Delay Estimation in Dense Multipath Environments using Time Series Segmentation
2022 IEEE WIRELESS COMMUNICATIONS AND NETWORKING CONFERENCE (WCNC)(2022)
摘要
Channel measurements at sufficiently high bandwidth in multipath-rich environments include a variety of delay information, which, if accurately extracted, can be exploited for accurate positioning. While previous methods are limited in practice as they rely on iteratively extracting a fixed number of delays, we instead formulate the delay extraction problem as a time series segmentation task. For this, we propose a pipeline built upon the U-Net convolutional neural network architecture. Unlike the state of the art our pipeline extracts an arbitrary number of delays without prior knowledge, includes a threshold for weighting between detection rate and false alarms, and does not rely on computationally demanding operations such as eigenvalue decomposition. We evaluate the presented method with synthetic data of different noise configurations and signal bandwidths and a publicly available dataset, achieving considerable performance gains w.r.t. detection performance and tracking accuracy. Furthermore, we show that the proposed method is far less computationally demanding in inference.
更多查看译文
关键词
Delay estimation, U-Net, deep learning
AI 理解论文
溯源树
样例
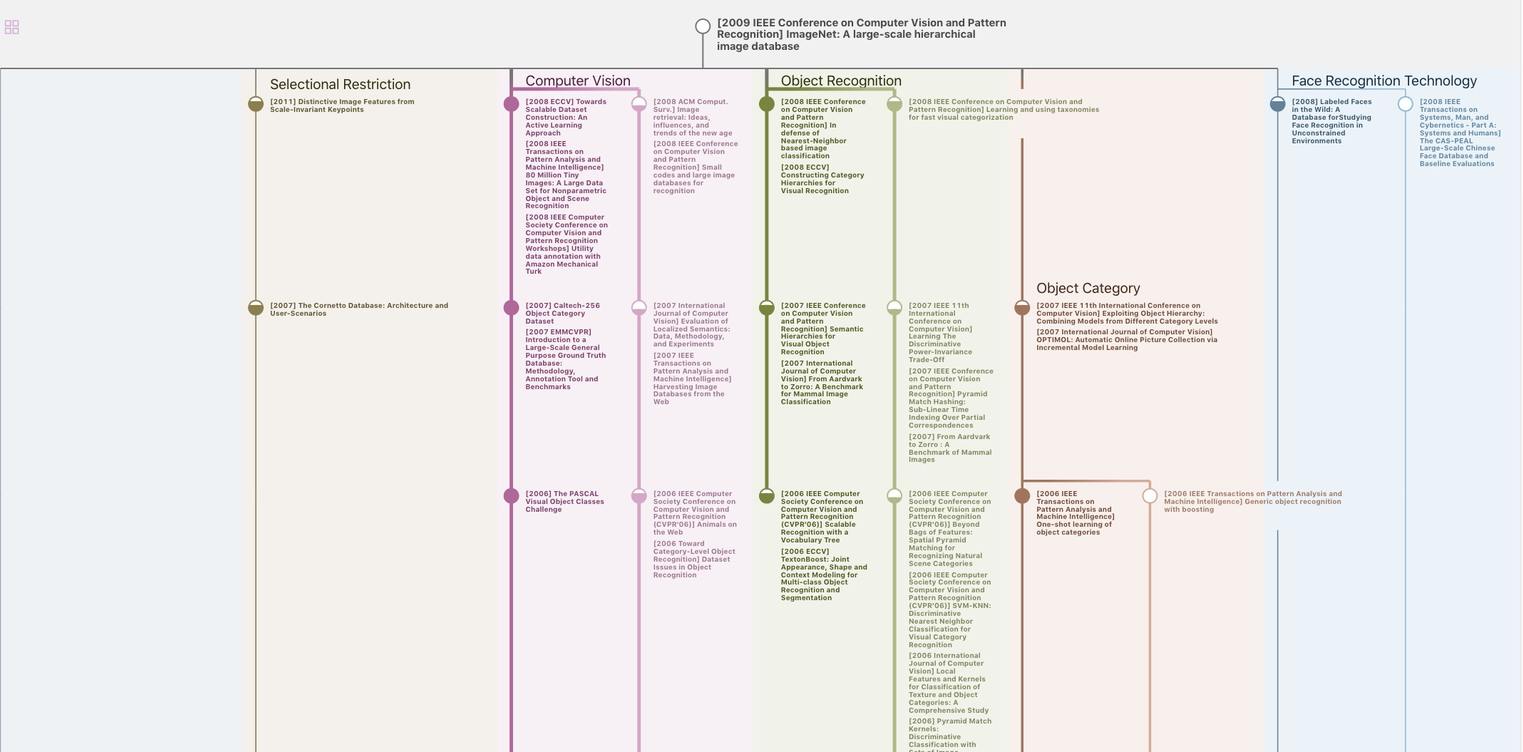
生成溯源树,研究论文发展脉络
Chat Paper
正在生成论文摘要