Development of an operation trajectory design algorithm for control of multiple 0D parameters using deep reinforcement learning in KSTAR
NUCLEAR FUSION(2022)
摘要
This work develops an artificially intelligent (AI) tokamak operation design algorithm that provides an adequate operation trajectory to control multiple plasma parameters simultaneously into different targets. An AI is trained with the reinforcement learning technique in the data-driven tokamak simulator, searching for the best action policy to get a higher reward. By setting the reward function to increase as the achieved beta (p), q (95), and l (i) are close to the given target values, the AI tries to properly determine the plasma current and boundary shape to reach the given targets. After training the AI with various targets and conditions in the simulation environment, we demonstrated that we could successfully achieve the target plasma states with the AI-designed operation trajectory in a real KSTAR experiment. The developed algorithm would replace the human task of searching for an operation setting for given objectives, provide clues for developing advanced operation scenarios, and serve as a basis for the autonomous operation of a fusion reactor.
更多查看译文
关键词
machine learning, reinforcement learning, tokamak control, tokamak operation scenario, artificial intelligence (AI), nuclear fusion, operation design algorithm
AI 理解论文
溯源树
样例
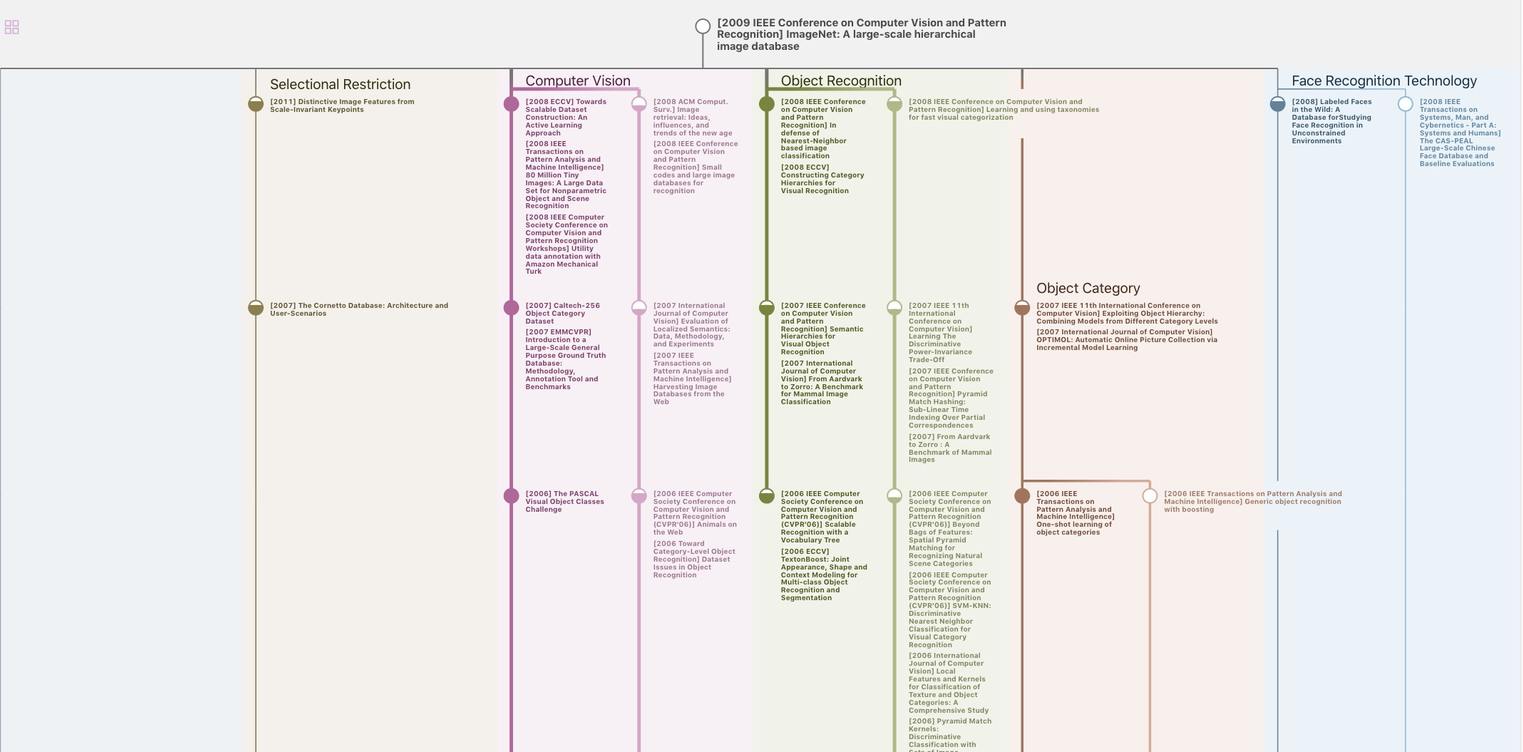
生成溯源树,研究论文发展脉络
Chat Paper
正在生成论文摘要