Mid- to Long-Term Electric Load Forecasting Based on the EMD-Isomap-Adaboost Model
SUSTAINABILITY(2022)
摘要
Accurate load forecasting is an important issue for the reliable and efficient operation of a power system. In this study, a hybrid algorithm (EMDIA) that combines empirical mode decomposition (EMD), isometric mapping (Isomap), and Adaboost to construct a prediction mode for mid- to long-term load forecasting is developed. Based on full consideration of the meteorological and economic factors affecting the power load trend, the EMD method is used to decompose the load and its influencing factors into multiple intrinsic mode functions (IMF) and residuals. Through correlation analysis, the power load is divided into fluctuation term and trend term. Then, the key influencing factors of feature sequences are extracted by Isomap to eliminate the correlations and redundancy of the original multidimensional sequences and reduce the dimension of model input. Eventually, the Adaboost prediction method is adopted to realize the prediction of the electrical load. In comparison with the RF, LSTM, GRU, BP, and single Adaboost method, the prediction obtained by this proposed model has higher accuracy in the mean absolute percentage error (MAPE), mean absolute error (MAE), root mean square error (RMSE), and determination coefficient (R-2). Compared with the single Adaboost algorithm, the EMDIA reduces MAE by 11.58, MAPE by 0.13%, and RMSE by 49.93 and increases R-2 by 0.04.
更多查看译文
关键词
power load forecasting, Isomap, Adaboost, differential empirical mode decomposition
AI 理解论文
溯源树
样例
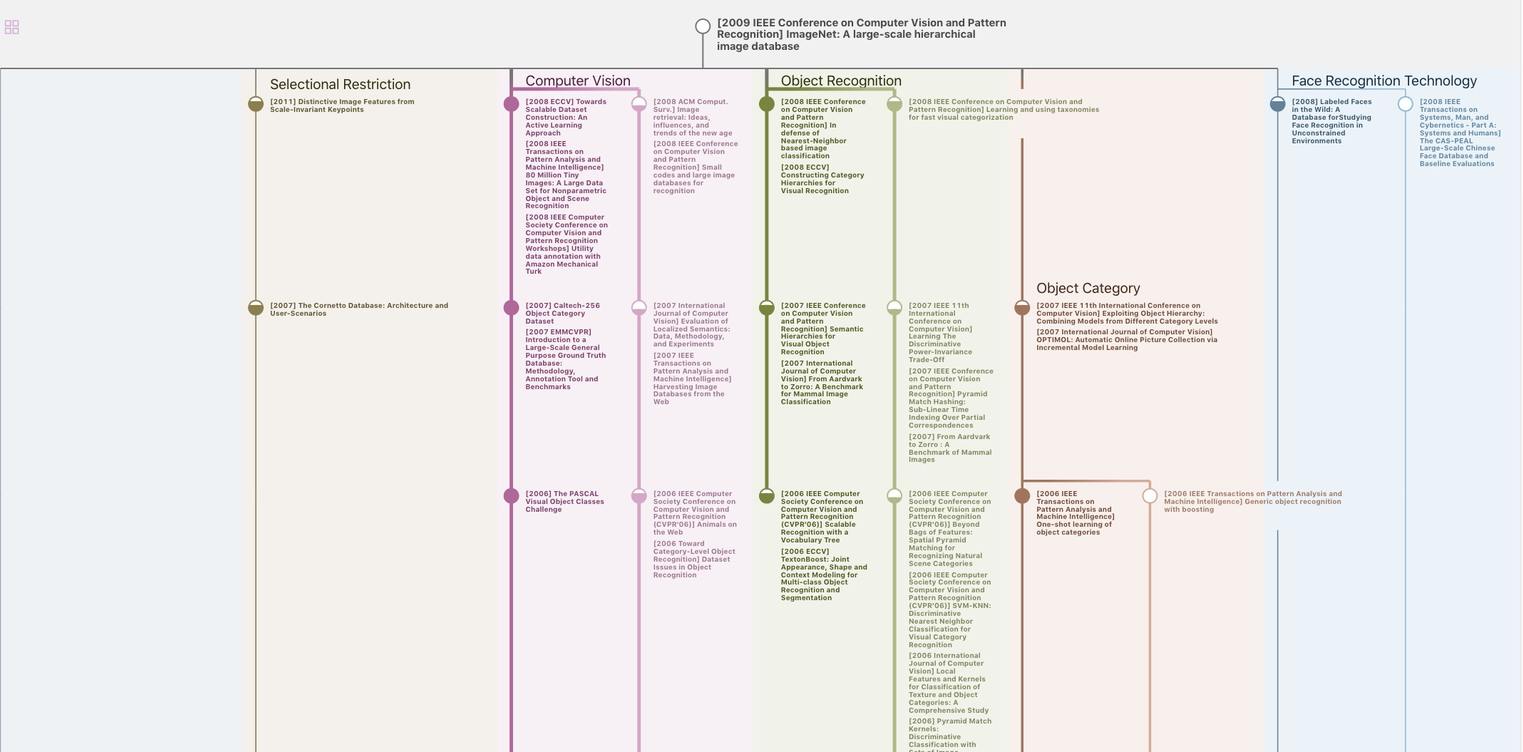
生成溯源树,研究论文发展脉络
Chat Paper
正在生成论文摘要