Effectively Identifying Compound-Protein Interaction using Graph Neural Representation.
IEEE/ACM transactions on computational biology and bioinformatics(2022)
摘要
Effectively identifying compound-protein interactions (CPIs) is crucial for new drug design, which is an important step in silico drug discovery. Current machine learning methods for CPI prediction mainly use one-demensional (1D) compound/protein strings and/or the specific descriptors. However, they often ignore the fact that molecules are essentially modeled by the molecular graph. We observe that in real-world scenarios, the topological structure information of the molecular graph usually provides an overview of how the atoms are connected, and the local chemical context reveals the functionality of the protein sequence in CPI. These two types of information are complementary to each other and they are both significant for modeling compound-protein pairs. Motivated by this, we propose an end-to-end deep learning framework named GraphCPI, which captures the structural information of compounds and leverages the chemical context of protein sequences for solving the CPI prediction task. Our framework can integrate any popular graph neural networks for learning compounds, and it combines with a convolutional neural network for embedding sequences. To compare our method with classic and state-of-the-art deep learning methods, we conduct extensive experiments based on several widely-used CPI datasets. The experimental results show the feasibility and competitiveness of our proposed method.
更多查看译文
关键词
Neural Networks, Computer,Machine Learning,Drug Discovery,Proteins,Amino Acid Sequence
AI 理解论文
溯源树
样例
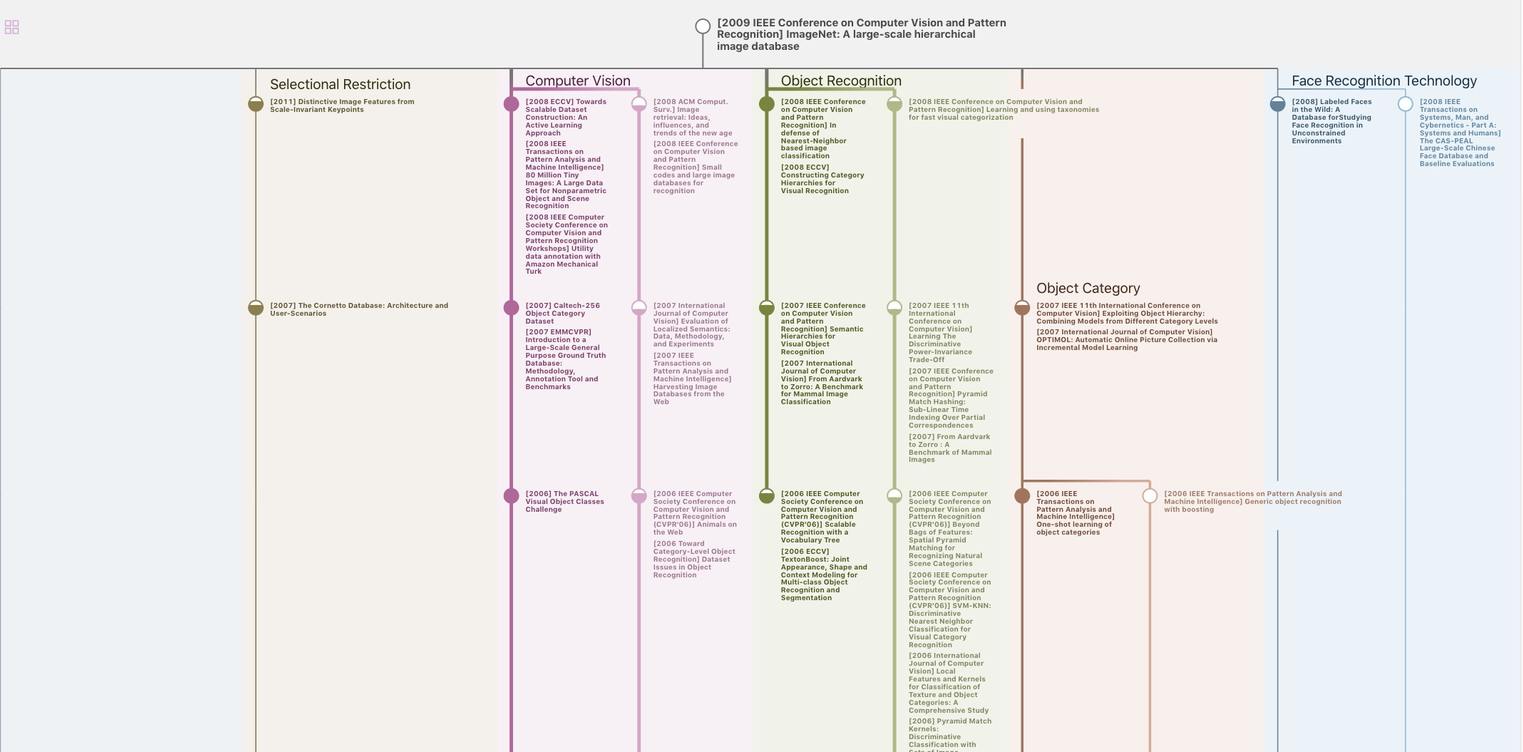
生成溯源树,研究论文发展脉络
Chat Paper
正在生成论文摘要