Two-level Filtering method with Extended Lasso and Information Gain in Microarray DataAnalysis
2022 International Conference on Inventive Computation Technologies (ICICT)(2022)
摘要
Most growing real-time applications operate with high-dimensional data and require appropriate feature selection and prediction analysis technique. The regularization approach is one of the most widely used strategies for genomic data processing. The selection of features in high-dimensional data with substantially linked variables is a critical challenge. As a result, in this work, a unique approach is proposed for feature selection, wherein TLFM (Two-Level Filtering for Microarray Data), is identified for achieving an optimal gene data. Using the Information Gain (IG), each gene was prioritized from the early stages based on its value for classification. At the first level, a subset of candidate genes is created. Later, the redundant genes are filtered and the retained information genes from the subset (i.e., candidate genes) obtained from the previous step is done by using the Extended Lasso (EL) method. The real-time datasets have tested the proposed method against the standard methods. The results of the proposed study proven that the proposed method has produced better classification results with fewer genes.
更多查看译文
关键词
extended lasso,two-level
AI 理解论文
溯源树
样例
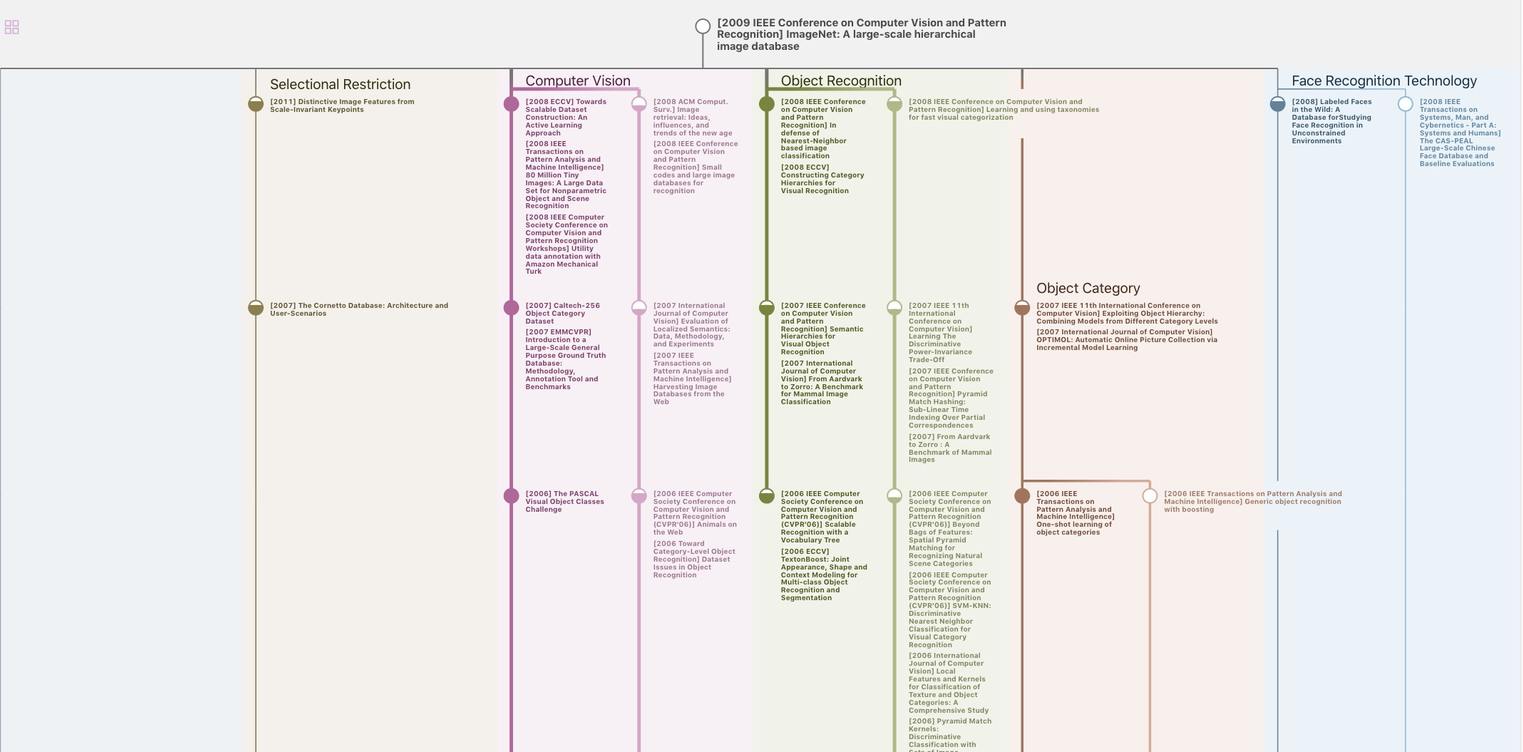
生成溯源树,研究论文发展脉络
Chat Paper
正在生成论文摘要