Solving nonequilibrium statistical mechanics by evolving variational autoregressive networks
arxiv(2022)
摘要
Nonequilibrium statistical mechanics inherits the challenges of the equilibrium, including accurately describing the joint distribution of a large number of configurations. It also poses new challenges as the joint distribution evolves over time. While a number of methods have been proposed, e.g., tensor networks for one-dimensional lattices, we lack a method for arbitrary finite time in higher dimensions. In this work, we propose a general approach to study the time evolution of nonequilibrium systems in statistical mechanics by leveraging variational autoregressive networks, which offers an efficient computation on normalized probabilities of configurations and the dynamical partition function, i.e., the moment generating function of dynamical observables. We apply the approach to a prototype model of nonequilibrium statistical mechanics, the kinetically constrained models of structural glasses up to three dimensions. The results reveal the dynamical phase diagram versus time and counting field, as well as new scaling relations. Our approach paves the way toward solving nonequilibrium statistical mechanics based on modern machine learning.
更多查看译文
关键词
autoregressive networks,statistical mechanics,nonequilibrium
AI 理解论文
溯源树
样例
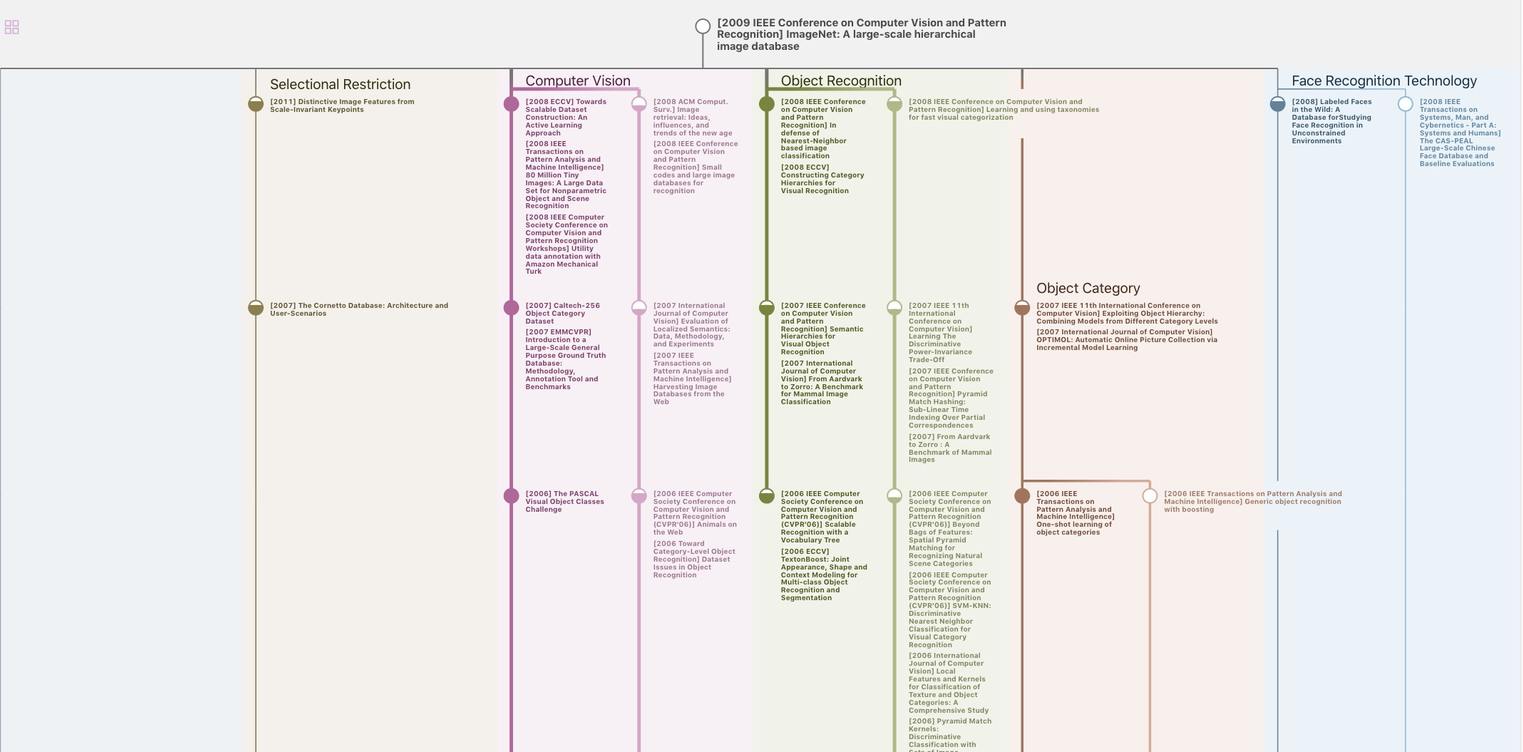
生成溯源树,研究论文发展脉络
Chat Paper
正在生成论文摘要