A Deep Learning Algorithm for Detecting Lytic Bone Lesions of Multiple Myeloma on CT
Skeletal radiology(2022)
摘要
Background Whole-body low-dose CT is the recommended initial imaging modality to evaluate bone destruction as a result of multiple myeloma. Accurate interpretation of these scans to detect small lytic bone lesions is time intensive. A functional deep learning) algorithm to detect lytic lesions on CTs could improve the value of these CTs for myeloma imaging. Our objectives were to develop a DL algorithm and determine its performance at detecting lytic lesions of multiple myeloma. Methods Axial slices (2-mm section thickness) from whole-body low-dose CT scans of subjects with biochemically confirmed plasma cell dyscrasias were included in the study. Data were split into train and test sets at the patient level targeting a 90%/10% split. Two musculoskeletal radiologists annotated lytic lesions on the images with bounding boxes. Subsequently, we developed a two-step deep learning model comprising bone segmentation followed by lesion detection. Unet and “You Look Only Once” (YOLO) models were used as bone segmentation and lesion detection algorithms, respectively. Diagnostic performance was determined using the area under the receiver operating characteristic curve (AUROC). Results Forty whole-body low-dose CTs from 40 subjects yielded 2193 image slices. A total of 5640 lytic lesions were annotated. The two-step model achieved a sensitivity of 91.6% and a specificity of 84.6%. Lesion detection AUROC was 90.4%. Conclusion We developed a deep learning model that detects lytic bone lesions of multiple myeloma on whole-body low-dose CTs with high performance. External validation is required prior to widespread adoption in clinical practice.
更多查看译文
关键词
Multiple myeloma,Deep learning,Whole-body low-dose CT,Bone segmentation,Lesion detection
AI 理解论文
溯源树
样例
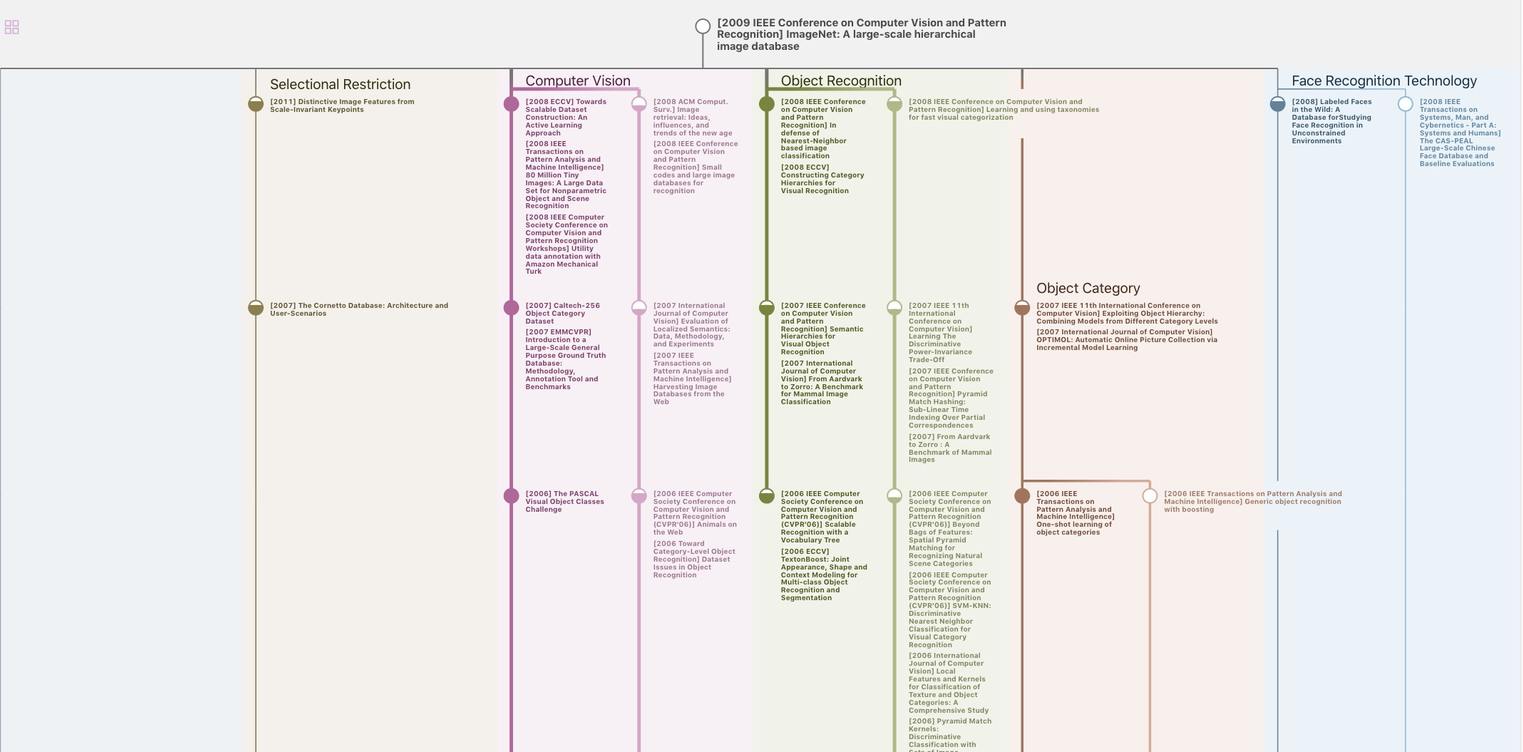
生成溯源树,研究论文发展脉络
Chat Paper
正在生成论文摘要