NeuroPred-CLQ: incorporating deep temporal convolutional networks and multi-head attention mechanism to predict neuropeptides
BRIEFINGS IN BIOINFORMATICS(2022)
摘要
Neuropeptides (NPs) are a particular class of informative substances in the immune system and physiological regulation. They play a crucial role in regulating physiological functions in various biological growth and developmental stages. In addition, NPs are crucial for developing new drugs for the treatment of neurological diseases. With the development of molecular biology techniques, some data-driven tools have emerged to predict NPs. However, it is necessary to improve the predictive performance of these tools for NPs. In this study, we developed a deep learning model (NeuroPred-CLQ) based on the temporal convolutional network (TCN) and multi-head attention mechanism to identify NPs effectively and translate the internal relationships of peptide sequences into numerical features by the Word2vec algorithm. The experimental results show that NeuroPred-CLQ learns data information effectively, achieving 93.6% accuracy and 98.8% AUC on the independent test set. The model has better performance in identifying NPs than the state-of-the-art predictors. Visualization of features using t-distribution random neighbor embedding shows that the NeuroPred-CLQ can clearly distinguish the positive NPs from the negative ones. We believe the NeuroPred-CLQ can facilitate drug development and clinical trial studies to treat neurological disorders.
更多查看译文
关键词
deep learning,Word2vec,neuropeptide prediction,TCN,multi-head attention mechanism
AI 理解论文
溯源树
样例
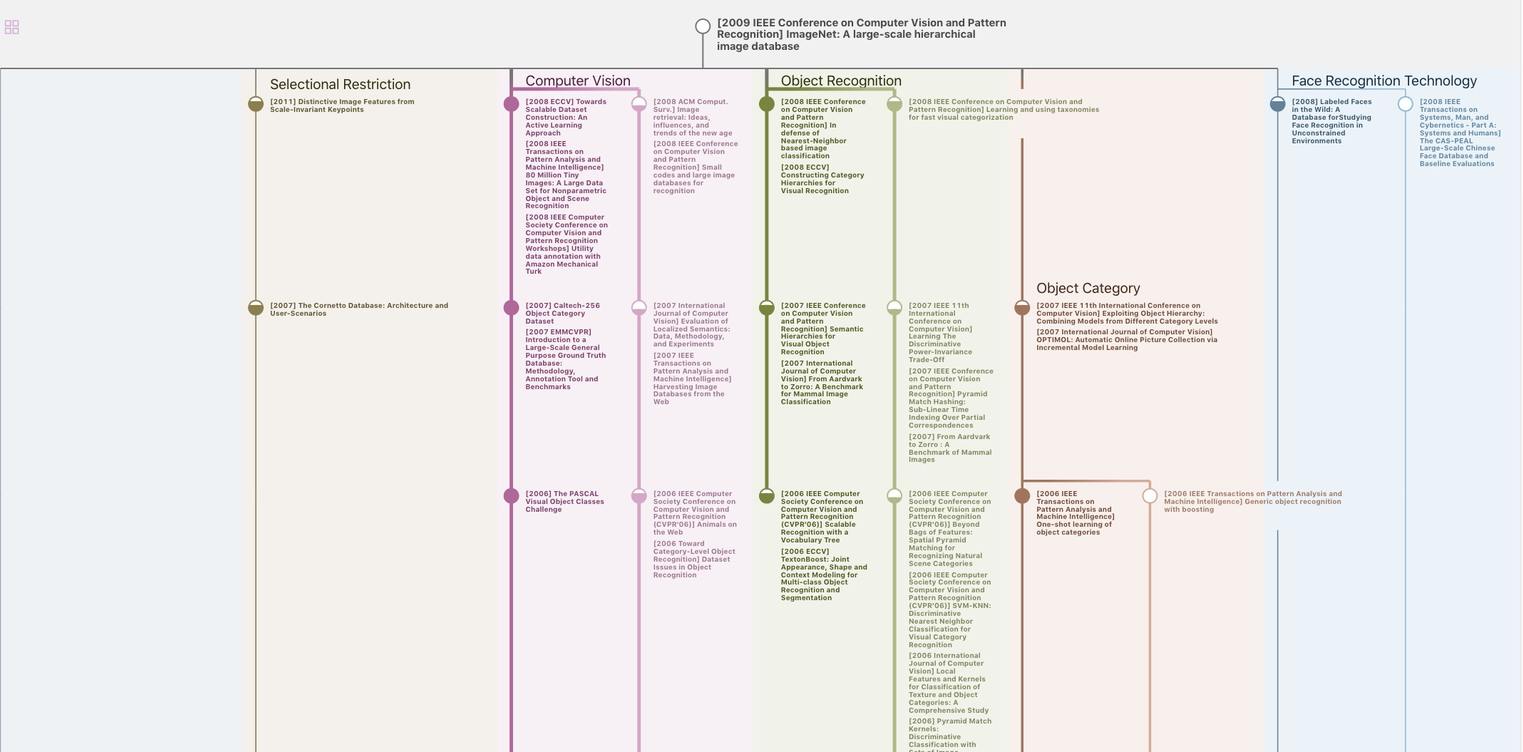
生成溯源树,研究论文发展脉络
Chat Paper
正在生成论文摘要