Industrial battery operation and utilization in the presence of electrical load uncertainty using Bayesian decision theory
Journal of Energy Storage(2022)
摘要
Behind the meter battery storage is becoming increasing popular in all sectors, though enthusiasm has recently lagged in the industrial sector. Even though there may be many factors contributing to this including lack of innovation, prohibitive costs, and undesirable rate structures, a difficulty arises in accounting for uncertainty of electrical load in industrial facilities while still attempting to utilize battery storage as much as possible all while trying to achieve fiscal profitability. This study utilizes Gaussian process regression and Bayesian decision theory to organize load data and quantify electrical load uncertainty to properly and effectively discharge industrial battery storage. The study employs a simulation model to set battery load setpoints for the span of the utility billing period according to the degree of risk aversion. This combination of economic analysis according to utility billing period and utilization of degree of risk aversion to make decisions on the uncertainty of the data has not before been applied to battery storage. The method resulted in an annual average reduction of peak demand by 3.8 % at the lowest amount of savings and lowest risk aversion. The highest risk aversion resulted in an annual average reduction of peak demand of 7.5 %. The maximum reduction of peak load in any month was 13.8 % in the month of December with a relatively high risk aversion. With a the highest amount risk aversion tested, the model reduced demand ten of the twelve months of the year.
更多查看译文
关键词
Batteries,Energy storage,Bayesian,Industrial energy,Energy management,Gaussian process regression
AI 理解论文
溯源树
样例
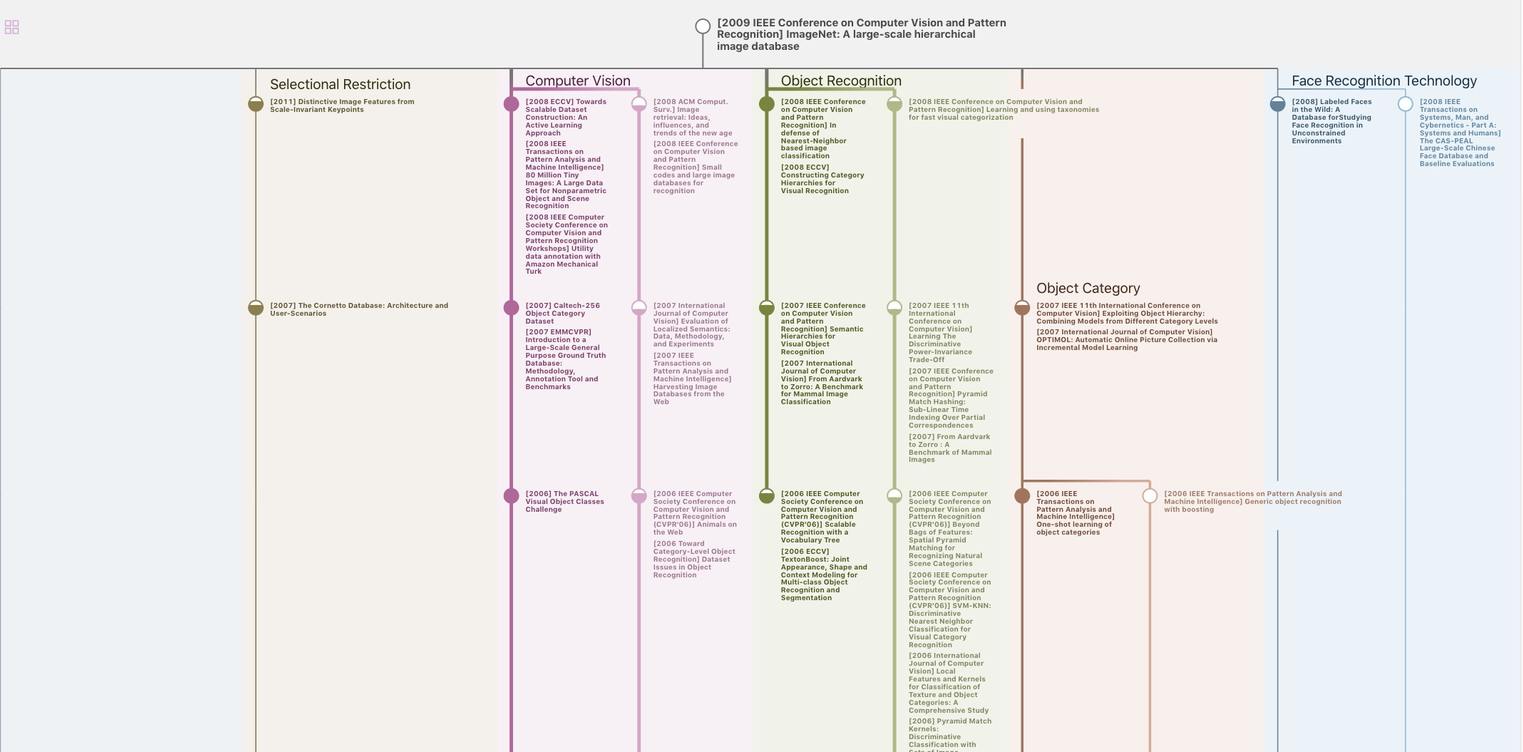
生成溯源树,研究论文发展脉络
Chat Paper
正在生成论文摘要