A Knowledge Query Network Model Based on Rasch Model Embedding for Personalized Online Learning
FRONTIERS IN PSYCHOLOGY(2022)
摘要
The vigorous development of online education has produced massive amounts of education data. How to mine and analyze education big data has become an urgent problem in the field of education and big data knowledge engineering. As for the dynamic learning data, knowledge tracing aims to track learners' knowledge status over time by analyzing the learners' exercise data, so as to predict their performance in the next time step. Deep learning knowledge tracking performs well, but they mainly model the knowledge components while ignoring the personalized information of questions and learners, and provide limited interpretability in the interaction between learners' knowledge status and questions. A context-aware attentive knowledge query network (CAKQN) model is proposed in this paper, which combines flexible neural network models with interpretable model components inspired by psychometric theory. We use the Rasch model to regularize the embedding of questions and learners' interaction tuples, and obtain personalized representations from them. In addition, the long-term short-term memory network and monotonic attention mechanism are used to mine the contextual information of learner interaction sequences and question sequences. It can not only retain the ability to model sequences, but also use the monotonic attention mechanism with exponential decay term to extract the hidden forgetting behavior and other characteristics of learners in the learning process. Finally, the vector dot product is used to simulate the interaction between the learners' knowledge state and questions to improve the interpretability. A series of experimental results on 4 real-world online learning datasets show that CAKQN has the best performance, and its AUC value is improved by an average of 2.945% compared with the existing optimal model. Furthermore, the CAKQN proposed in this paper can not only track learners' knowledge status like other models but also model learners' forgetting behavior. In the future, our research will have high application value in the realization of personalized learning strategies, teaching interventions, and resource recommendations for intelligent online education platforms.
更多查看译文
关键词
personalized education, deep learning, knowledge tracking, forgetting behavior, interpretability
AI 理解论文
溯源树
样例
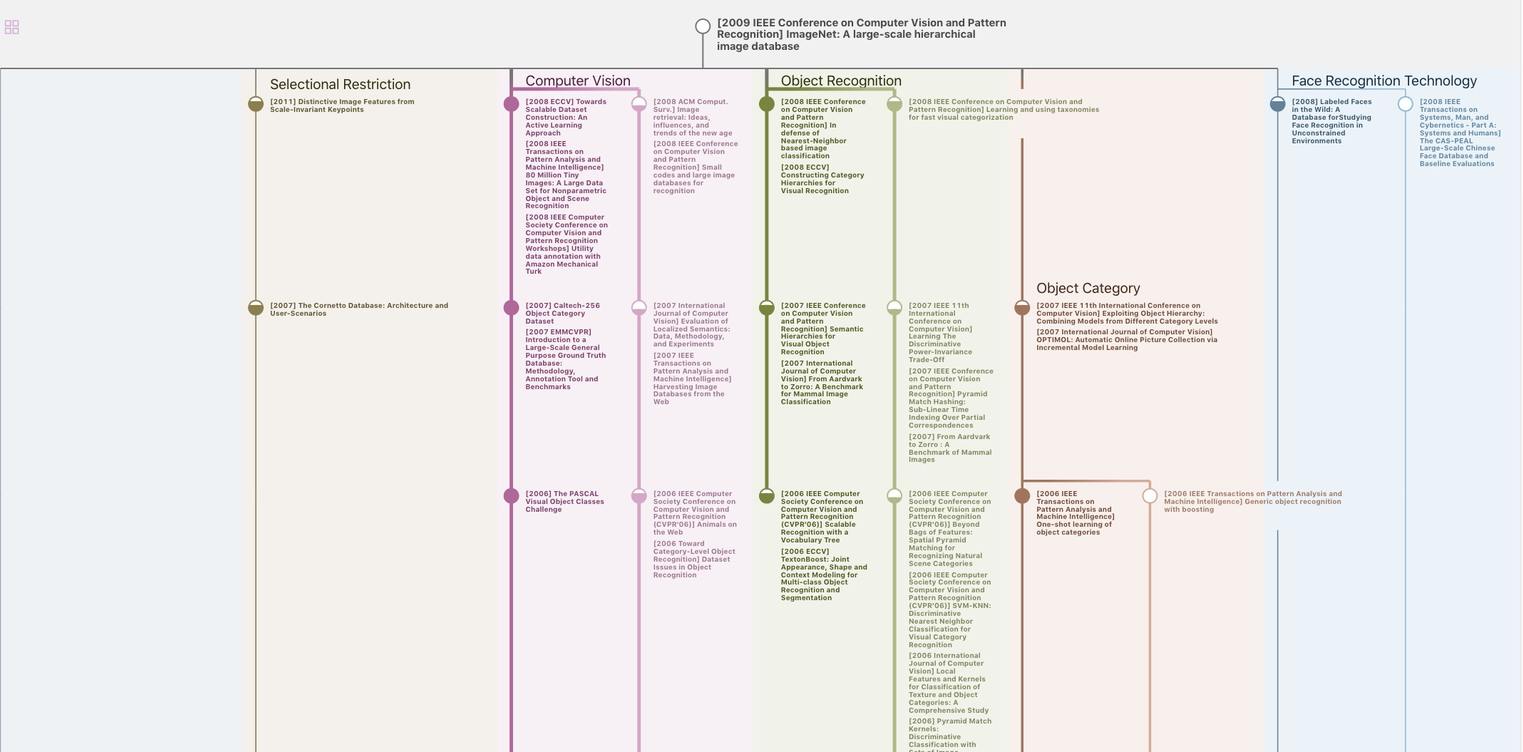
生成溯源树,研究论文发展脉络
Chat Paper
正在生成论文摘要