Demystifying Randomly Initialized Networks for Evaluating Generative Models
AAAI'23/IAAI'23/EAAI'23 Proceedings of the Thirty-Seventh AAAI Conference on Artificial Intelligence and Thirty-Fifth Conference on Innovative Applications of Artificial Intelligence and Thirteenth Symposium on Educational Advances in Artificial Intelligence(2022)
摘要
Evaluation of generative models is mostly based on the comparison between the estimated distribution and the ground truth distribution in a certain feature space. To embed samples into informative features, previous works often use convolutional neural networks optimized for classification, which is criticized by recent studies. Therefore, various feature spaces have been explored to discover alternatives. Among them, a surprising approach is to use a randomly initialized neural network for feature embedding. However, the fundamental basis to employ the random features has not been sufficiently justified. In this paper, we rigorously investigate the feature space of models with random weights in comparison to that of trained models. Furthermore, we provide an empirical evidence to choose networks for random features to obtain consistent and reliable results. Our results indicate that the features from random networks can evaluate generative models well similarly to those from trained networks, and furthermore, the two types of features can be used together in a complementary way.
更多查看译文
关键词
models,networks,randomly
AI 理解论文
溯源树
样例
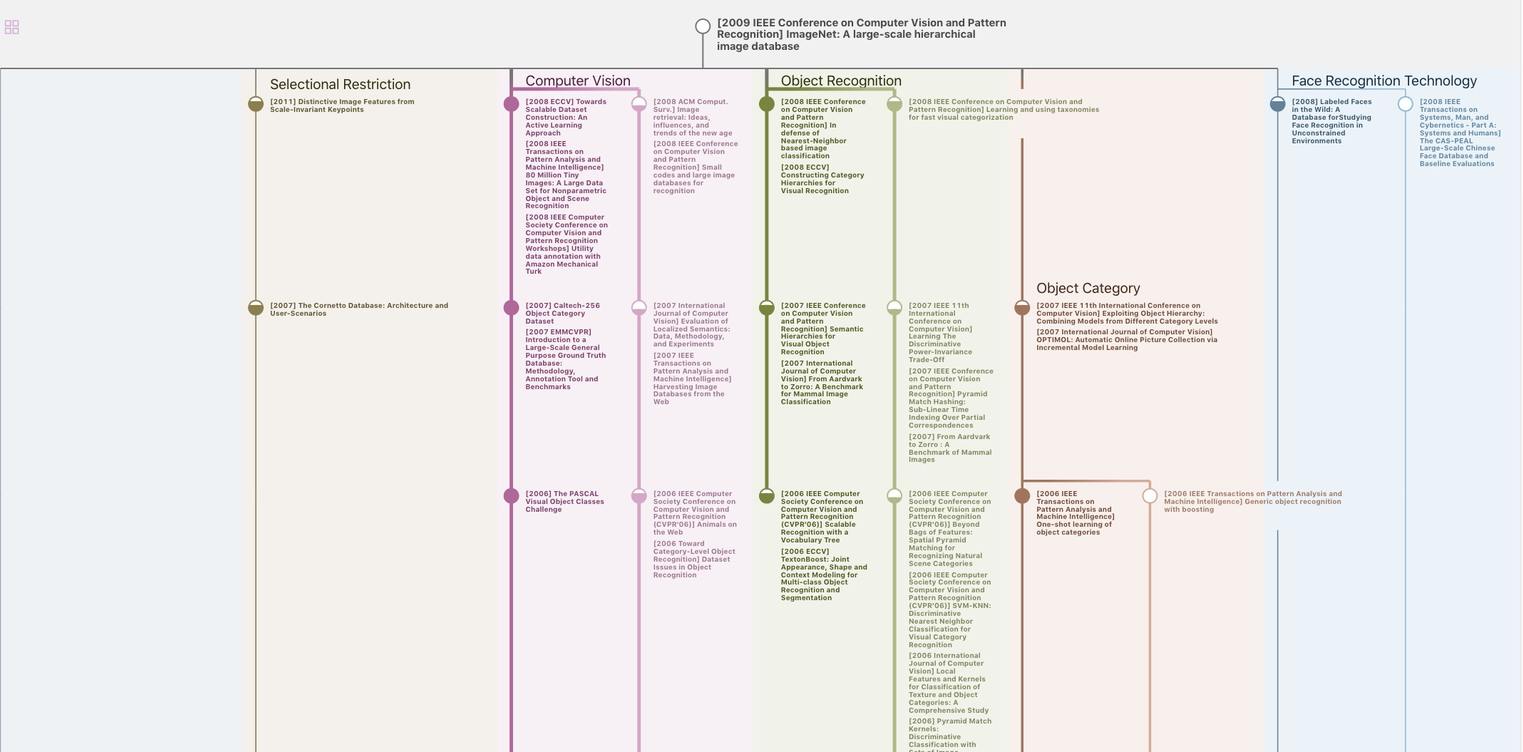
生成溯源树,研究论文发展脉络
Chat Paper
正在生成论文摘要