Road damage detection using super-resolution and semi-supervised learning with generative adversarial network
AUTOMATION IN CONSTRUCTION(2022)
摘要
Road maintenance technology is required to maintain favorable driving conditions and prevent accidents. In particular, a sensor technology is required for detecting road damage. In this study, we developed a new sensor technology that can detect road damage using a deep learning-based image processing algorithm. The proposed technology includes a super-resolution and semi-supervised learning method based on a generative adversarial network. The former improves the quality of the road image to make the damaged area clearly visible. The latter enhances the detection performance using 5327 road images and 1327 label images. These two methods were applied to four lightweight segmentation neural networks. For 400 road images, the average recognition performance was 81.540% and 79.228% in terms of the mean intersection over union and F1-score, respectively. Consequently, the proposed training method improves the road damage detection algorithm and can be used for efficient road management in the future.
更多查看译文
关键词
Road damage detection, Image processing, Super resolution, GAN, Semi-supervised learning
AI 理解论文
溯源树
样例
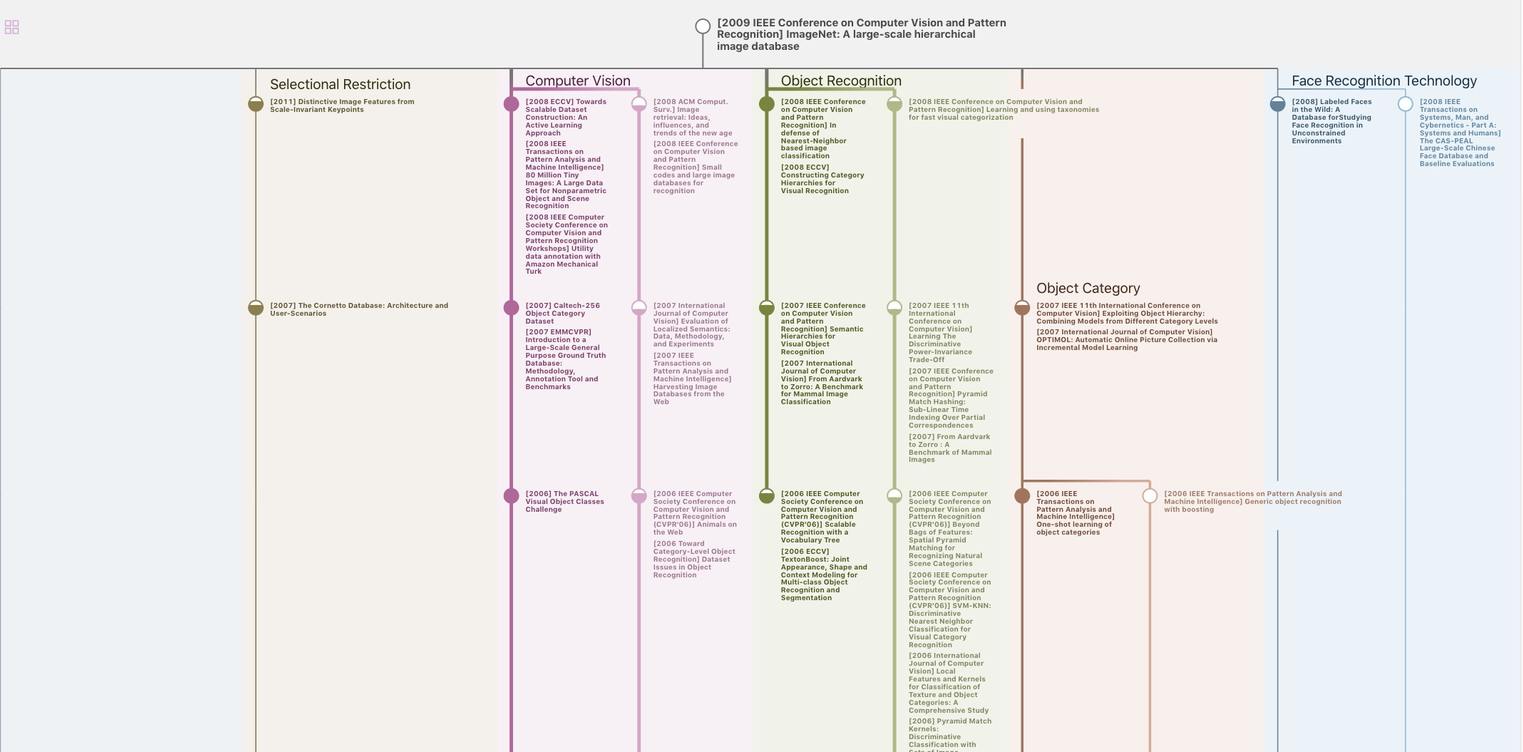
生成溯源树,研究论文发展脉络
Chat Paper
正在生成论文摘要