Bearing Fault Diagnosis Using Lightweight and Robust One-Dimensional Convolution Neural Network in the Frequency Domain
SENSORS(2022)
摘要
The massive environmental noise interference and insufficient effective sample degradation data of the intelligent fault diagnosis performance methods pose an extremely concerning issue. Realising the challenge of developing a facile and straightforward model that resolves these problems, this study proposed the One-Dimensional Convolutional Neural Network (1D-CNN) based on frequency-domain signal processing. The Fast Fourier Transform (FFT) analysis is initially utilised to transform the signals from the time domain to the frequency domain; the data was represented using a phasor notation, which separates magnitude and phase and then fed to the 1D-CNN. Subsequently, the model is trained with White Gaussian Noise (WGN) to improve its robustness and resilience to noise. Based on the findings, the proposed model successfully achieved 100% classification accuracy from clean signals and simultaneously achieved considerable robustness to noise and exceptional domain adaptation ability. The diagnosis accuracy retained up to 97.37%, which was higher than the accuracy of the CNN without training under noisy conditions at only 43.75%. Furthermore, the model achieved an accuracy of up to 98.1% under different working conditions, which was superior to other reported models. In addition, the proposed model outperformed the state-of-art methods as the Signal-to-Noise Ratio (SNR) was lowered to -10 dB achieving 97.37% accuracy. In short, the proposed 1D-CNN model is a promising effective rolling bearing fault diagnosis.
更多查看译文
关键词
deep learning, one-dimensional convolutional neural network, signal-to-noise ratio, fault diagnosis, fast Fourier transform, bearing
AI 理解论文
溯源树
样例
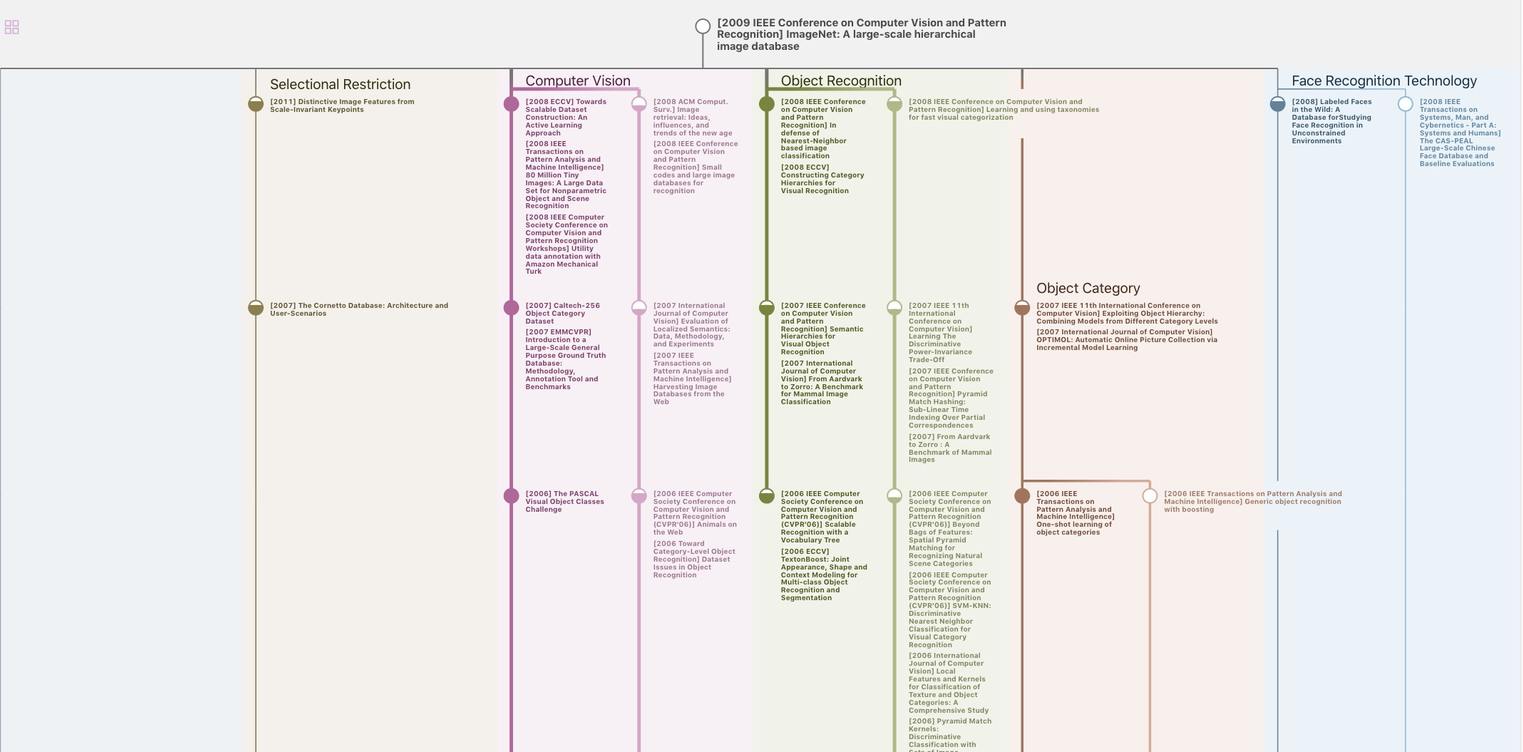
生成溯源树,研究论文发展脉络
Chat Paper
正在生成论文摘要