A Feature-Encoded Physics-Informed Parameter Identification Neural Network for Musculoskeletal Systems.
Journal of biomechanical engineering(2022)
摘要
Identification of muscle-tendon force generation properties and muscle activities from physiological measurements, e.g., motion data and raw surface electromyography (sEMG), offers opportunities to construct a subject-specific musculoskeletal (MSK) digital twin system for health condition assessment and motion prediction. While machine learning approaches with capabilities in extracting complex features and patterns from a large amount of data have been applied to motion prediction given sEMG signals, the learned data-driven mapping is black-box and may not satisfy the underlying physics and has reduced generality. In this work, we propose a feature-encoded physics-informed parameter identification neural network (FEPI-PINN) for simultaneous prediction of motion and parameter identification of human MSK systems. In this approach, features of high-dimensional noisy sEMG signals are projected onto a low-dimensional noise-filtered embedding space for the enhancement of forwarding dynamics prediction. This FEPI-PINN model can be trained to relate sEMG signals to joint motion and simultaneously identify key MSK parameters. The numerical examples demonstrate that the proposed framework can effectively identify subject-specific muscle parameters and the trained physics-informed forward-dynamics surrogate yields accurate motion predictions of elbow flexion-extension motion that are in good agreement with the measured joint motion data.
更多查看译文
关键词
data-driven computing,feature-encoding,musculoskeletal system,parameter identification,physics-informed neural networks,surface electromyography
AI 理解论文
溯源树
样例
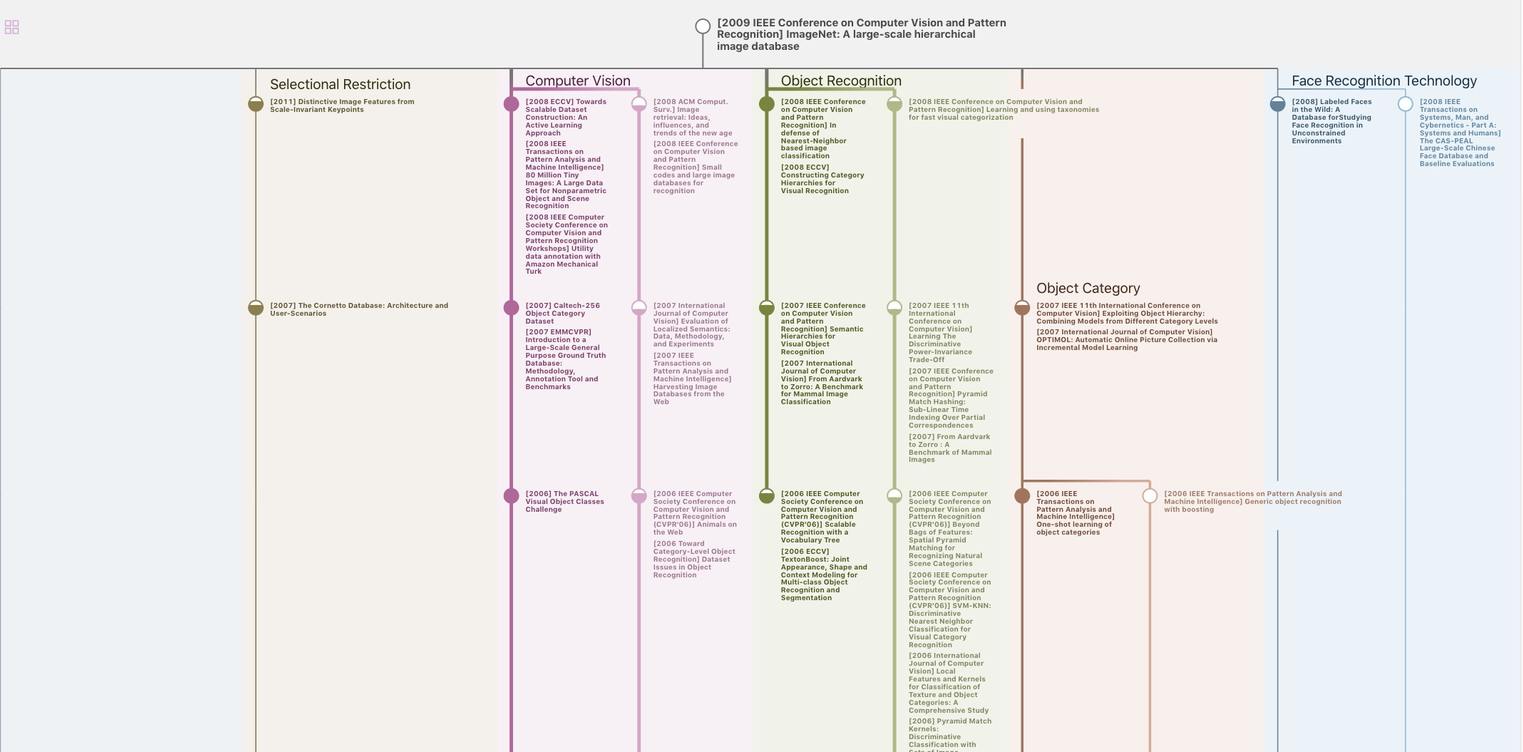
生成溯源树,研究论文发展脉络
Chat Paper
正在生成论文摘要