Communication-Efficient Decentralized Online Continuous DR-Submodular Maximization
PROCEEDINGS OF THE 32ND ACM INTERNATIONAL CONFERENCE ON INFORMATION AND KNOWLEDGE MANAGEMENT, CIKM 2023(2023)
摘要
Maximizing a monotone submodular function is a fundamental task in data mining, machine learning, economics, and statistics. In this paper, we present two communication-efficient decentralized online algorithms for the monotone continuous DR-submodular maximization problem, both of which reduce the number of perfunction gradient evaluations and per-round communication complexity from T-3/2 to 1. The first one, One-shot Decentralized MetaFrank-Wolfe (Mono-DMFW), achieves a ( 1 - 1/e)-regret bound of O(T-4/5). As far as we know, this is the first one-shot and projectionfree decentralized online algorithm for monotone continuous DRsubmodular maximization. Next, inspired by the non-oblivious boosting function [29], we propose the Decentralized Online Boosting Gradient Ascent (DOBGA) algorithm, which attains a (1- 1/e)-regret of O (root T). To the best of our knowledge, this is the first result to obtain the optimal O (root T) against a ( 1- 1/e)-approximation with only one gradient inquiry for each local objective function per step. Finally, various experimental results confirm the effectiveness of the proposed methods.
更多查看译文
关键词
distributed data mining,online learning,submodular maximization
AI 理解论文
溯源树
样例
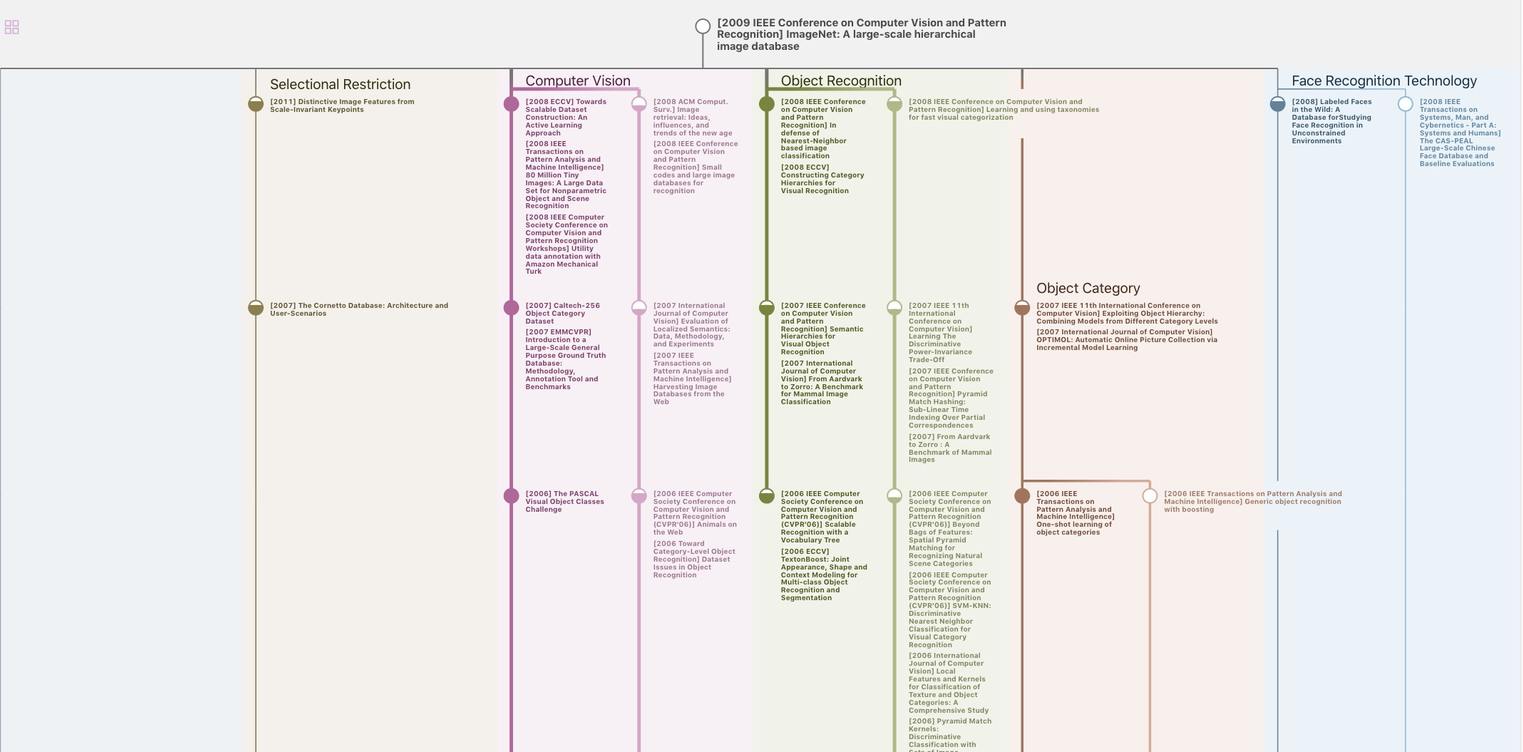
生成溯源树,研究论文发展脉络
Chat Paper
正在生成论文摘要