Online Clustering: Algorithms, Evaluation, Metrics, Applications and Benchmarking
Proceedings of the 28th ACM SIGKDD Conference on Knowledge Discovery and Data Mining(2022)
摘要
Online clustering algorithms play a critical role in data science, especially with the advantages regarding time, memory usage and complexity, while maintaining a high performance compared to traditional clustering methods. This tutorial serves, first, as a survey on online machine learning and, in particular, data stream clustering methods. During this tutorial, state-of-the-art algorithms and the associated core research threads will be presented by identifying different categories based on distance, density grids and hidden statistical models. Clustering validity indices, an important part of the clustering process which are usually neglected or replaced with classification metrics, resulting in misleading interpretation of final results, will also be deeply investigated. Then, this introduction will be put into the context with River, a go-to Python library merged between Creme and scikit-multiflow. It is also the first open-source project to include an online clustering module that can facilitate reproducibility and allow direct further improvements. From this, we propose methods of clustering configuration, applications and settings for benchmarking, using real-world problems and datasets.
更多查看译文
关键词
online
AI 理解论文
溯源树
样例
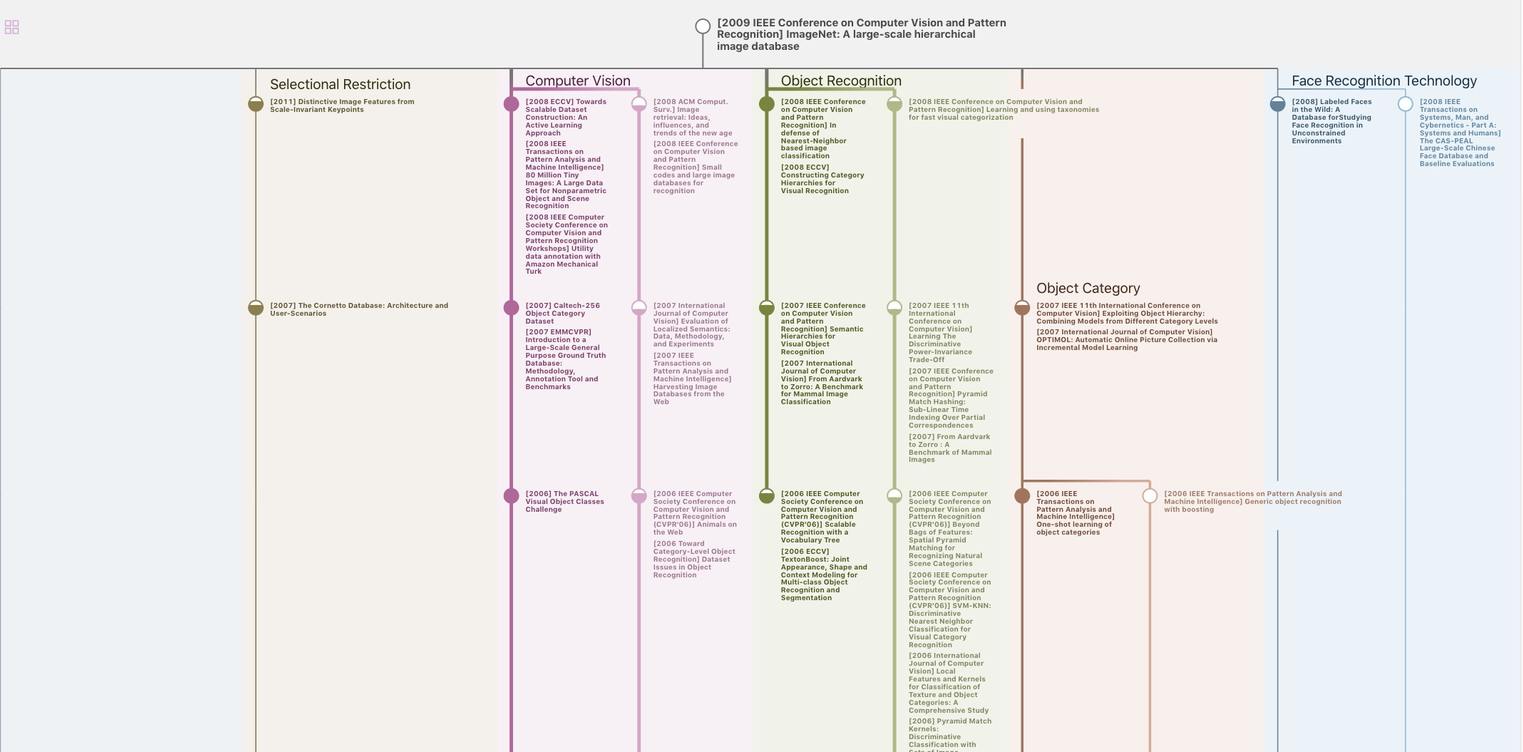
生成溯源树,研究论文发展脉络
Chat Paper
正在生成论文摘要