ZiXia: A Reinforcement Learning Approach via Adjusted Ranking Reward for Internet Congestion Control
ICC 2022 - IEEE International Conference on Communications(2022)
摘要
Congestion control (CC) algorithms based on deep reinforcement learning (DRL) have shown their great potential to adapt themselves to a variety of network conditions. However, as the real-world network conditions are diverse and the optimization goals for CC are made up of some contradicted metrics, it is hard to balance these contradicted absolute valves, which makes it difficult to faithfully reflect the algorithm performance if only considering transient status as the reward. In this work, we propose a novel DRL-based CC approach ZiXia, which considers the adjusted ranking reward, the long-term relative performance reward adjusted by the transient reward. In detail, we design a virtual algorithm arena including the DRL-agents and other classic algorithms as competitors in the same environment. After these algorithms end, we rank their delay and throughput respectively and combine the two relative rankings as the ranking reward using the special preference. The ranking reward gives us a more flexible and interpretable long-term evaluation method compared with the absolute value of the transient status, which gives us a more intuitive perspective to support multi-objection. To get more fine-grained action rewards, we adjust the ranking reward using a linear combination of transient status. Through various experiments, in simulated environments, ZiXia achieves the highest throughput and reduces 87% delay compared with BBR, and in global real-world environments, ZiXia improves 13% throughput and reduces 3% delay than BBR.
更多查看译文
关键词
Congestion Control,Deep Reinforcement Learning
AI 理解论文
溯源树
样例
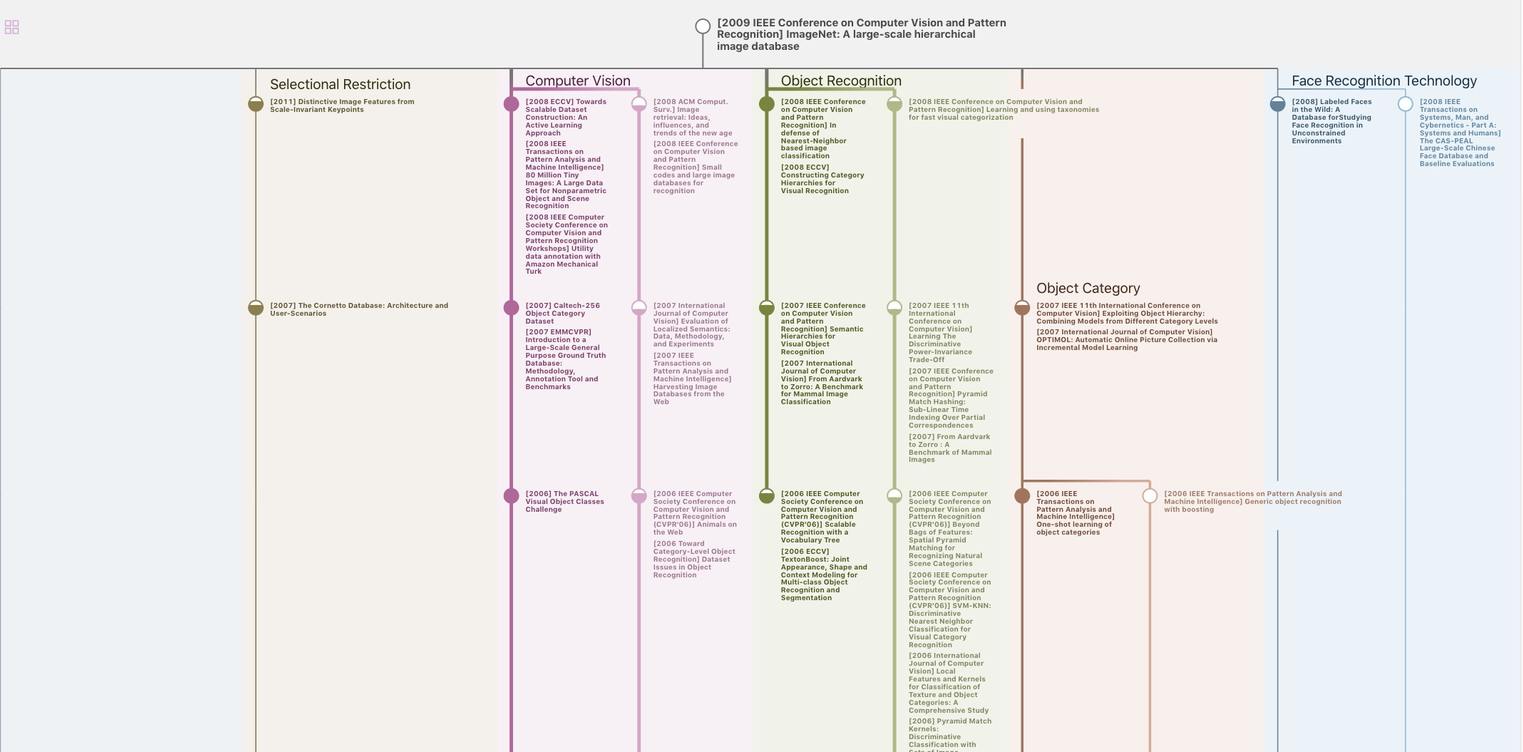
生成溯源树,研究论文发展脉络
Chat Paper
正在生成论文摘要