A Hybrid Deep Learning Model for Water Supply Time Series Data Prediction
2022 7th International Conference on Computer and Communication Systems (ICCCS)(2022)
摘要
With the development of intelligent water services, accurate prediction of water supply data plays a vital role in water supply safety and security. At the same time, it is important to ensure ecological water, water for people's livelihood and industrial water. Therefore, a hybrid multi-step prediction model called VMD-ConvGRUAE is proposed. By combining variational modal decomposition (VMD) and deep autoencoder model based on convolutional long short-term memory (ConvLSTM) and gated recurrent unit (GRU) for water supply prediction. In the proposed VMD-ConvGRUAE model, VMD is used to decompose the original water supply data into modal components with different trends and then combine the deep encoder-decoder network structure to predict the component data, and add the predicted results of each component to obtain the predicted value. Experiments on real water supply datasets, using the MAE evaluation metrics as an example, compared with several other baseline methods, the VMD-ConvGRUAE model has a 4.34% to 65.27% improvement in prediction accuracy, which verifies the validity of the model.
更多查看译文
关键词
smart water,water supply prediction,variational modal decomposition,encoder-decoder,time series
AI 理解论文
溯源树
样例
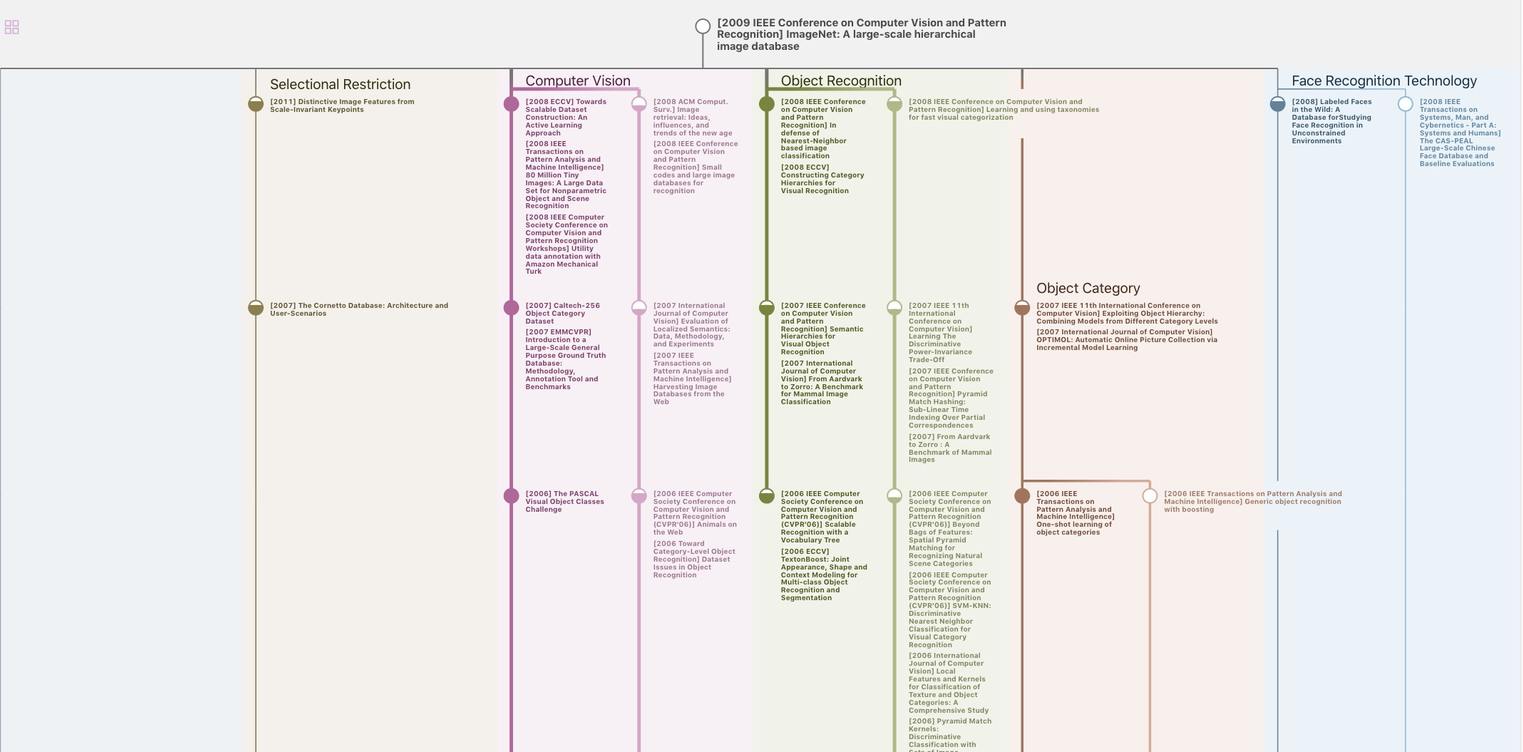
生成溯源树,研究论文发展脉络
Chat Paper
正在生成论文摘要