AB-FGSM: AdaBelief optimizer and FGSM-based approach to generate adversarial examples
Journal of Information Security and Applications(2022)
摘要
Deep neural networks (DNNs) can be misclassified by adversarial examples, which are legitimate inputs integrated with imperceptible perturbations at the testing stage. Extensive research has made progress for white-box adversarial attacks to craft adversarial examples with a high success rate. However, these crafted examples have a low success rate in misleading black-box models with defensive mechanisms. To tackle this problem, we design an AdaBelief based iterative Fast Gradient Sign Method (AB-FGSM) to generalize adversarial examples. By integrating the AdaBelief optimizer into the iterative-FGSM (I-FGSM), the generalization of adversarial examples is boosted, considering that the AdaBelief method can find the transferable adversarial point in the ε ball around the legitimate input on different optimization surfaces. We carry out white-box and black-box attacks on various adversarially trained models and ensemble models to verify the effectiveness and transferability of the adversarial examples crafted by AB-FGSM. Our experimental results indicate that the proposed AB-FGSM can efficiently and effectively craft adversarial examples in the white-box setting compared with state-of-the-art attacks. In addition, the transfer rate of adversarial examples is 4% to 21% higher than that of state-of-the-art attacks in the black-box manner.
更多查看译文
关键词
Adversarial Examples,Deep Learning,Generalization,Optimization,Security,Transferability
AI 理解论文
溯源树
样例
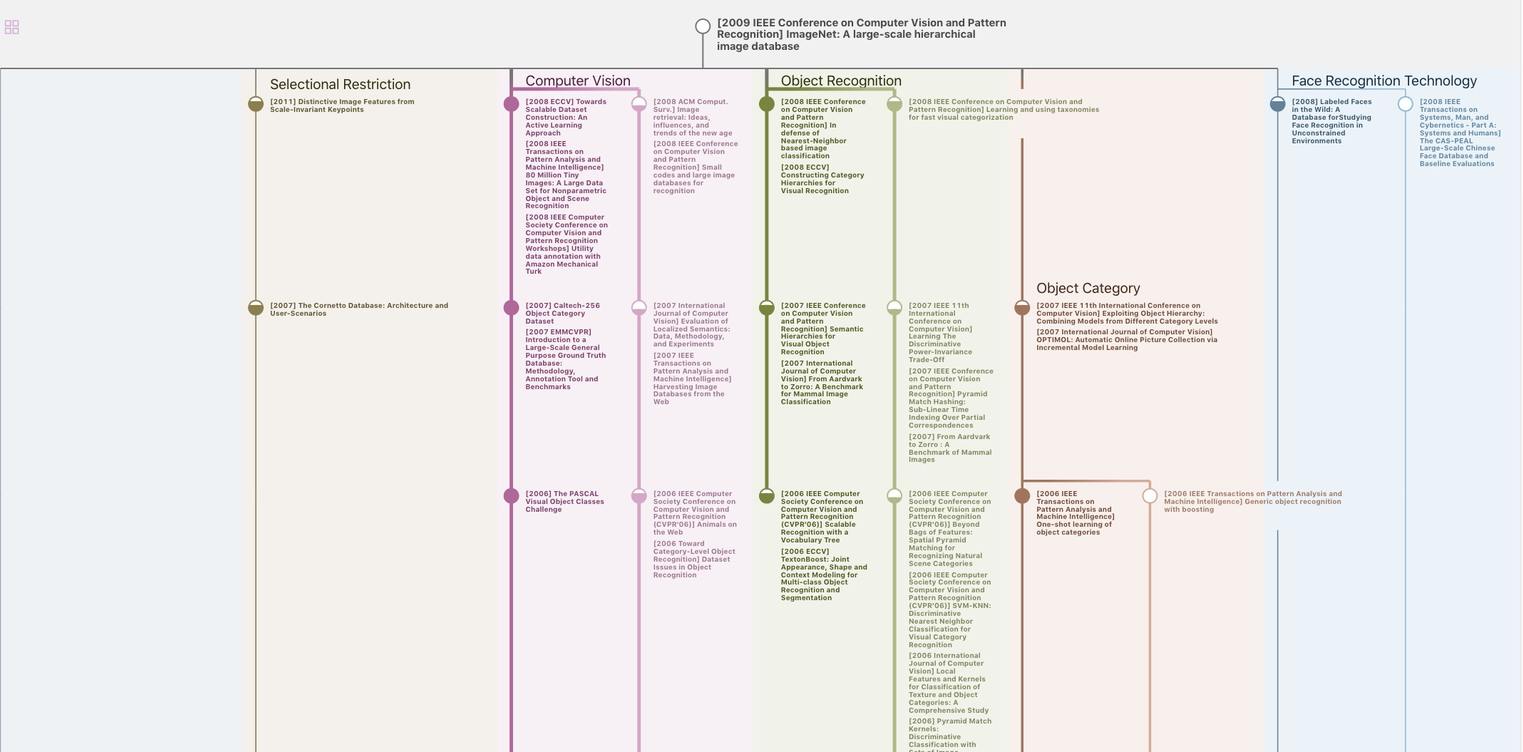
生成溯源树,研究论文发展脉络
Chat Paper
正在生成论文摘要