Machine learning inversions incorporating geologic information through variational autoencoder and physics-based neural network
Second International Meeting for Applied Geoscience & Energy(2022)
摘要
PreviousNext No AccessSecond International Meeting for Applied Geoscience & EnergyMachine learning inversions incorporating geologic information through variational autoencoder and physics-based neural networkAuthors: Yaoguo LiTaqi AlyousufAndy McAlileyYaoguo LiColorado School of MinesSearch for more papers by this author, Taqi AlyousufColorado School of MinesSearch for more papers by this author, and Andy McAlileyColorado School of MinesSearch for more papers by this authorhttps://doi.org/10.1190/image2022-3746882.1 SectionsAboutPDF/ePub ToolsAdd to favoritesDownload CitationsTrack CitationsPermissions ShareFacebookTwitterLinked InRedditEmail AbstractGeophysical inversions require the inclusion of prior geological information. Many types of prior information are implicit or conceptual in nature. For instance, the information about the features in the subsurface geology is embedded in the multiple geophysical data sets, but not directly accessible; or we may know the types of structural elements present in the subsurface but not their specifics. A long-standing challenge is in how to integrate this type of prior information. The ability of machine learning (ML) in capturing such conceptual and implicit information provides a new avenue to address this challenge. In this presentation, we discuss two approaches which we have developed. The first is a physics-based neural network that captures the implicit geological information embedded in the multiple data sets to be inverted. The second is a conditional variational autoencoder that captures the prior geological information embedded in the training models with a set of known features and associated geophysical data. Both approaches highlight the unique advantage of ML in capturing general geology information.Keywords: machine learning, inversion, geological informationPermalink: https://doi.org/10.1190/image2022-3746882.1FiguresReferencesRelatedDetails Second International Meeting for Applied Geoscience & EnergyISSN (print):1052-3812 ISSN (online):1949-4645Copyright: 2022 Pages: 3694 publication data© 2022 Published in electronic format with permission by the Society of Exploration Geophysicists and the American Association of Petroleum GeologistsPublisher:Society of Exploration Geophysicists HistoryPublished Online: 15 Aug 2022 CITATION INFORMATION Yaoguo Li, Taqi Alyousuf, and Andy McAliley, (2022), "Machine learning inversions incorporating geologic information through variational autoencoder and physics-based neural network," SEG Technical Program Expanded Abstracts : 2977-2980. https://doi.org/10.1190/image2022-3746882.1 Plain-Language Summary Keywordsmachine learninginversiongeological informationPDF DownloadLoading ...
更多查看译文
关键词
variational autoencoder,geologic information,inversions,neural network,machine learning,physics-based
AI 理解论文
溯源树
样例
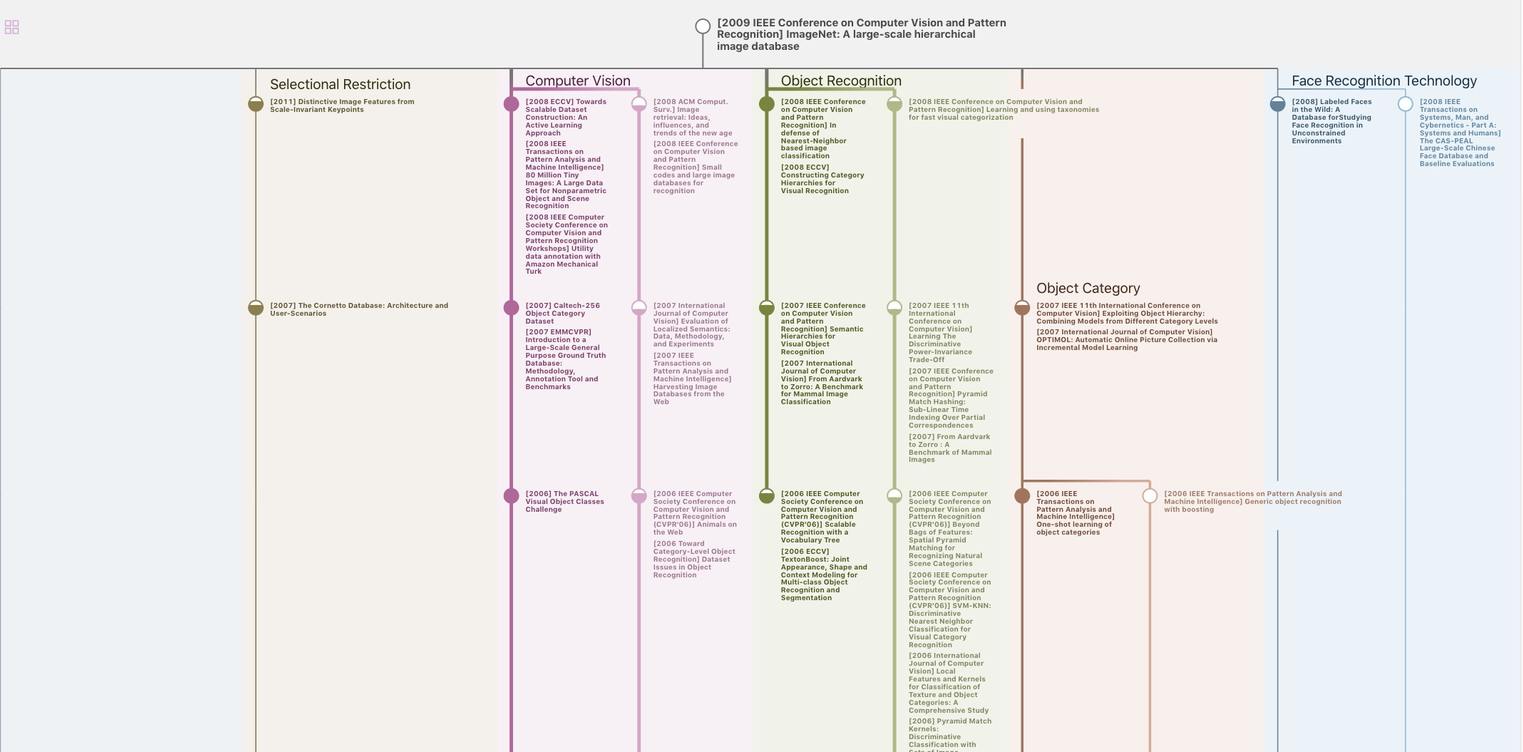
生成溯源树,研究论文发展脉络
Chat Paper
正在生成论文摘要