LaueNN: neural-network-based hkl recognition of Laue spots and its application to polycrystalline materials
JOURNAL OF APPLIED CRYSTALLOGRAPHY(2022)
摘要
A feed-forward neural-network-based model is presented to index, in real time, the diffraction spots recorded during synchrotron X-ray Laue microdiffraction experiments. Data dimensionality reduction is applied to extract physical 1D features from the 2D X-ray diffraction Laue images, thereby making it possible to train a neural network on the fly for any crystal system. The capabilities of the LaueNN model are illustrated through three examples: a two-phase nanostructure, a textured high-symmetry specimen deformed in situ and a polycrystalline low-symmetry material. This work provides a novel way to efficiently index Laue spots in simple and complex recorded images in <1 s, thereby opening up avenues for the realization of real-time analysis of synchrotron Laue diffraction data.
更多查看译文
关键词
synchrotron X-ray Laue microdiffraction, neural networks, hkl recognition
AI 理解论文
溯源树
样例
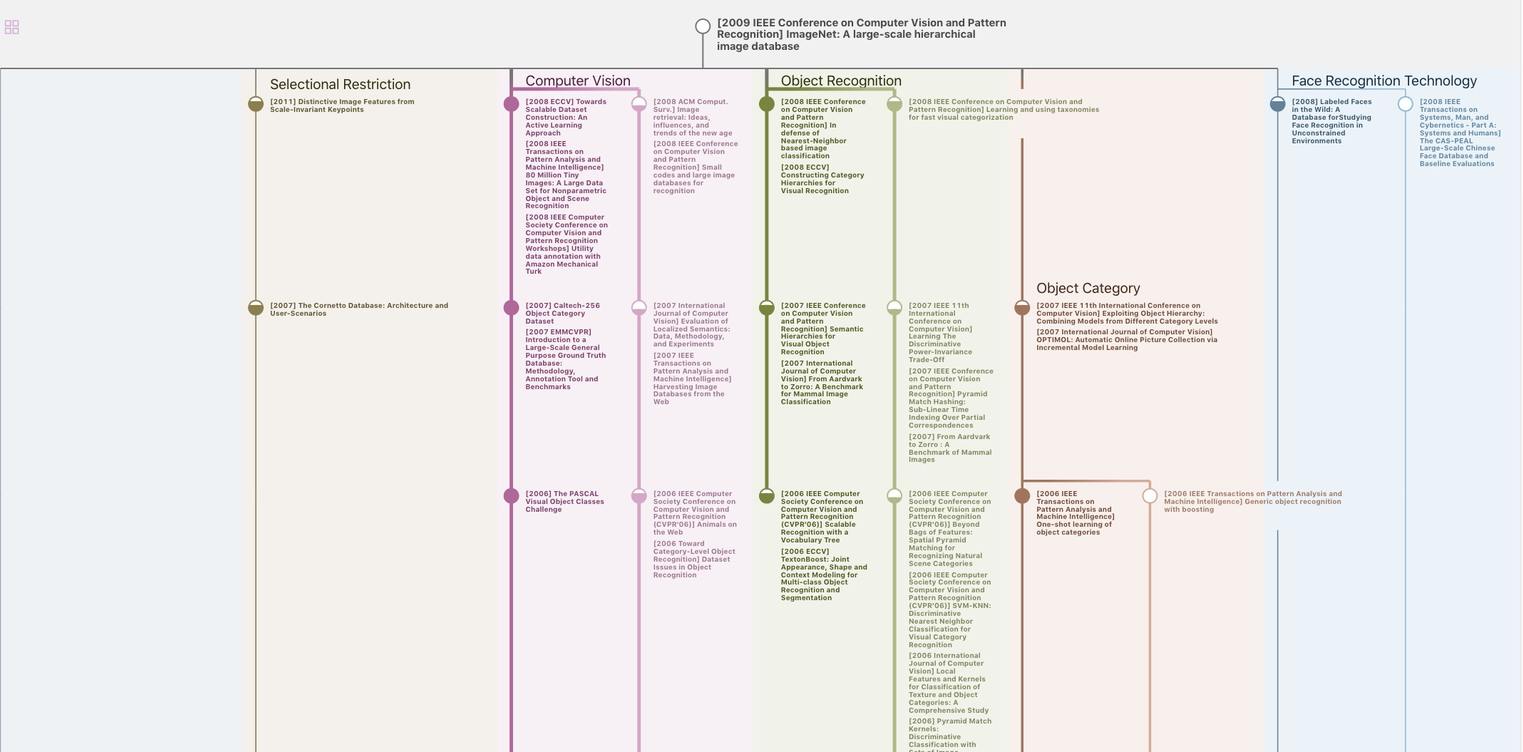
生成溯源树,研究论文发展脉络
Chat Paper
正在生成论文摘要