究论文发展脉络
Chat Paper
正在生成论文摘要