Improved Deep Unsupervised Hashing with Fine-grained Semantic Similarity Mining for Multi-Label Image Retrieval
European Conference on Artificial Intelligence (ECAI)(2022)
摘要
In this paper, we study deep unsupervised hashing, a critical problem for approximate nearest neighbor research. Most recent methods solve this problem by semantic similarity reconstruction for guiding hashing network learning or contrastive learning of hash codes. However, in multi-label scenarios, these methods usually either generate an inaccurate similarity matrix without reflection of similarity ranking or suffer from the violation of the underlying assumption in contrastive learning, resulting in limited retrieval performance. To tackle this issue, we propose a novel method termed HAMAN, which explores semantics from a fine-grained view to enhance the ability of multi-label image retrieval. In particular, we reconstruct the pairwise similarity structure by matching fine-grained patch features generated by the pre-trained neural network, serving as reliable guidance for similarity preserving of hash codes. Moreover, a novel conditional contrastive learning on hash codes is proposed to adopt self-supervised learning in multi-label scenarios. According to extensive experiments on three multi-label datasets, the proposed method outperforms a broad range of state-of-the-art methods.
更多查看译文
AI 理解论文
溯源树
样例
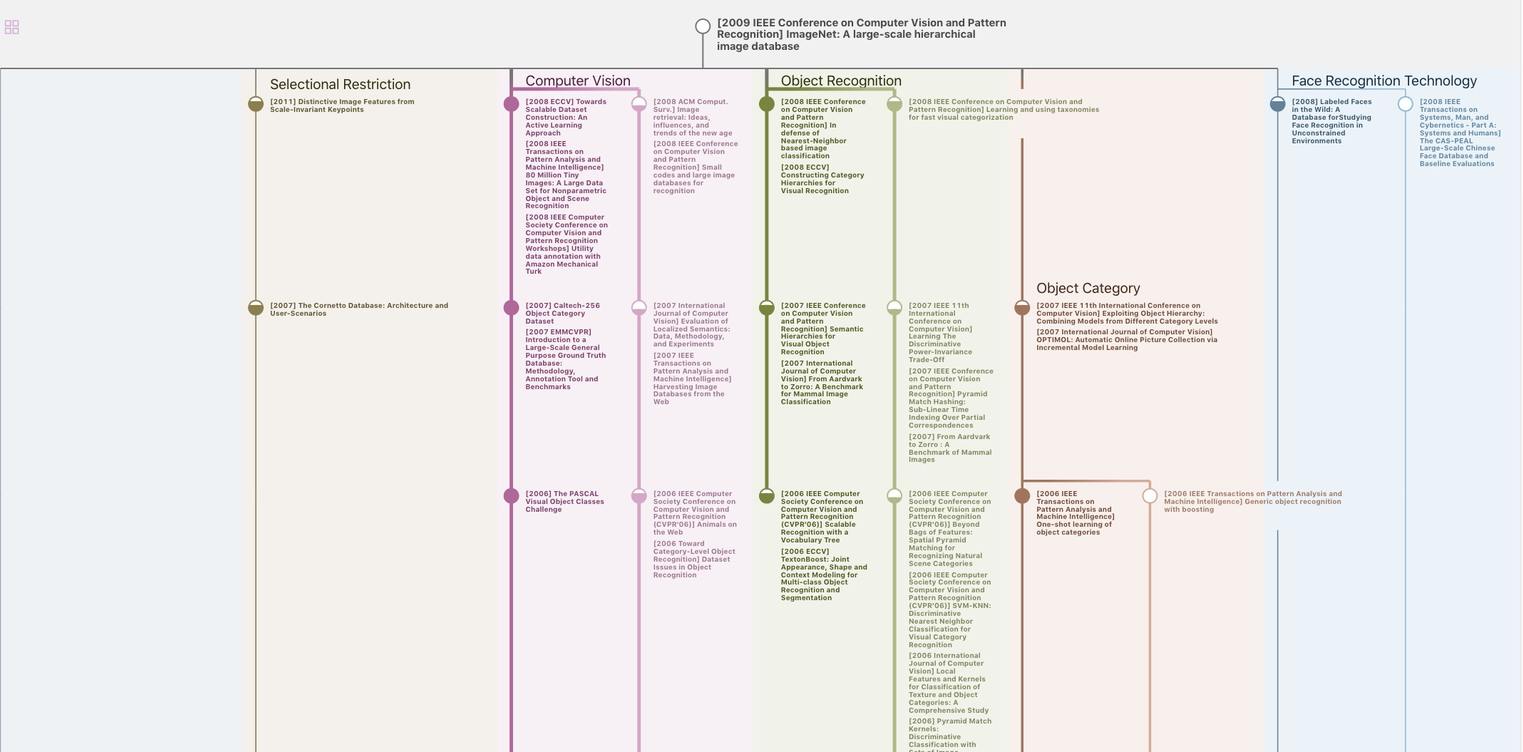
生成溯源树,研究论文发展脉络
Chat Paper
正在生成论文摘要