Multi-policy Grounding and Ensemble Policy Learning for Transfer Learning with Dynamics Mismatch
European Conference on Artificial Intelligence (ECAI)(2022)
摘要
We propose a new transfer learning algorithm between tasks with different dynamics. The proposed algorithm solves an Imitation from Observation problem (IfO) to ground the source environment to the target task before learning an optimal policy in the grounded environment. The learned policy is deployed in the target task without additional training. A particular feature of our algorithm is the employment of multiple rollout policies during training with a goal to ground the environment more globally; hence, it is named as Multi-Policy Grounding (MPG). The quality of final policy is further enhanced via ensemble policy learning. We demonstrate the superiority of the proposed algorithm analytically and numerically. Numerical studies show that the proposed multi-policy approach allows comparable grounding with single policy approach with a fraction of target samples, hence the algorithm is able to maintain the quality of obtained policy even as the number of interactions with the target environment becomes extremely small.
更多查看译文
关键词
ensemble multi-policy learning,transfer learning
AI 理解论文
溯源树
样例
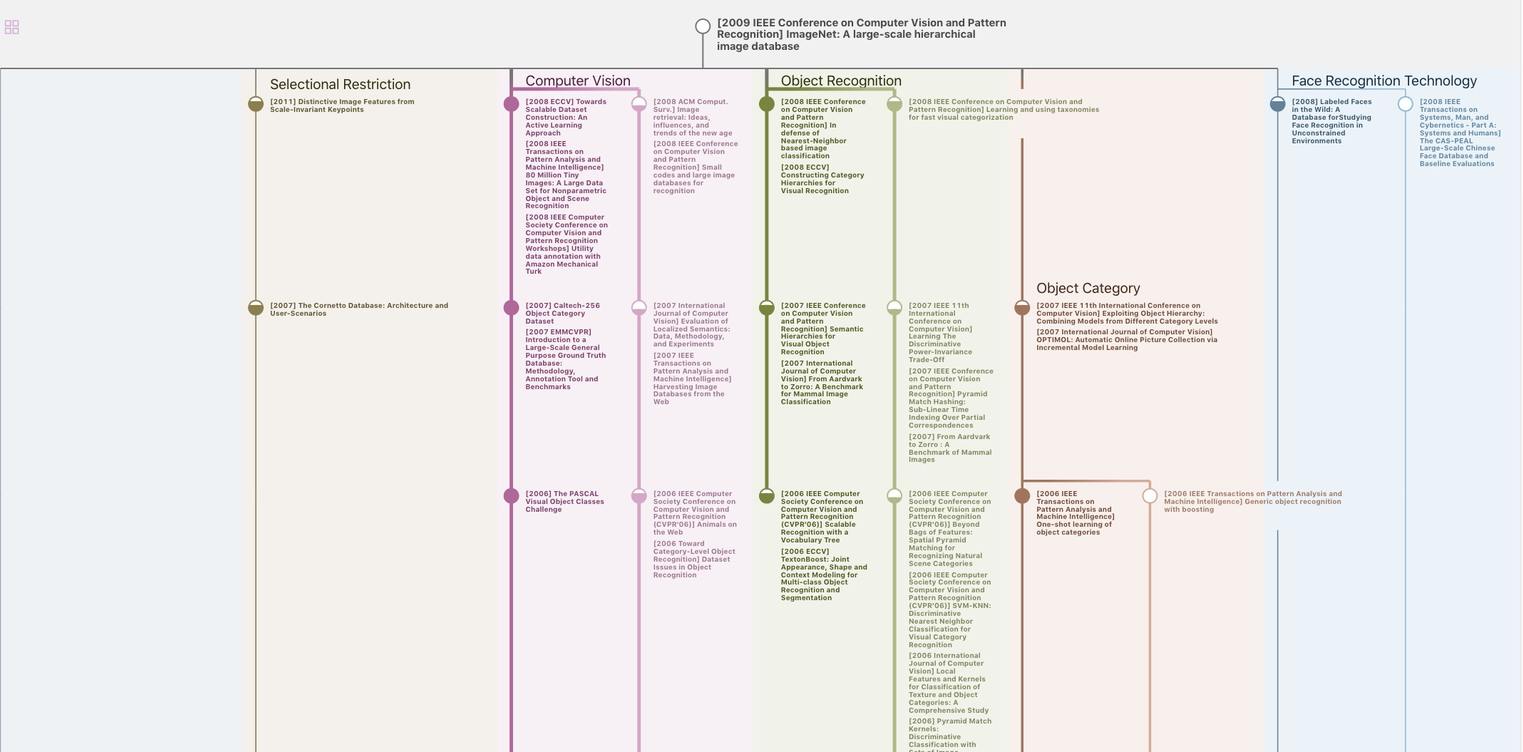
生成溯源树,研究论文发展脉络
Chat Paper
正在生成论文摘要