Automatic defect detection of texture surface with an efficient texture removal network
Advanced Engineering Informatics(2022)
摘要
Automated defect inspection of texture surface is still a challenging task in the industrial automation field due to the tremendous changes in the appearance of various surface textures. We present a simple but powerful image transformation network to remove textures and highlight defects at full resolution. The simple full convolution network consists only of 3 × 3 regular convolution and several dilated convolution blocks, which makes it compact and able to capture multi-scale features effectively. To further improve the ability of the network to suppress texture and highlight defects, a polynomial loss function combining perceptual loss, structural similarity loss and image gradient loss is proposed. In addition, a semi-automatic annotation method mainly composed of wavelet transform and relative total variation is designed to generate a data set of image pairs containing the original texture image and the desired texture removal image. We conducted experiments on a milled metal surface defect dataset and an open data set containing various textured backgrounds to evaluate the performance of our method. Compared with other convolutional neural network approaches, the results demonstrate the superiority of the proposed method. The method has been applied to the surface defect online detection system of an aluminum ingot milling production line, which effectively improves the surface inspection efficiency and product quality.
更多查看译文
关键词
Texture surface defect inspection,Texture removal convolutional network,Dilated convolution,Composite perceptual loss
AI 理解论文
溯源树
样例
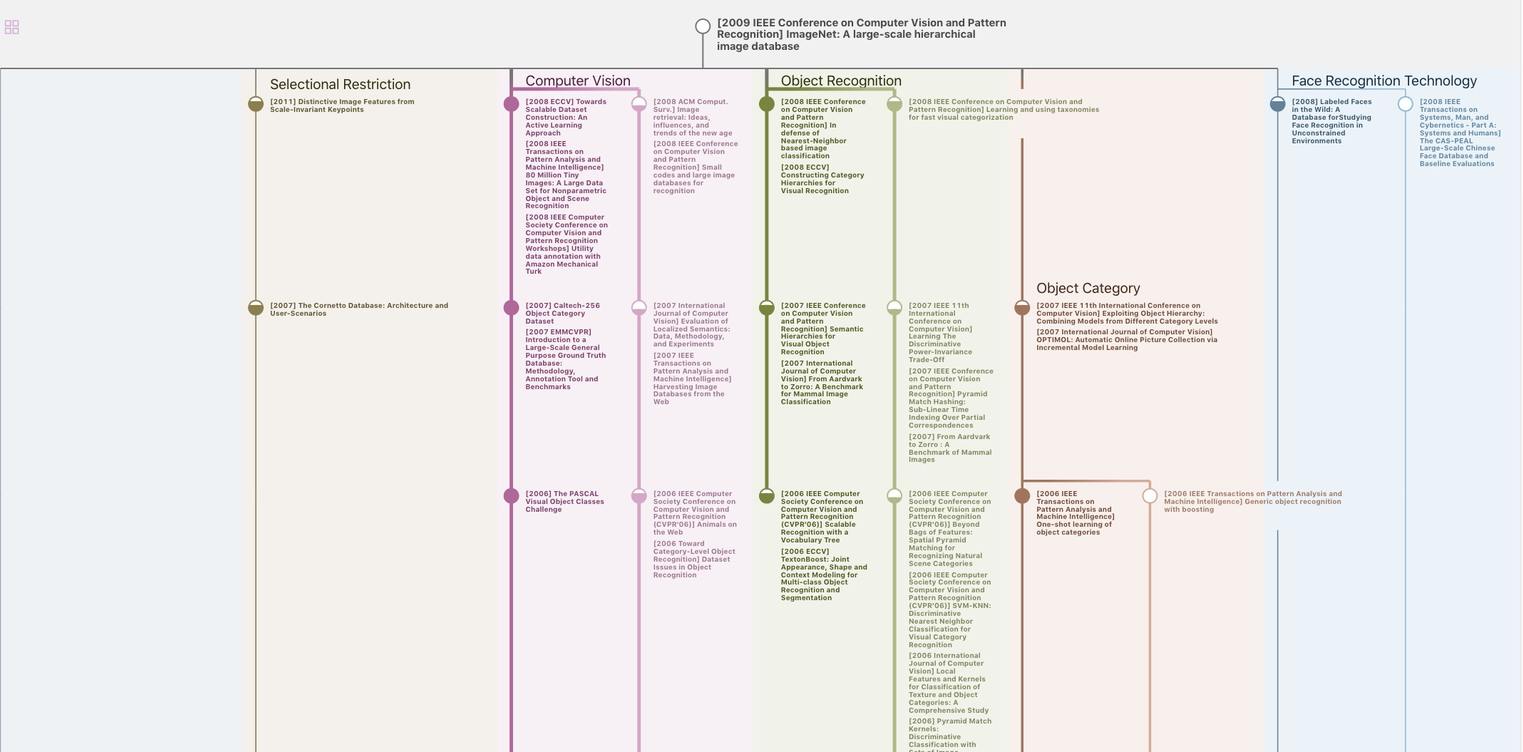
生成溯源树,研究论文发展脉络
Chat Paper
正在生成论文摘要