Temporally-Consistent Annual Land Cover from Landsat Time Series in the Southern Cone of South America
REMOTE SENSING(2022)
摘要
The impact of land cover change across the planet continues to necessitate accurate methods to detect and monitor evolving processes from satellite imagery. In this context, regional and global land cover mapping over time has largely treated time as independent and addressed temporal map consistency as a post-classification endeavor. However, we argue that time can be better modeled as codependent during the model classification stage to produce more consistent land cover estimates over long time periods and gradual change events. To produce temporally-dependent land cover estimates-meaning land cover is predicted over time in connected sequences as opposed to predictions made for a given time period without consideration of past land cover-we use structured learning with conditional random fields (CRFs), coupled with a land cover augmentation method to produce time series training data and bi-weekly Landsat imagery over 20 years (1999-2018) across the Southern Cone region of South America. A CRF accounts for the natural dependencies of land change processes. As a result, it is able to produce land cover estimates over time that better reflect real change and stability by reducing pixel-level annual noise. Using CRF, we produced a twenty-year dataset of land cover over the region, depicting key change processes such as cropland expansion and tree cover loss at the Landsat scale. The augmentation and CRF approach introduced here provides a more temporally consistent land cover product over traditional mapping methods.
更多查看译文
关键词
landsat,time series,land cover,conditional random fields,southern cone
AI 理解论文
溯源树
样例
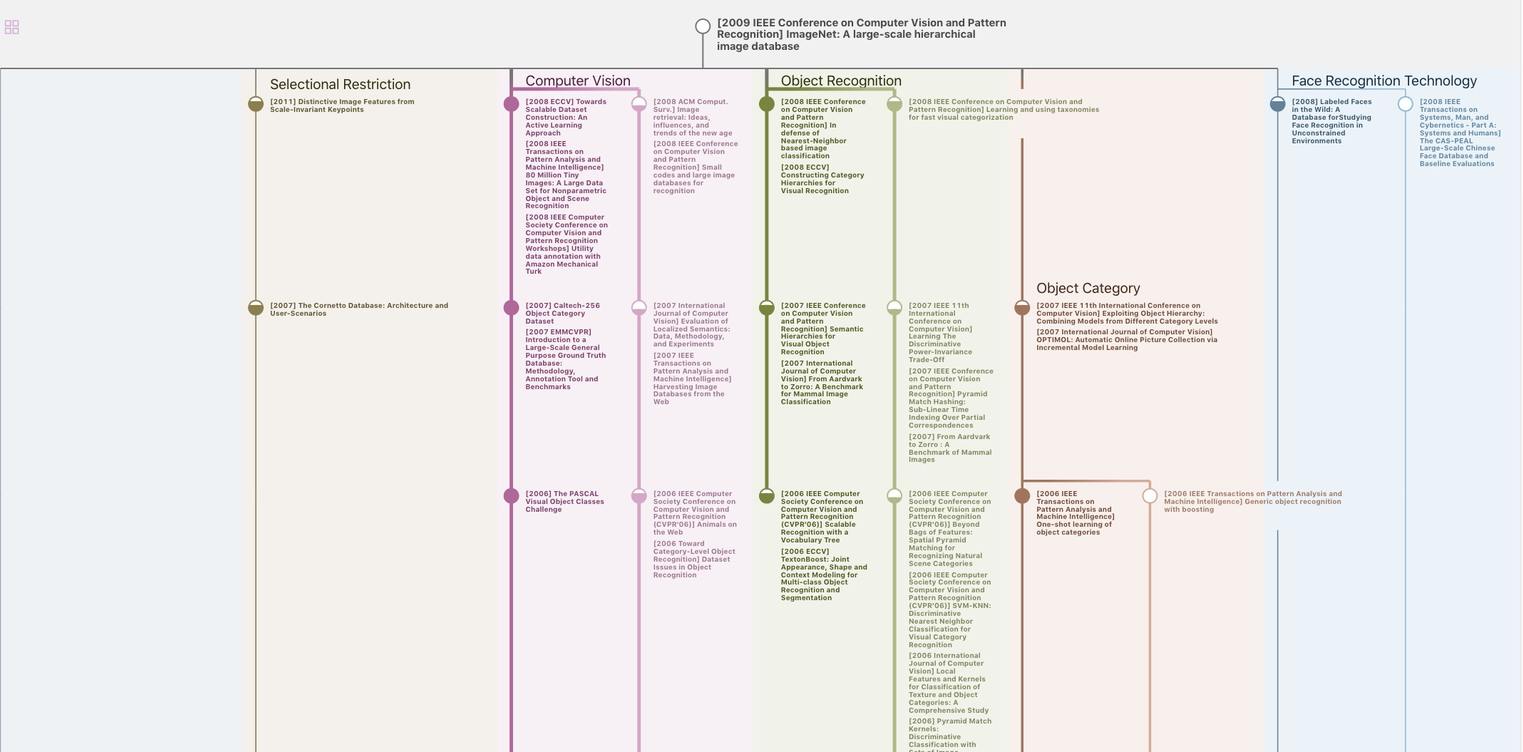
生成溯源树,研究论文发展脉络
Chat Paper
正在生成论文摘要