Uncertainty-driven ensembles of multi-scale deep architectures for image classification
Information Fusion(2023)
摘要
The use of automatic systems for medical image classification has revolutionized the diagnosis of a high number of diseases. These alternatives, which are usually based on artificial intelligence (AI), provide a helpful tool for clinicians, eliminating the inter and intra-observer variability that the diagnostic process entails. Convolutional Neural Network (CNNs) have proved to be an excellent option for this purpose, demonstrating a large performance in a wide range of contexts. However, it is also extremely important to quantify the reliability of the model’s predictions in order to guarantee the confidence in the classification. In this work, we propose a multi-level ensemble classification system based on a Bayesian Deep Learning approach in order to maximize performance while providing the uncertainty of each classification decision. This tool combines the information extracted from different architectures by weighting their results according to the uncertainty of their predictions. Performance is evaluated in a wide range of real scenarios: in the first one, the aim is to differentiate between different pulmonary pathologies: controls vs bacterial pneumonia vs viral pneumonia. A two-level decision tree is employed to divide the 3-class classification into two binary classifications, yielding an accuracy of 98.19%. In the second context, performance is assessed for the diagnosis of Parkinson’s disease, leading to an accuracy of 95.31%. The reduced preprocessing needed for obtaining this high performance, in addition to the information provided about the reliability of the predictions evidence the applicability of the system to be used as an aid for clinicians.
更多查看译文
关键词
Bayesian Deep Learning,Uncertainty,Ensemble classification,Pneumonia,Parkinson’s
AI 理解论文
溯源树
样例
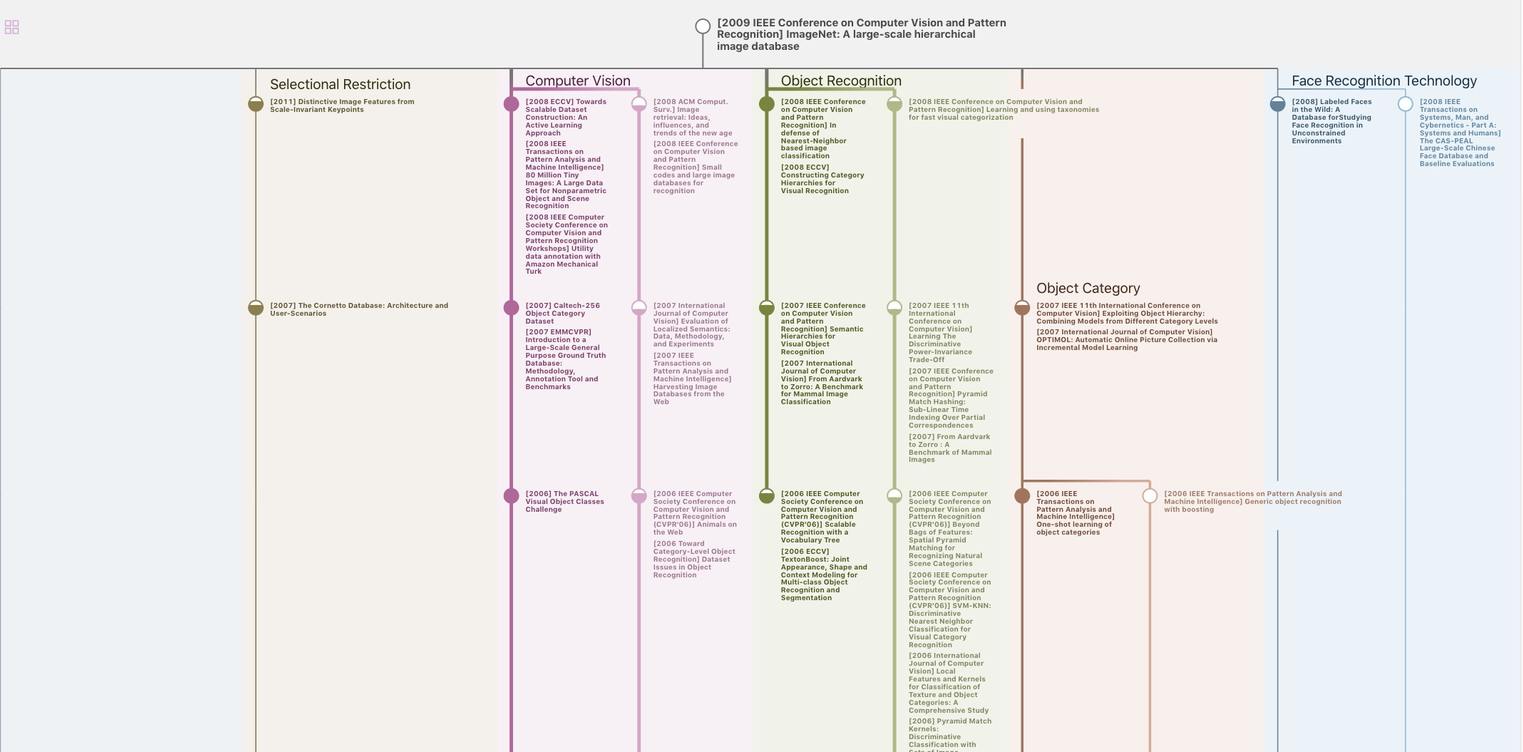
生成溯源树,研究论文发展脉络
Chat Paper
正在生成论文摘要