Latent State Space Modeling of High-Dimensional Time Series With a Canonical Correlation Objective
IEEE CONTROL SYSTEMS LETTERS(2022)
摘要
High-dimensional time series are commonly encountered in modern control systems, especially in autonomous systems. In this letter, a novel parsimonious latent state space (LaSS) model is proposed to characterize the latent dynamics, achieving general latent dynamic modeling with dimension reduction. The LaSS model is optimized by alternating estimations of the dimension reduction projection and the latent state space model. Specifically, the latent state dynamics are estimated by stochastic subspace identification methods. Furthermore, the canonical correlation analysis (CCA) objective is employed to acquire the optimal predictability for the extracted latent variables. The proposed LaSS-CCA algorithm is tested on a real industrial case for its effectiveness.
更多查看译文
关键词
Time series analysis,Predictive models,Data models,Correlation,Feature extraction,Reactive power,Heuristic algorithms,Dynamic latent variable models,reduced-dimensional dynamics,dynamic factor models,latent state space models
AI 理解论文
溯源树
样例
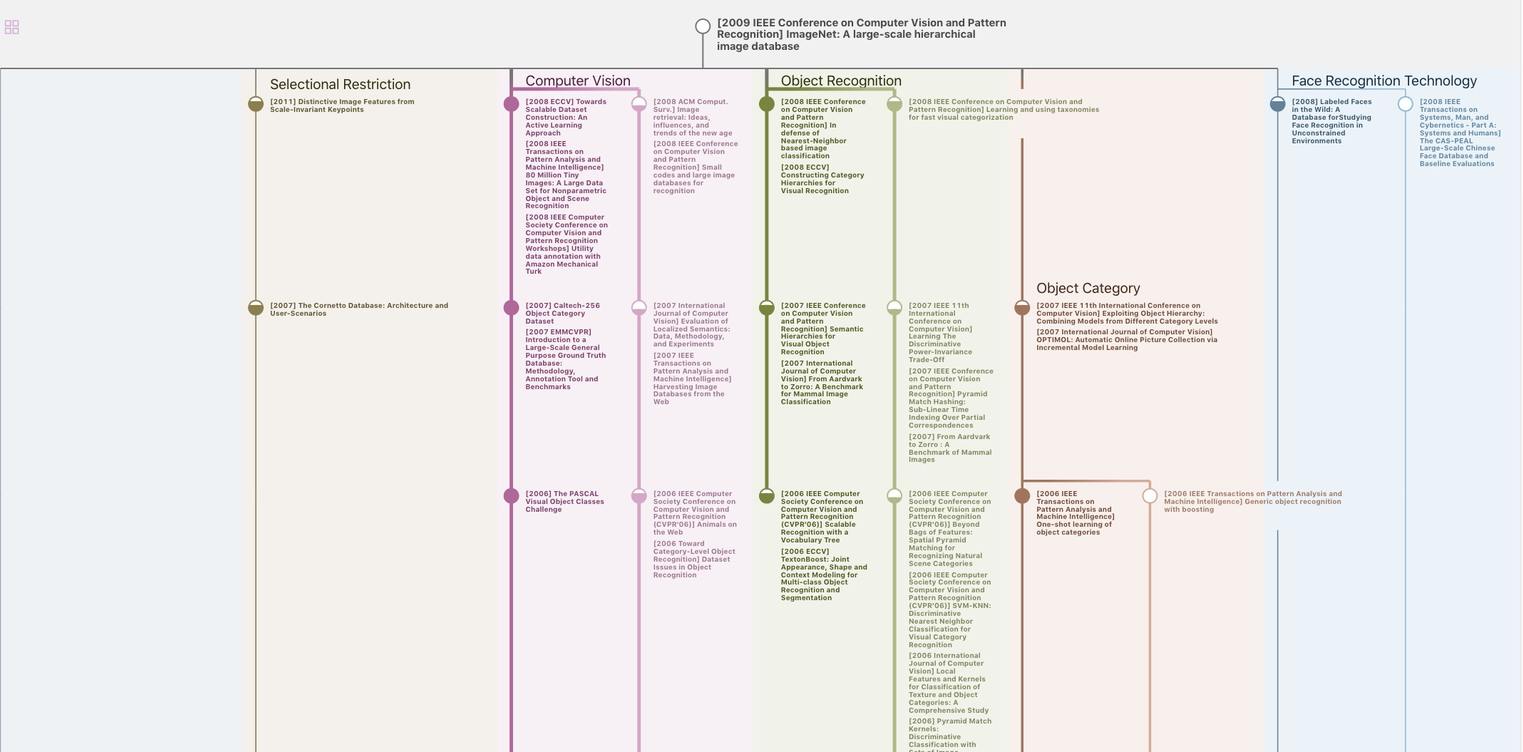
生成溯源树,研究论文发展脉络
Chat Paper
正在生成论文摘要