Improving The Minstrel Rate Adaptation Algorithm using Shallow Neural Networks in IEEE 802.11ah
2022 IEEE WIRELESS COMMUNICATIONS AND NETWORKING CONFERENCE (WCNC)(2022)
摘要
IEEE 802.11ah targets long-range IoT applications where a large number of low power wireless sensor stations are connected to an access point (AP). Rate adaptation (RA) algorithm plays a prominent role in adaptively selecting an appropriate transmission rate in order to maximize throughput in that. Minstrel RA algorithm that uses a random sampling approach has been the defacto RA in industry due to its ability to adapt rate fast in a time-varying channel and non-dependency to the air-interface design used in 802.11ah as compared to other existing RA approaches. However, the throughput performance and convergence time of the Minstrel RA algorithm are still suboptimal due to its random sampling mechanism. In this paper, we propose to improve the Minstrel RA algorithm for the 802.11ah system by designing a shallow neural networks (SNNs) module that predicts a rate input for the random sampling mechanism in Minstrel. The SNNs module is implemented in the access point and consists of several SNNs. Our simulation results in the network simulator-3 (NS-3) show that our proposed algorithm is able to improve the conventional Minstrel algorithm's convergence time and throughput by around 3.5 times and 40%.
更多查看译文
关键词
IEEE 802.11.ah, network simulator 3, shallow neural network, rate adaptation
AI 理解论文
溯源树
样例
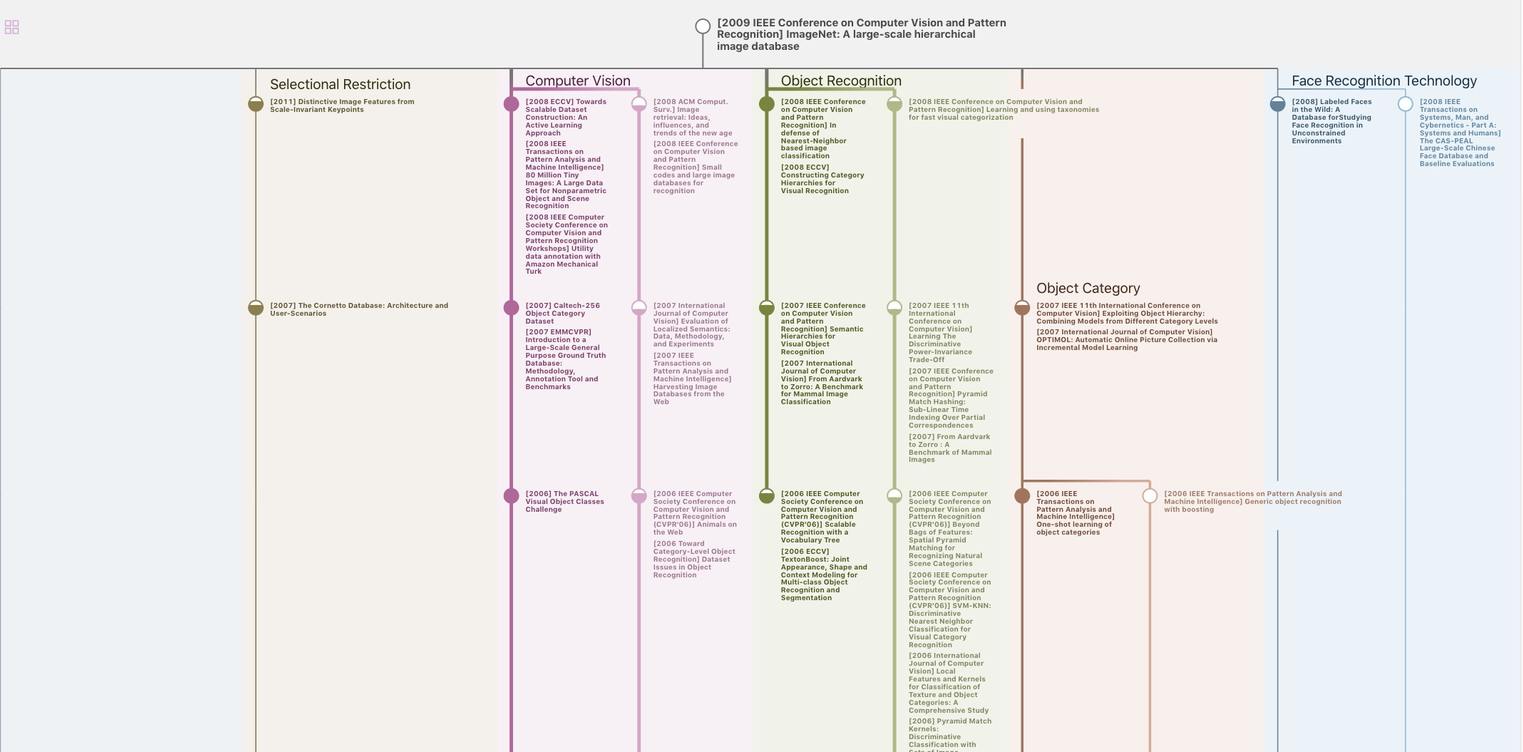
生成溯源树,研究论文发展脉络
Chat Paper
正在生成论文摘要