Crop type discrimination using Geo-Stat Endmember extraction and machine learning algorithms
ADVANCES IN SPACE RESEARCH(2024)
摘要
The identification of crop diversity in today's world is very crucial to ensure adaptation of the crop with changing climate for better productivity as well as food security. Towards this, Hyperspectral Remote Sensing (HRS) is an efficient technique that offers the opportunity to discriminate crop types based on morphological as well as physiological features due to availability of contiguous spectral bands. The current work utilized the benefits of Airborne Visible Infrared Imaging Spectrometer-New Generation (AVIRIS-NG) data and explored the techniques for classification and identification of crop types. The endmembers were identified using the Geo-Stat End member Extraction (GSEE) algorithm for pure pixels identification and to generate the spectral library of the different crop types. Spectral feature comparison was done among AVIRIS-NG, Analytical Spectral Device (ASD)-Spectroradiometer and Continuum Removed (CR) spectra. The best-fit spectra obtained with the Reference ASD-Spectroradiometer and Pure Pixel spectral library were then used for crop discrimination using the ten supervised classifiers namely Spectral Angle Mapper (SAM), Spectral Information Divergence (SID), Support Vector Machine (SVM), Minimum Distance Classifier (MDC), Binary Encoding, deep learning-based Convolution Neural Network (CNN) and different algorithms of Ensemble learning such as Tree Bag, AdaBoost (Adaptive Boosting), Discriminant and RUSBoost (Random Under Sampling). In total, nine crop types were identified, namely, wheat, maize, tobacco, sorghum, linseed, castor, pigeon pea, fennel and chickpea. The performance evaluation of the classifiers was made using various metrics like Overall Accuracy, Kappa Coefficient, Precision, Recall and F1 score. The classifier 2D-CNN was found to be the best with Overall Accuracy, Kappa Coefficient, Precision, Recall and F1 score values of 89.065 %, 0.871, 87.565%, 89.541% and 88.678% respectively. The output of this work can be utilized for large scale mapping of crop types at the species level in a short interval of time with high accuracy. (c) 2022 COSPAR. Published by Elsevier B.V. All rights reserved.
更多查看译文
关键词
Hyperspectral,Crop Discrimination,Endmember Extraction,Continuum Removal,Supervised Classification
AI 理解论文
溯源树
样例
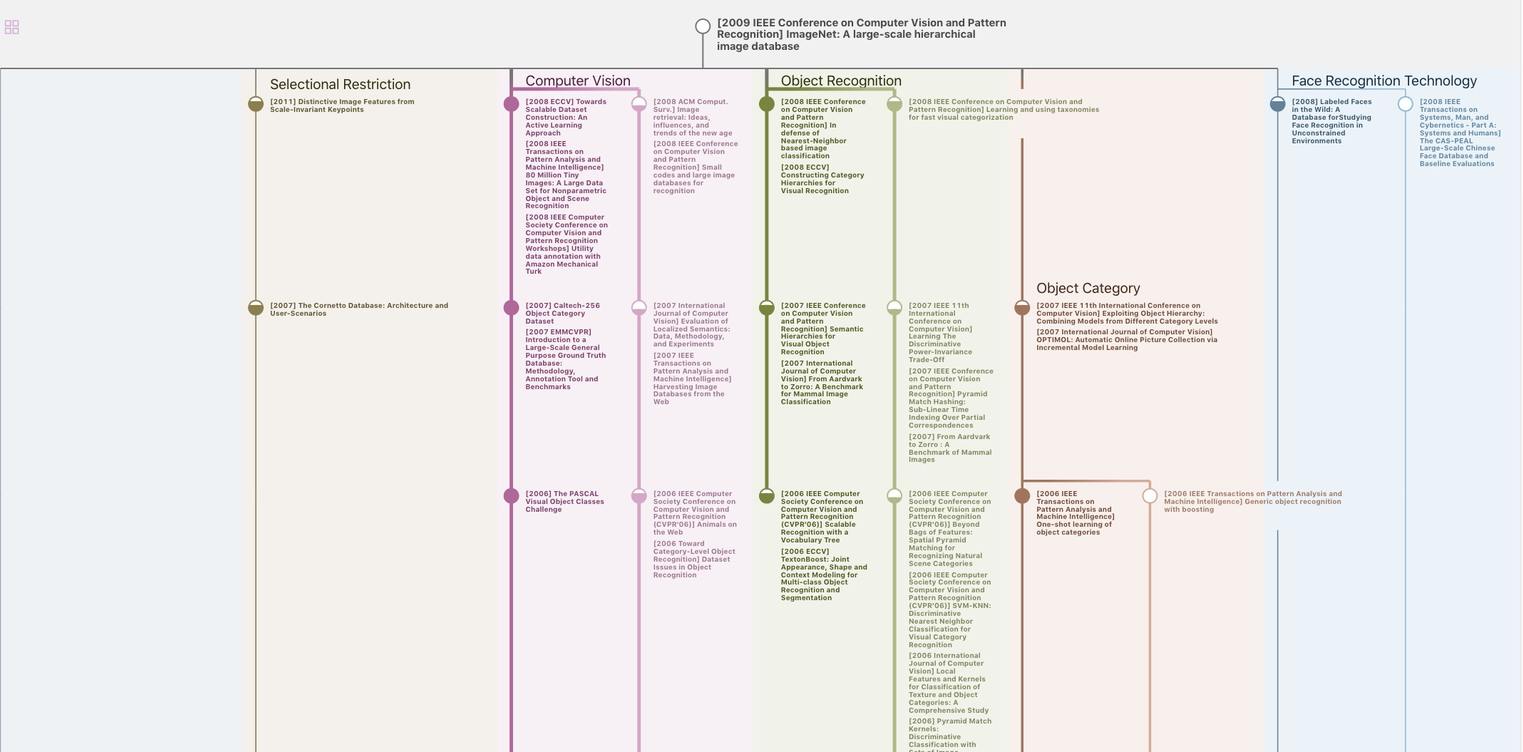
生成溯源树,研究论文发展脉络
Chat Paper
正在生成论文摘要