Two extended versions of ranking vector approach to estimation performance ranking
Expert Systems with Applications(2022)
摘要
For estimation performance ranking, the ranking vector (RV) approach using pairwise comparison information is efficient and processes advanced properties (e,g, homogeneity, invariance, monotonicity, decisiveness, and getting around intransitivity problem). However, the existing RV approach does not use quantity information of comparison and thus may be unreliable for inadequate number of error measures. To address this drawback, we develop two extended versions of the previously proposed RV approach in this paper. The first one focusing on using quantity information is based on a novel data normalization approach named pairwise data normalization. It quantifies the relative distance (goodness) of two elements with respect to a perfect point as the reference. The second version accounts for attribute difference and determines the weights of attributes accordingly. If the data of an error measure are highly different from those of others, this error measure should be weighted more in the performance ranking because it definitely reflects a different performance aspect. We compare different RV approaches, analyze their pros and cons, and discuss how to apply them for different cases. Examples are also provided to illustrate the application of these approaches to estimation performance ranking.
更多查看译文
关键词
Performance ranking,Decision making,Data normalization,Estimation,Ranking vector
AI 理解论文
溯源树
样例
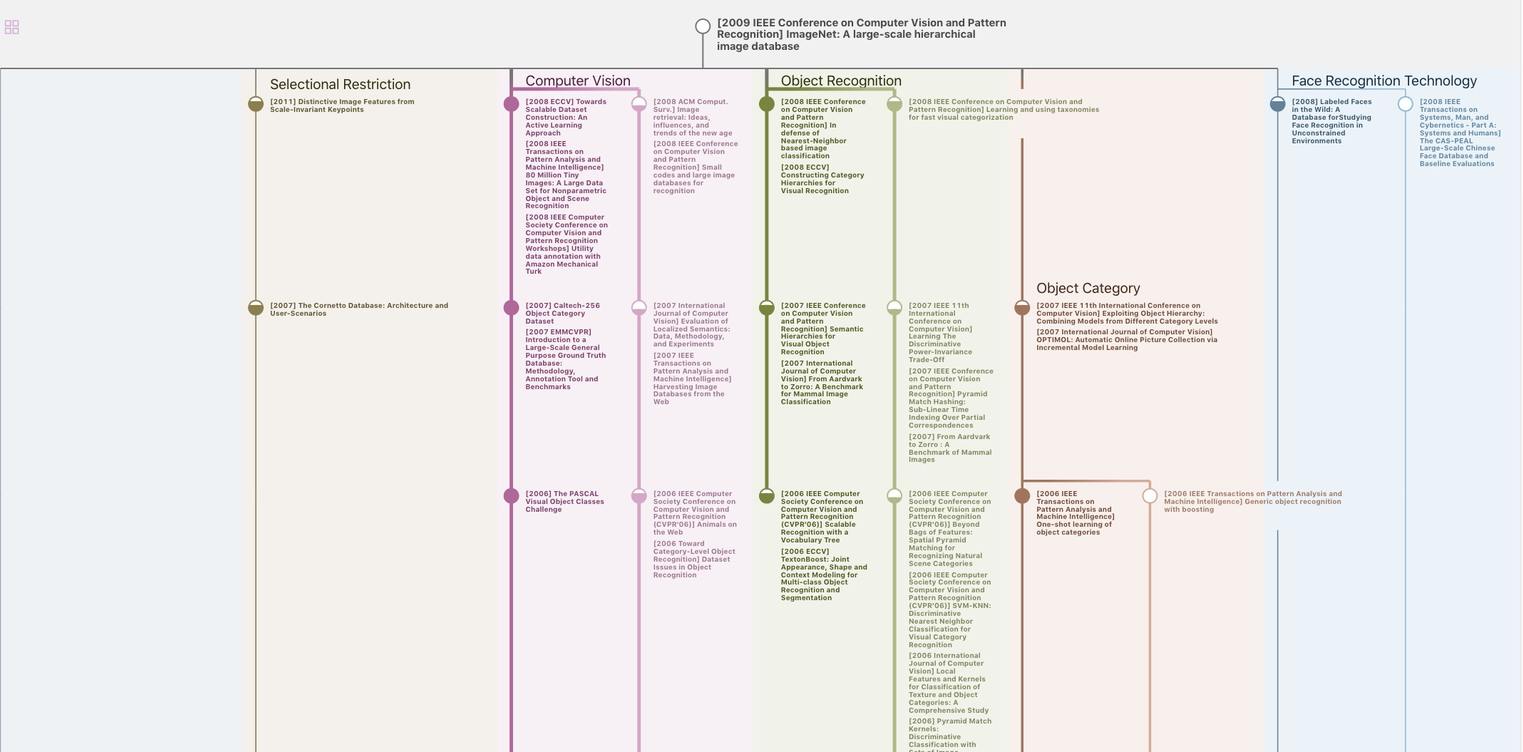
生成溯源树,研究论文发展脉络
Chat Paper
正在生成论文摘要