Matching Multiple Perspectives for Efficient Representation Learning
arxiv(2022)
摘要
Representation learning approaches typically rely on images of objects captured from a single perspective that are transformed using affine transformations. Additionally, self-supervised learning, a successful paradigm of representation learning, relies on instance discrimination and self-augmentations which cannot always bridge the gap between observations of the same object viewed from a different perspective. Viewing an object from multiple perspectives aids holistic understanding of an object which is particularly important in situations where data annotations are limited. In this paper, we present an approach that combines self-supervised learning with a multi-perspective matching technique and demonstrate its effectiveness on learning higher quality representations on data captured by a robotic vacuum with an embedded camera. We show that the availability of multiple views of the same object combined with a variety of self-supervised pretraining algorithms can lead to improved object classification performance without extra labels.
更多查看译文
关键词
multiple perspectives,representation,learning
AI 理解论文
溯源树
样例
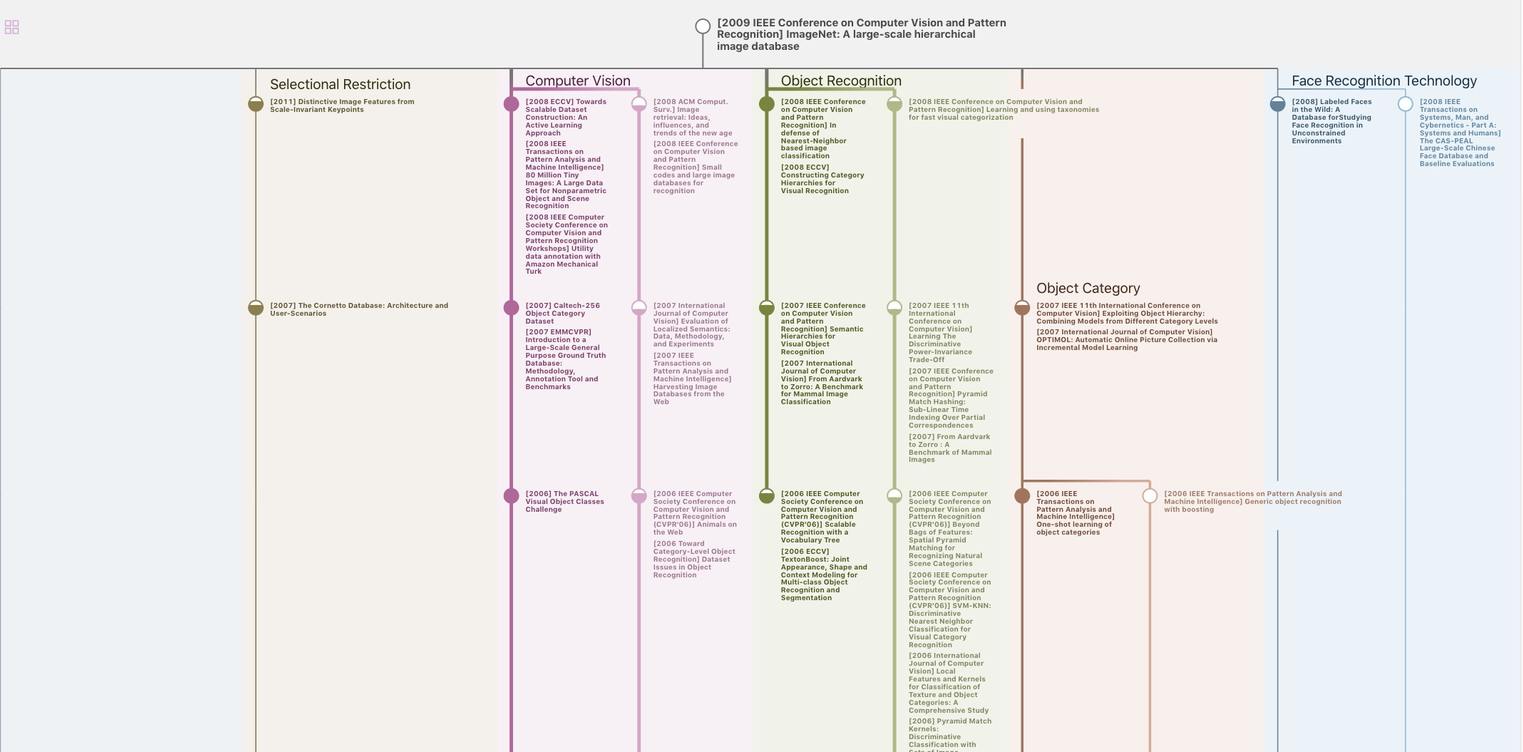
生成溯源树,研究论文发展脉络
Chat Paper
正在生成论文摘要