Speech Representation Disentanglement with Adversarial Mutual Information Learning for One-shot Voice Conversion.
Conference of the International Speech Communication Association (INTERSPEECH)(2022)
摘要
One-shot voice conversion (VC) with only a single target speaker's speech for reference has become a hot research topic. Existing works generally disentangle timbre, while information about pitch, rhythm and content is still mixed together. To perform one-shot VC effectively with further disentangling these speech components, we employ random resampling for pitch and content encoder and use the variational contrastive log-ratio upper bound of mutual information and gradient reversal layer based adversarial mutual information learning to ensure the different parts of the latent space containing only the desired disentangled representation during training. Experiments on the VCTK dataset show the model achieves state-of-the-art performance for one-shot VC in terms of naturalness and intellgibility. In addition, we can transfer characteristics of one-shot VC on timbre, pitch and rhythm separately by speech representation disentanglement. Our code, pre-trained models and demo are available at https://im1eon.github.io/IS2022-SRDVC/.
更多查看译文
关键词
speech representation disentanglement,adversarial mutual information learning,voice,conversion,one-shot
AI 理解论文
溯源树
样例
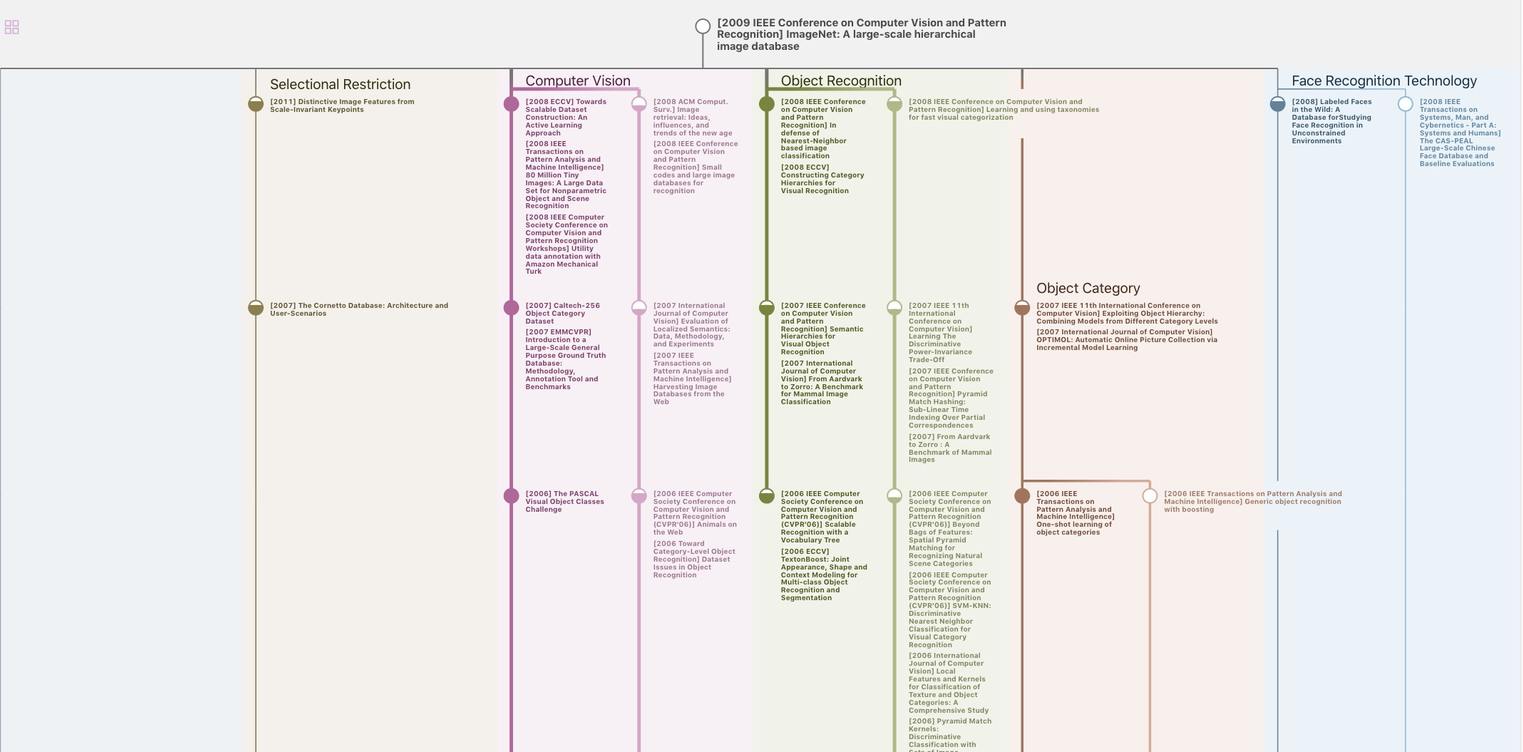
生成溯源树,研究论文发展脉络
Chat Paper
正在生成论文摘要