Deep Learning Velocity Model Building Using an Ensemble Regression Approach
Second International Meeting for Applied Geoscience & Energy(2022)
AI 理解论文
溯源树
样例
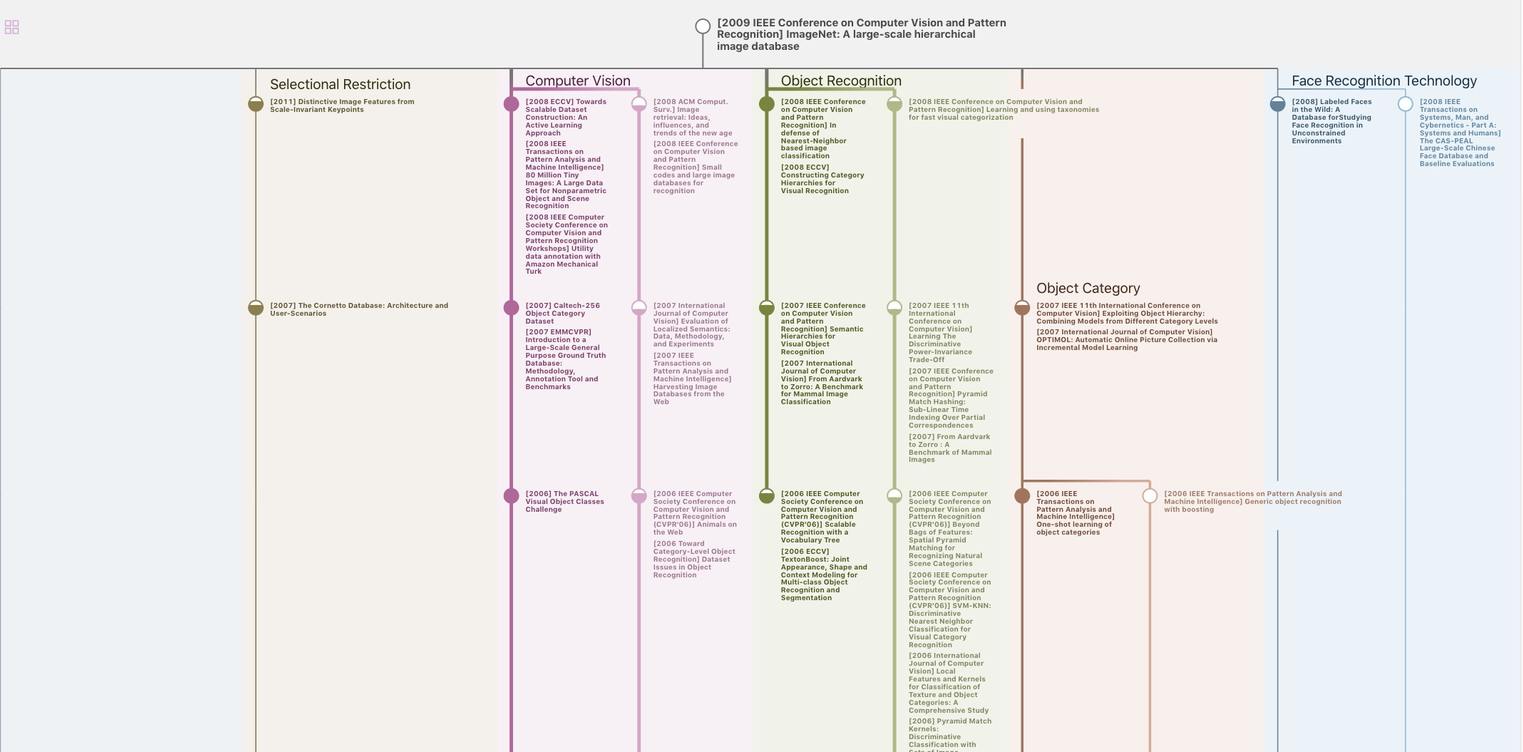
生成溯源树,研究论文发展脉络
Chat Paper
正在生成论文摘要
Second International Meeting for Applied Geoscience & Energy(2022)