Phishing website detection: How effective are deep learning-based models and hyperparameter optimization
SECURITY AND PRIVACY(2022)
摘要
Phishing websites are fraudulent websites that appear legitimate and trick unsuspecting users into interacting with them, stealing their valuable information. Because phishing attacks are a leading cause of data breach, different anti-phishing solutions have been explored for cybersecurity management including machine learning-based technical approaches. However, there is a gap in understanding how robust deep learning-based models together with hyperparameter optimization are for phishing website detection. In this vein, this study pursues the tasks of developing parsimonious deep learning models and hyperparameter optimization to achieve high accuracy and reproducible results for phishing website detection. This paper demonstrates a systematic process of building detection models based on three deep learning algorithm architectures (Long Short-Term Memory-based detection models, Fully Connected Deep Neural Network-based detection models, and convolutional neural network-based detection models) that are built and evaluated using four publicly available phishing website datasets, achieving the best accuracy of 97.37%. We also compared two different optimization algorithms for hyperparameter optimization: Grid Search and Genetic Algorithm, which contributed to 0.1%-1% increase in accuracy.
更多查看译文
关键词
convolutional neural network, deep learning, fully connected deep neural network, genetic algorithms, grid search algorithms, hyperparameter optimization, long short-term memory, phishing website detection, URL
AI 理解论文
溯源树
样例
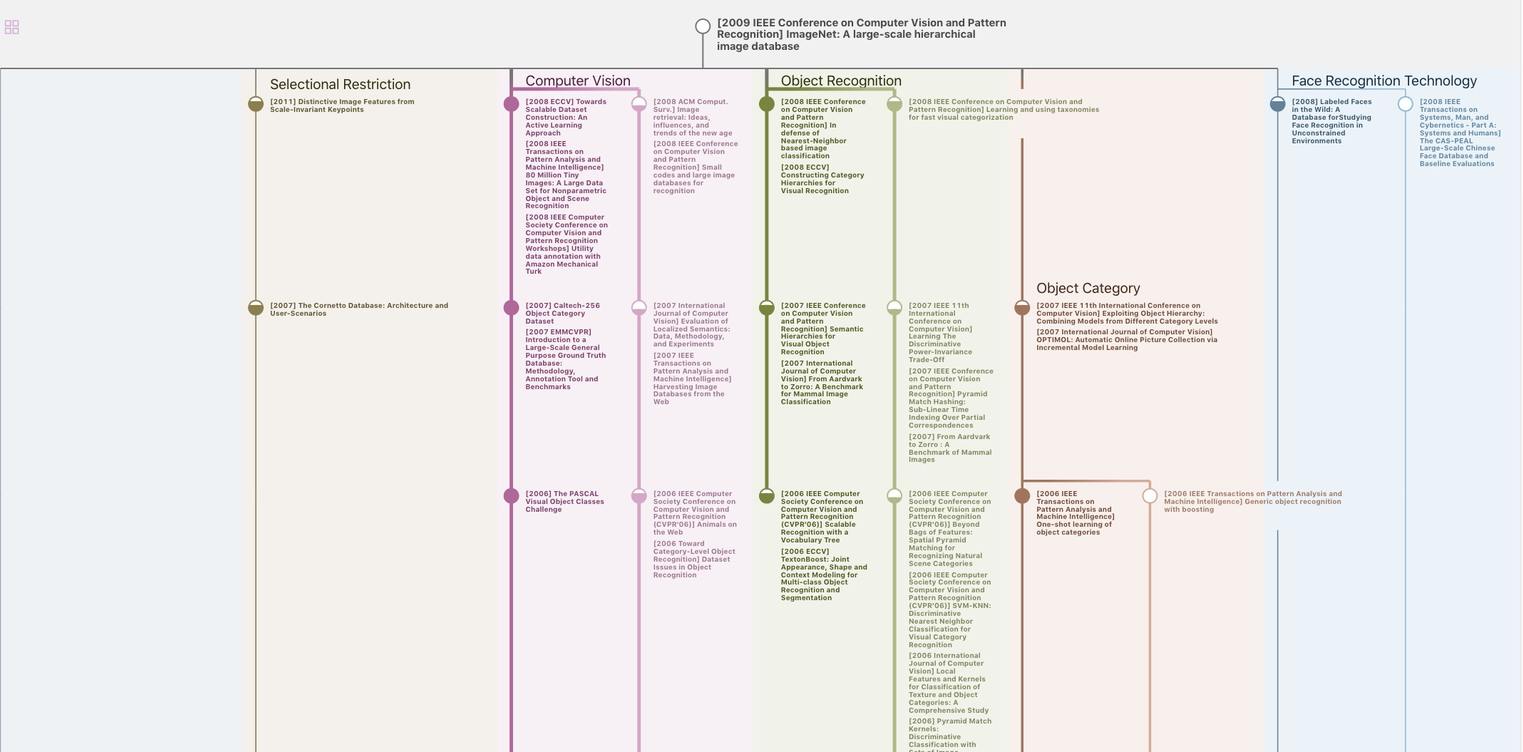
生成溯源树,研究论文发展脉络
Chat Paper
正在生成论文摘要