Privileged Information Learning with Weak Labels
SSRN Electronic Journal(2022)
摘要
Privileged information learning is proposed to construct the classifier by incorporating privileged knowledge. At present, most of the privileged information learning methods assume that the instance is accurately labeled. However, in real-world applications, an instance may be weakly labeled. In this paper, we propose a novel privileged information learning method with weak labels (PLWB). The hypothesis of our work is that an instance may be annotated by a number of labelers and different labelers may give different labels to this instance due to distinct professional knowledge and subjective factors. It leads to ambiguous labels of instances, namely weak labels. To solve this problem, our methodology is to give each labeler a weight and incorporate these weights into a privileged information learning model. Our technique is to employ a heuristic framework to optimize the labeler weights and the privileged information learning model jointly. The existing privileged information learning methods do not consider the weak label problem, and assign an equal or random weight to each labeler. Our work is different from these methods. The novelty and theoretical contribution is that this is the first work to deal with the weak label problem in privileged information learning. The merit is that we assign an unknown weight to each labeler and solve the optimal values of these weights in the optimization process, such that the performance of the learning model can be improved with the optimal labeler weights. In the experiments, the tool that we use is MATLAB, in which we implement our algorithm. The experimental datasets include one handwritten categorization dataset, two image classification datasets (i.e., Animals-with-Attributes dataset and Caltech-101 dataset), and one disease diagnosis dataset (i.e., Alzheimer’s Disease Neuroimaging Initiative dataset), in which the number of instances used is 2000, 6180, 8677 and 202, respectively. The obtained results are that: (1) by optimizing the labeler weights, the proposed PLWB method obtains explicitly higher classification accuracy than the existing privileged information learning methods; (2) PLWB has relatively higher training time since it needs to solve the labeler weights in the optimization process.
更多查看译文
关键词
Privileged information learning,Weak label,Support vector machine,Heuristic framework,Labeler re-weighting
AI 理解论文
溯源树
样例
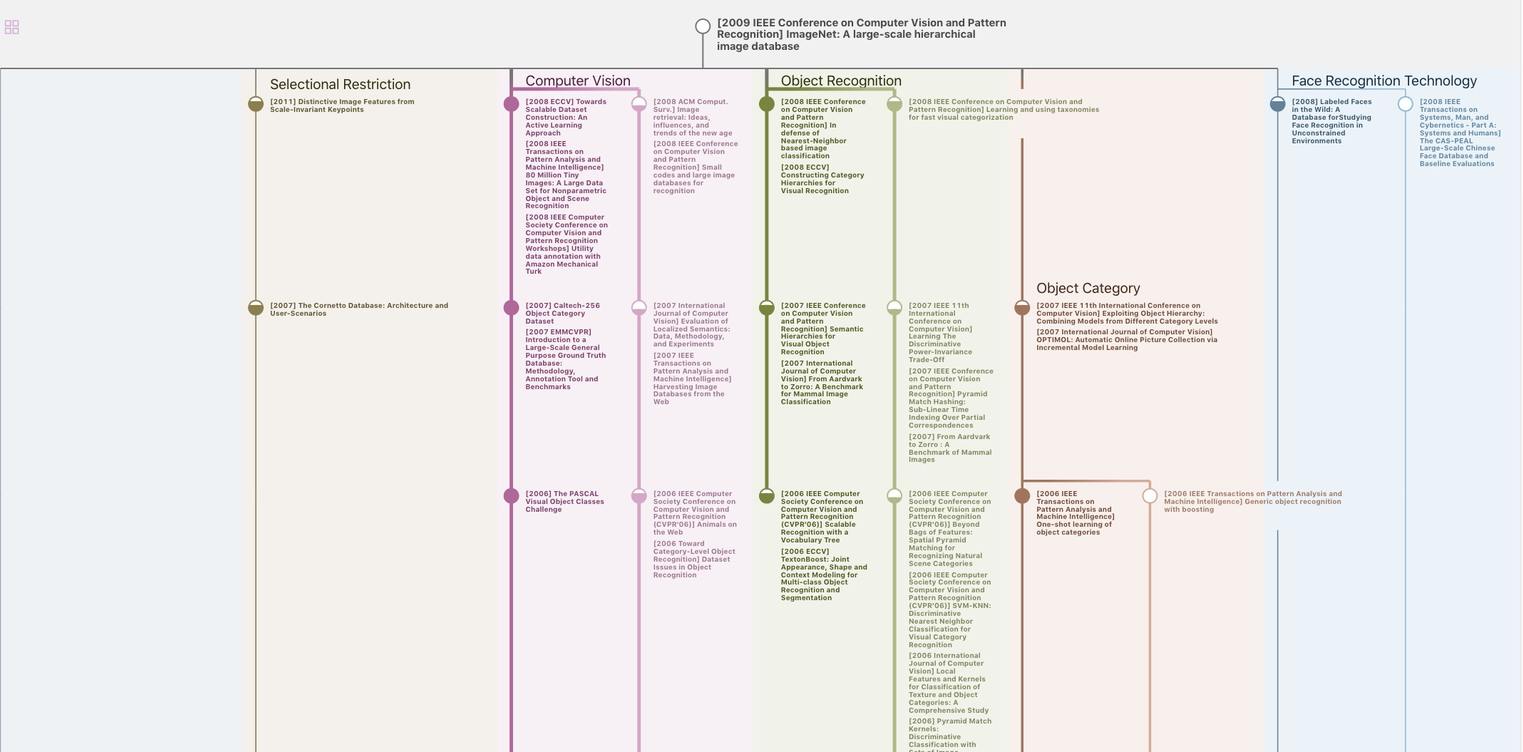
生成溯源树,研究论文发展脉络
Chat Paper
正在生成论文摘要