A DEEP-LEARNING FRAMEWORK FOR ACCURATE AND ROBUST DETECTION OF ADULT CONTENT
JOURNAL OF ENGINEERING SCIENCE AND TECHNOLOGY(2022)
摘要
Video streaming services has dominated the growth of Internet traffic, which accounts for more than 82% of global network traffic. As videos represent a powerful medium for user engagement, there has been an exponential growth in the different forms of video content being generated and distributed. Such a large diversity of content includes educational, gaming, historical, and entertainment among others. While the positive impact of these video services available through Internet has benefitted a large number of citizens, it is evident that the same platform has been subjected to misuse for the propagation of explicit content not suitable for children. Therefore, it is crucial to develop automated solutions that can successfully filter such content to protect children and vulnerable individuals. Over the last few years, deep learning algorithms have achieved high accuracy in object recognition in comparison to the statistical algorithms trained on hand-crafted features. The deep-learning algorithms has demonstrated the ability to automatically select most representative features to be extracted from visual representation of objects. While this technology has been successfully applied to many important computer vision problems, its use for the classification and filtering of adult content has not been fully explored yet. Addressing this challenge, the research presented in this paper reports a deep-learning framework that exploits spatio-temporal and visual features of video sequences for efficient and effective detection of adult content. The proposed network architecture aims at harvesting information from two important aspects of video content: spatial self learned features and cues from temporal redundancies and dynamics in videos. First, a Convolutional Neural Network (CNN) architecture based on Inception-v3 is used to model and learn the spatial video features. This CNN architecture builds the basis of the proposed deep-learning framework. Temporal characteristics are then modelled through a long-term short memory approach that correlates information from subsequent frames in the processed video clip. The proposed approach has been validated against an openly available dataset, which includes a large category of adult content and other video sequences that are hard to discriminate, e.g., beach footage, swimming and wrestling. The accuracy of the proposed approach reaches 97.4%, while the recall is 97%, making it highly suitable for practical applications.
更多查看译文
关键词
Adult content, Convolutional neural network, NPDI dataset, Video classification, LSTM network
AI 理解论文
溯源树
样例
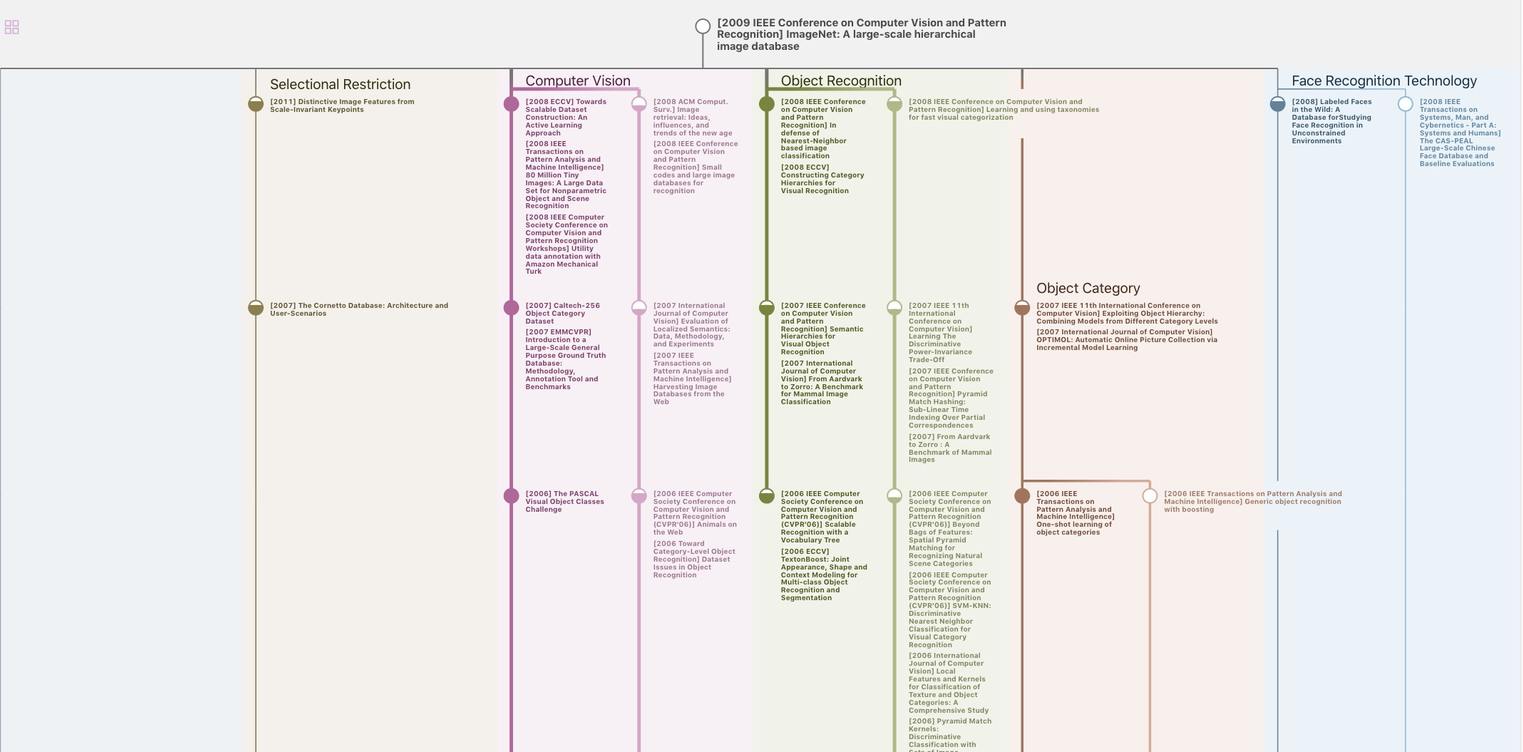
生成溯源树,研究论文发展脉络
Chat Paper
正在生成论文摘要