Towards Learning Ocean Models for Long-term Navigation in Dynamic Environments
OCEANS 2022(2022)
摘要
The use of underwater robot systems, including Autonomous Underwater Vehicles (AUVs), has been studied as an effective way of monitoring and exploring dynamic aquatic environments. Furthermore, advances in artificial intelligence techniques and computer processing led to a significant effort towards fully autonomous navigation and energy-efficient approaches. In this work, we formulate a reinforcement learning framework for long-term navigation of underwater vehicles in dynamic environments using the techniques of tile coding and eligibility traces. Simulation results used actual oceanic data from the Regional Ocean Modeling System (ROMS) data set collected in Southern California Bight (SCB) region, California, USA.
更多查看译文
关键词
reinforcement learning,long-term navigation,tile coding,eligibility traces,Regional Ocean Modeling System data,underwater robot systems,autonomous underwater vehicles,artificial intelligence,computer processing,ocean models learning,dynamic aquatic environments
AI 理解论文
溯源树
样例
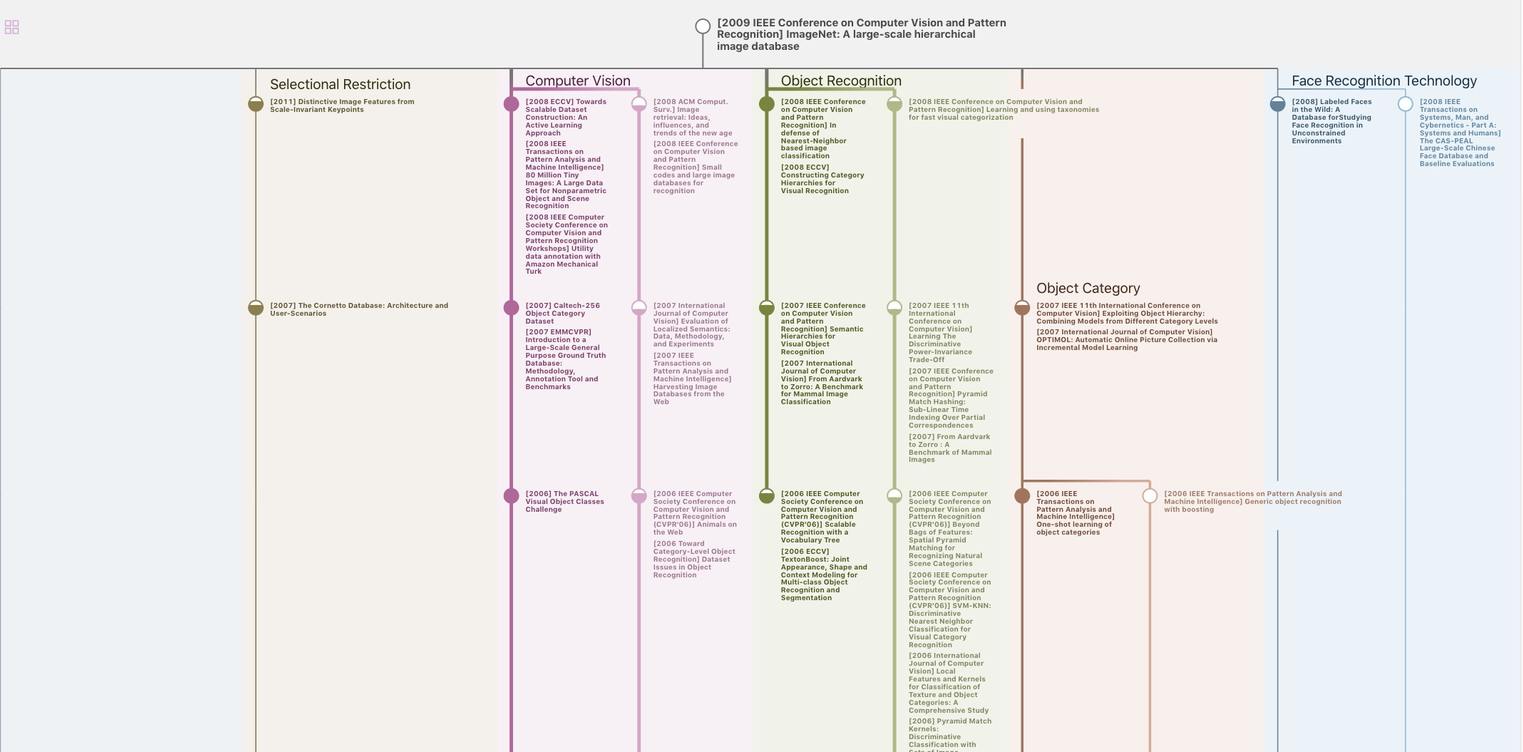
生成溯源树,研究论文发展脉络
Chat Paper
正在生成论文摘要