Linear and nonlinear dimensionality reduction from fluid mechanics to machine learning
arxiv(2023)
摘要
Dimensionality reduction is the essence of many data processing problems, including filtering, data compression, reduced-order modeling and pattern analysis. While traditionally tackled using linear tools in the fluid dynamics community, nonlinear tools from machine learning are becoming increasingly popular. This article, halfway between a review and a tutorial, introduces a general framework for linear and nonlinear dimensionality reduction techniques. Differences and links between autoencoders and manifold learning methods are highlighted, and popular nonlinear techniques such as kernel principal component analysis, isometric feature learning and locally linear embedding are placed in this framework. These algorithms are benchmarked in three classic problems: (a) filtering, (b) identification of oscillatory patterns, and (c) data compression. Their performances are compared against the traditional proper orthogonal decomposition to provide a perspective on their diffusion in fluid dynamics.
更多查看译文
关键词
Dimensionality reduction,kernel PCA,ISOMAPs,locally linear embedding
AI 理解论文
溯源树
样例
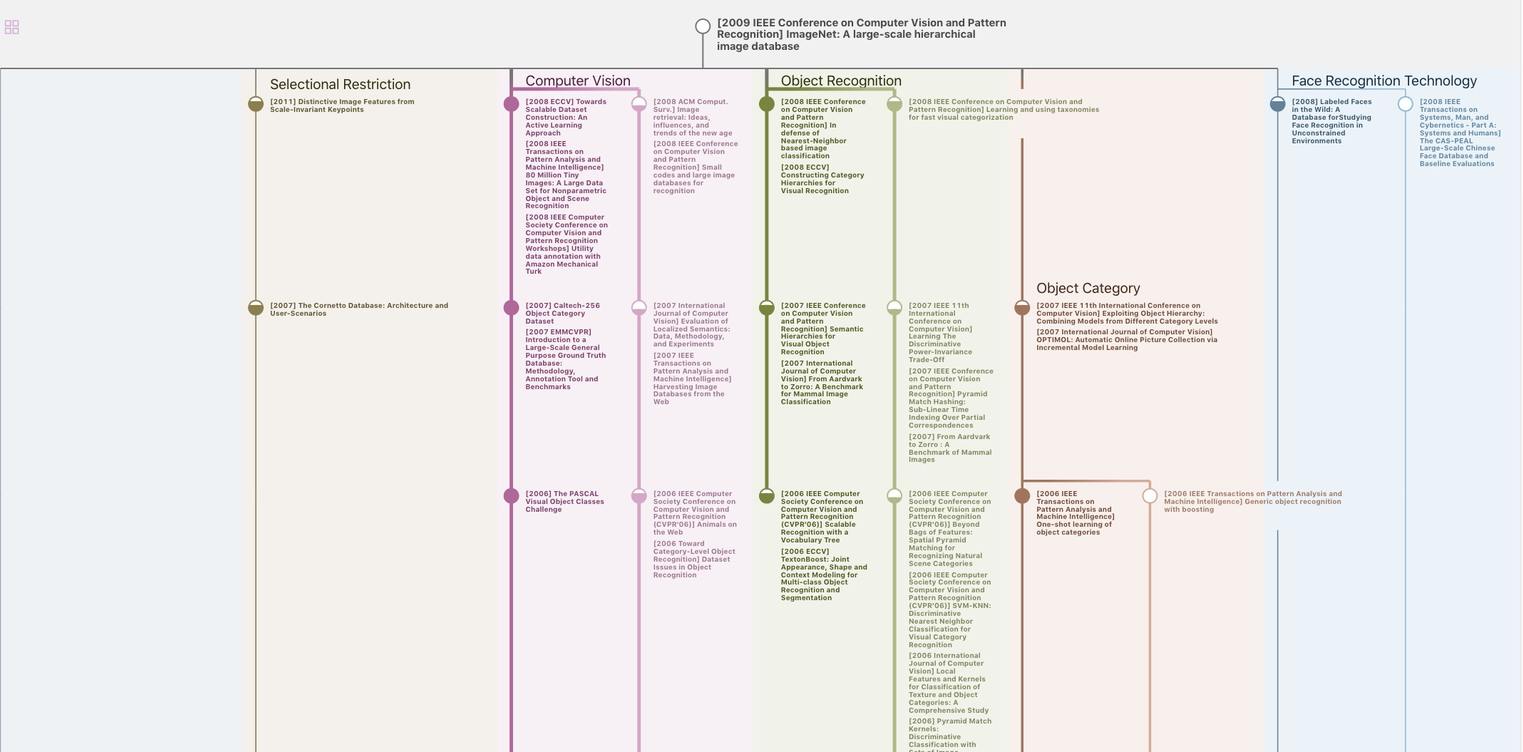
生成溯源树,研究论文发展脉络
Chat Paper
正在生成论文摘要