Scalable Wireless Anomaly Detection with Generative-LSTMs on RF Post-Detection Metadata
2022 IEEE WIRELESS COMMUNICATIONS AND NETWORKING CONFERENCE (WCNC)(2022)
摘要
Signal anomaly detection is commonly used to detect rogue or unexpected signals. It has many applications in interference mitigation, wireless security, optimized spectrum allocation, and radio coordination. Our work proposes a new method for anomaly detection on signal detection metadata using generative adversarial network output processed by a long short term memory recurrent neural network. We provide a performance analysis and comparison to baseline methods, and demonstrate that through the usage of metadata for analytics, we can provide robust detection, while also minimizing computation and bandwidth, and generalizing to numerous effects which differs from many prior works that focus on A.D. based signal processing on the raw RF sample data.
更多查看译文
关键词
Signal Processing, Anomaly Detection, Wireless, Elasticsearch, Metadata, Omnisig, Sensing, RF, Analytics, Edge Intelligence, Wireless Security
AI 理解论文
溯源树
样例
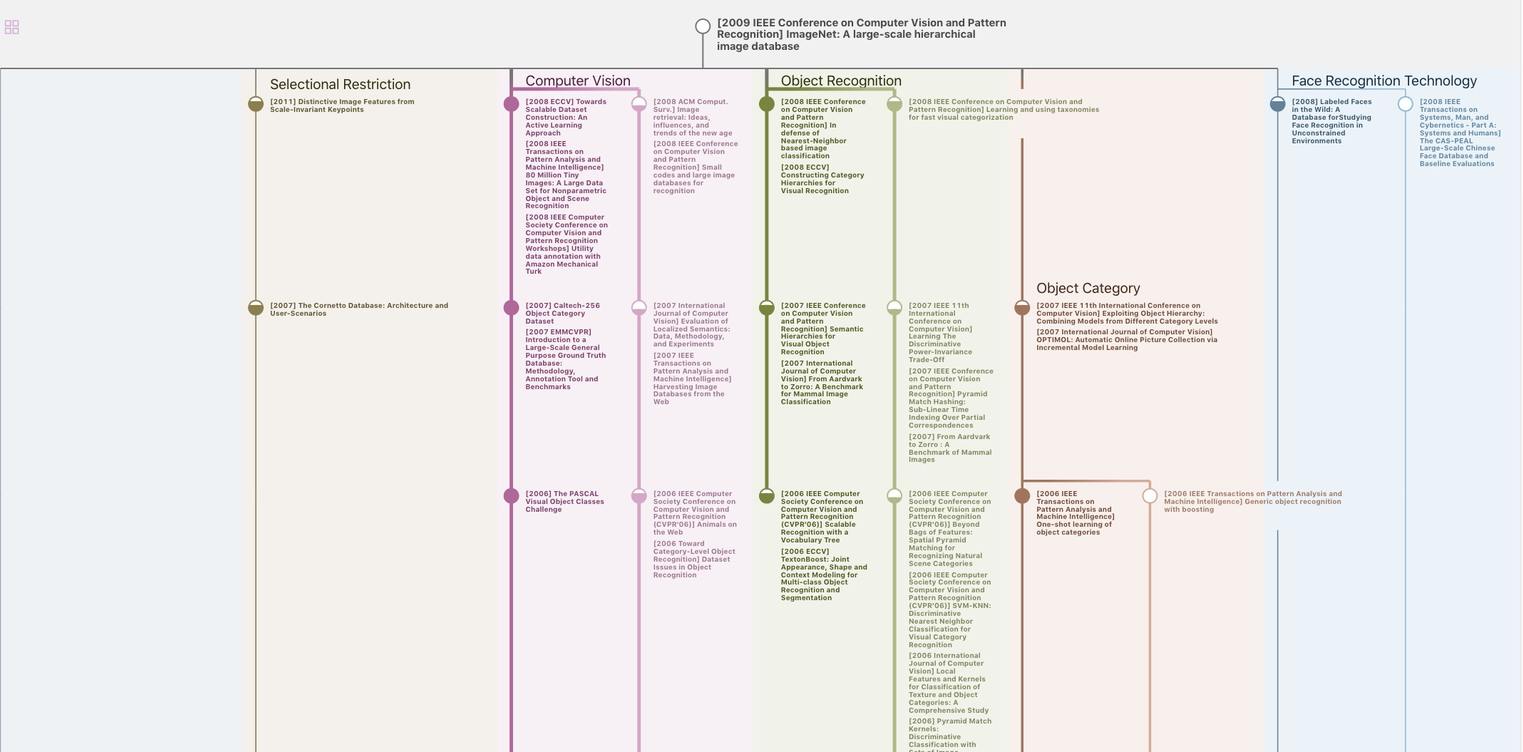
生成溯源树,研究论文发展脉络
Chat Paper
正在生成论文摘要