EGDE-Net: A building change detection method for high-resolution remote sensing imagery based on edge guidance and differential enhancement
ISPRS Journal of Photogrammetry and Remote Sensing(2022)
摘要
Buildings are some of the basic spatial elements of a city. Changes in the spatial distributions of buildings are of great significance for urban planning and monitoring illegal construction. Building change detection (CD) with high-resolution remote sensing images based on deep learning can be used to quickly identify large-scale spatial distribution changes, saving many workforce and material resources. However, existing CD networks mainly focus on regional accuracy, ignoring the importance of accurate boundary identification. It is often difficult for CD networks to achieve accurate boundary segmentation, especially for dense and continuously distributed buildings. In addition, due to the inconsistencies among classes and the discontinuities within classes, it is difficult for CD networks to obtain complete change results. In response to the above problems, a novel method called EGDE-Net that focuses on boundary accuracy and change region integrity is proposed in this paper. First, an edge-guided Transformer block is designed to encode dual-branch networks for EGDE-Net; this block combines a hierarchical Transformer with an edge-aware module (EAM) for long-range context modeling and feature refinement. Second, a feature differential enhancement module (FDEM) is developed to learn highly discriminative change feature maps by exploiting the differences between bitemporal features. In addition, feature maps are recovered through multiple upsampling operations and dilated asymmetric modules (DAMs) in the decoding part of the network. Finally, prior information for boundaries and change information are jointly used to implement a supervision process and obtain the optimal model. The proposed EGDE-Net achieves better results based on the WHU building CD dataset and LEVIR-CD dataset than do similar methods. Notably, F1 scores of 93.02% and 90.05% and intersection over union (IoU) scores of 86.96% and 81.91% are obtained for these two datasets.
更多查看译文
关键词
Building change detection,Transformer,Edge guidance,Feature fusion
AI 理解论文
溯源树
样例
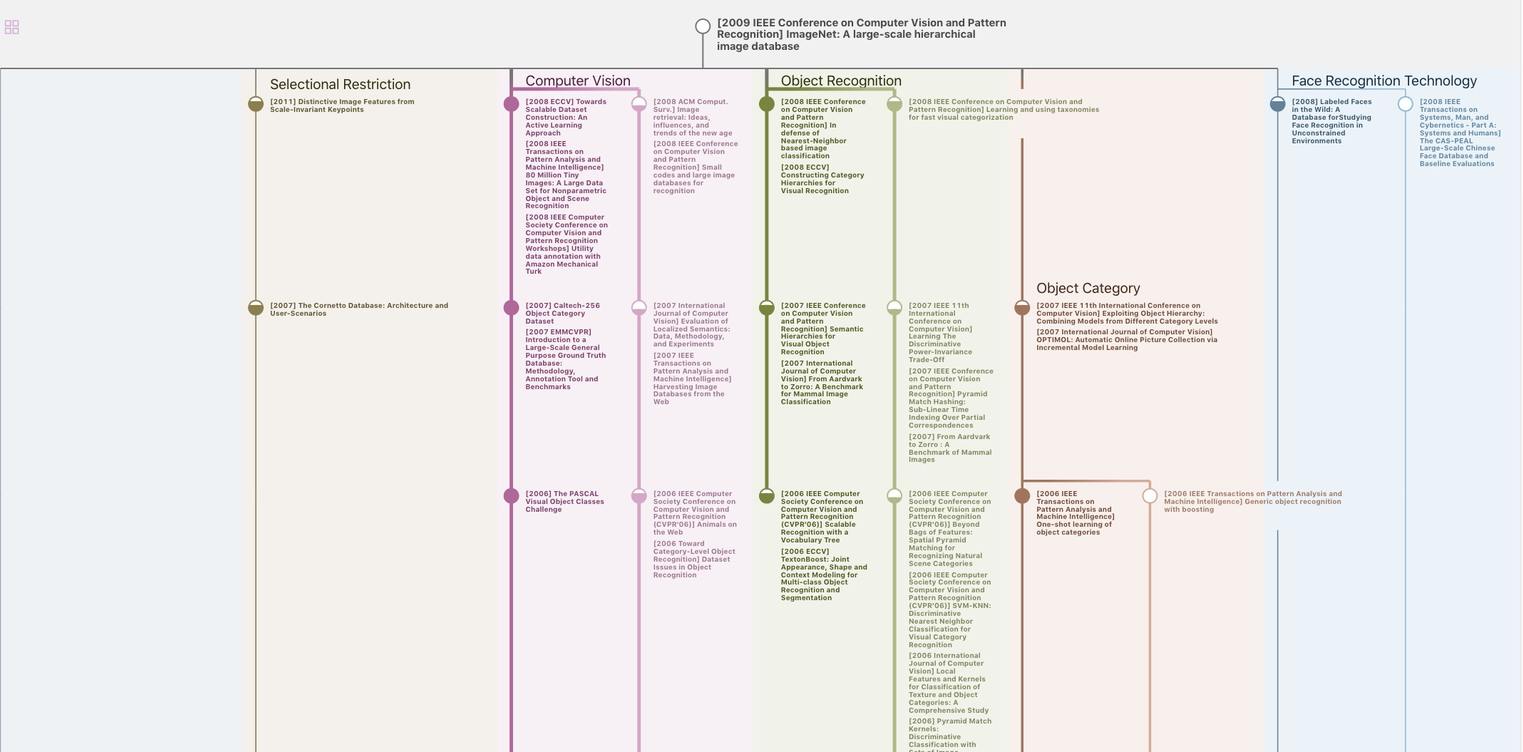
生成溯源树,研究论文发展脉络
Chat Paper
正在生成论文摘要