Active Learning for Testing and Evaluation in Field Robotics: A Case Study in Autonomous, Off-Road Navigation
IEEE International Conference on Robotics and Automation(2022)
摘要
Testing and evaluation of field robotic systems requires both experimentation in representative conditions and human supervision to effectively assess components, manage risk, and interpret results. Due to the complexity of robotic sys-tems, we argue this experimentation should be done adaptively by using insights gained from previous trials. Furthermore, we envision an advisory system that could assist experimenters with selecting trial configurations by learning and accounting for human preferences and risk tolerances; however, formal methods for human decision making in the context of field robotic experimentation remains an open question. In this work, we present and analyze a case study for how decisions were made during the testing and evaluation of an off-road, autonomous navigation system. From the perspective of active learning, we find that Bayesian Optimization is a promising mathematical framework for modeling human decision making in adaptive experimental design of field robotics and that a combination of the EI, KG, and PES acquisition functions would likely be useful for realizing an advisory system.
更多查看译文
关键词
active learning,testing evaluation,field robotics,off-road navigation,field robotic systems,representative conditions,human supervision,robotic sys-tems,previous trials,advisory system,experimenters,trial configurations,learning accounting,human preferences,risk tolerances,field robotic experimentation,autonomous navigation system,modeling human decision making,adaptive experimental design
AI 理解论文
溯源树
样例
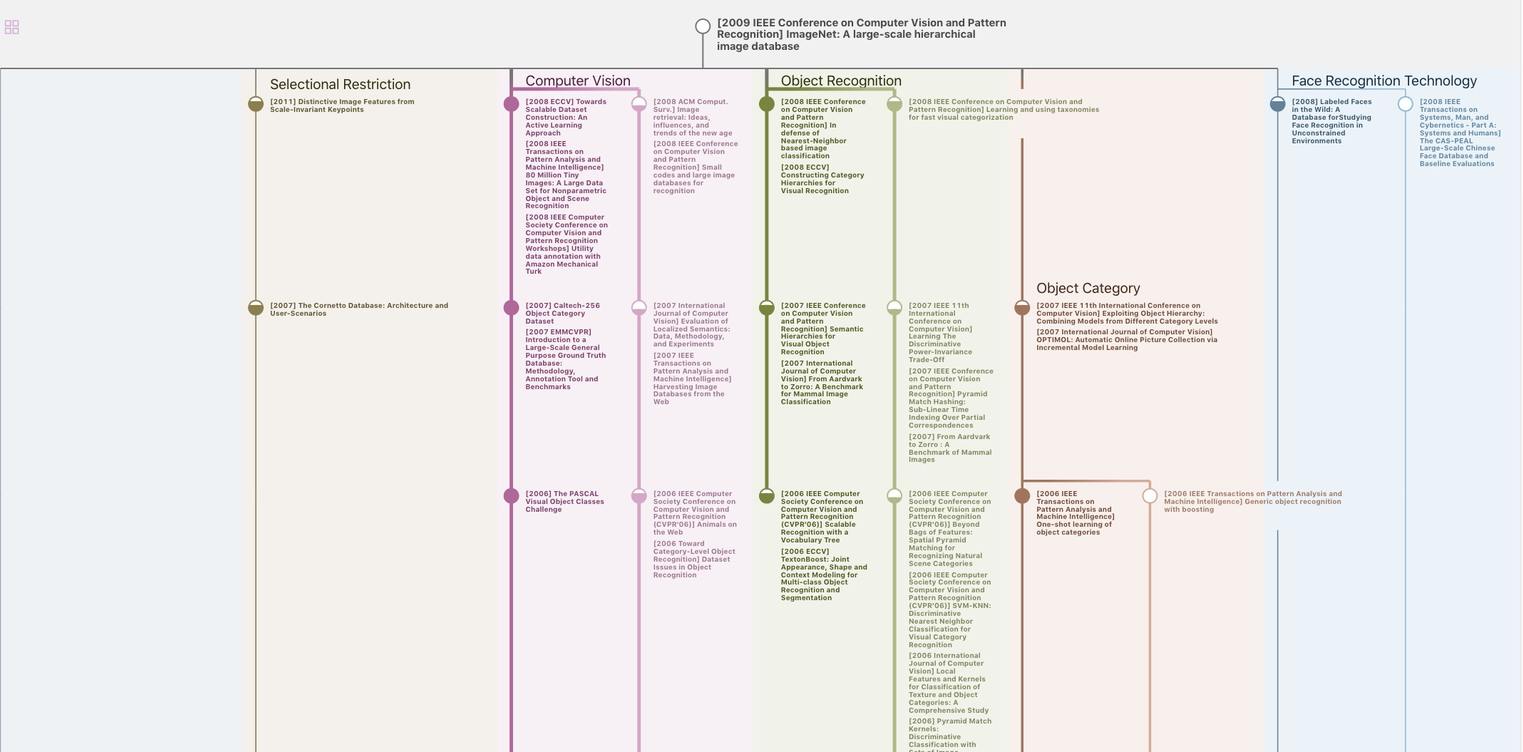
生成溯源树,研究论文发展脉络
Chat Paper
正在生成论文摘要