Spiking VGG7: Deep Convolutional Spiking Neural Network with Direct Training for Object Recognition
ELECTRONICS(2022)
摘要
We propose a deep convolutional spiking neural network (DCSNN) with direct training to classify concrete bridge damage in a real engineering environment. The leaky-integrate-and-fire (LIF) neuron model is employed in our DCSNN that is similar to VGG. Poisson encoding and convolution encoding strategies are considered. The gradient surrogate method is introduced to realize the supervised training for the DCSNN. In addition, we have examined the effect of observation time step on the network performance. The testing performance for two different spike encoding strategies are compared. The results show that the DCSNN using gradient surrogate method can achieve a performance of 97.83%, which is comparable to traditional CNN. We also present a comparison with STDP-based unsupervised learning and a converted algorithm, and the proposed DCSNN is proved to have the best performance. To demonstrate the generalization performance of the model, we also use a public dataset for comparison. This work paves the way for the practical engineering applications of the deep SNNs.
更多查看译文
关键词
deep convolutional spiking neural networks,surrogate gradient,bridge damage detection
AI 理解论文
溯源树
样例
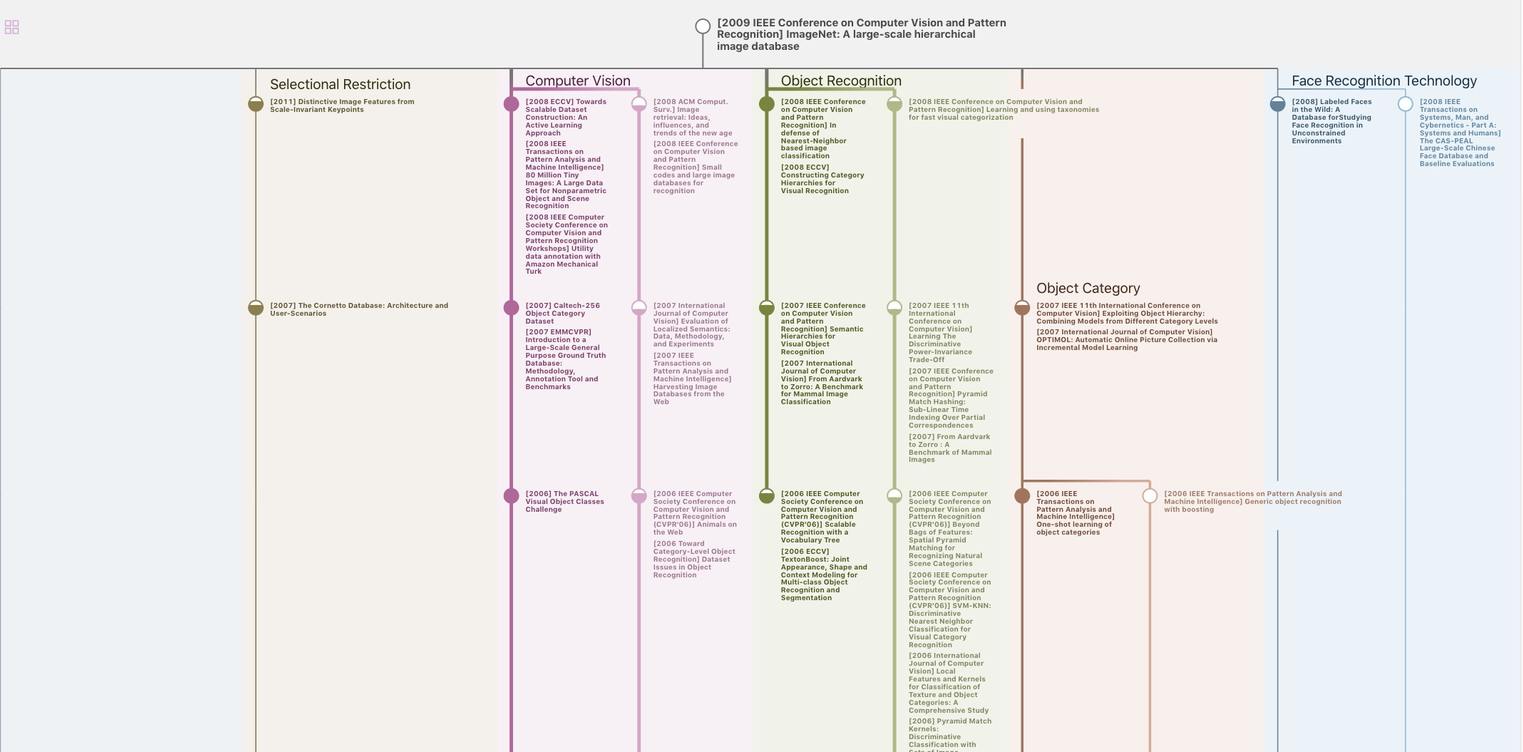
生成溯源树,研究论文发展脉络
Chat Paper
正在生成论文摘要