SMDM: Tackling zero-shot relation extraction with semantic max-divergence metric learning
Applied Intelligence(2022)
摘要
In zero-shot relation extraction, existing methods usually learn semantic features from seen relations to infer unseen relations. However, because there is no instance of unseen relation that can be used for training, it is still a challenge for the existing models to learn the semantic gap between seen relations and unseen relations, resulting in poor generalization performance of the learned semantic features. Therefore, we propose a Semantic Max-Divergence Metric (SMDM) based method to measure the distances between relations from both direct and indirect semantic differences. For that, we learn multiple binary feature reference-spaces to extract the semantic divergences of each unseen relation instance relative to each seen relation, which can be converted to a relative-affinity (RA) matrix as indirect semantic metrics. Furthermore, we combine RA with direct semantic metrics based on BERT to maximum the divergences between unseen relation instances and get clearer unseen relation boundaries. Empirical results on benchmark datasets demonstrate SMDM can the superior improvement on F1-score and external indicators of SMDM compared to the state-of-the-art methods.
更多查看译文
关键词
Relation extraction, Zero-shot, Semantic metrics, Unseen relations
AI 理解论文
溯源树
样例
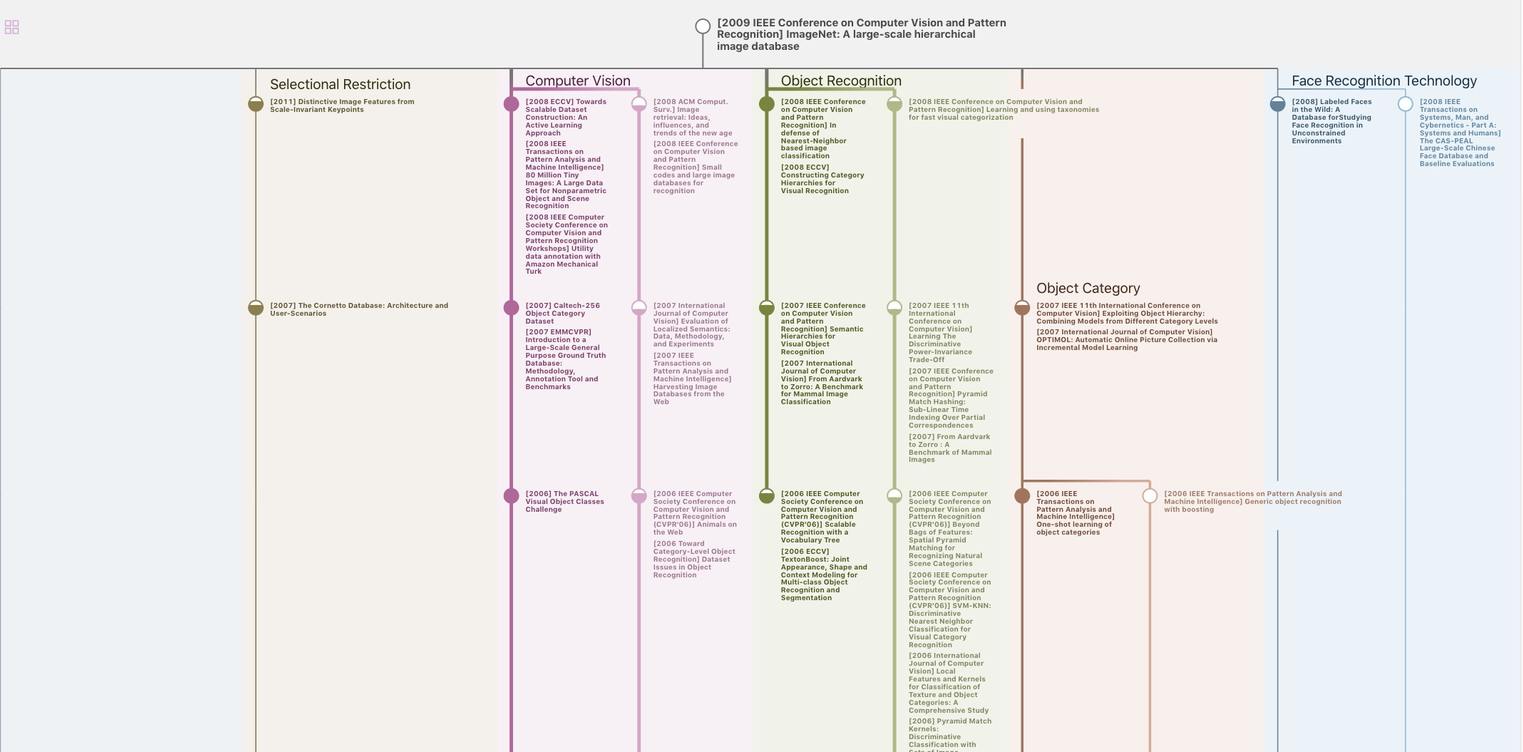
生成溯源树,研究论文发展脉络
Chat Paper
正在生成论文摘要