Variational Graph Author Topic Modeling
KDD '22: Proceedings of the 28th ACM SIGKDD Conference on Knowledge Discovery and Data Mining(2022)
摘要
While Variational Graph Auto-Encoder (VGAE) has presented promising ability to learn representations for documents, most existing VGAE methods do not model a latent topic structure and therefore lack semantic interpretability. Exploring hidden topics within documents and discovering key words associated with each topic allow us to develop a semantic interpretation of the corpus. Moreover, documents are usually associated with authors. For example, news reports have journalists specializing in writing certain type of events, academic papers have authors with expertise in certain research topics, etc. Modeling authorship information could benefit topic modeling, since documents by the same authors tend to reveal similar semantics. This observation also holds for documents published on the same venues. However, most topic models ignore the auxiliary authorship and publication venues. Given above two challenges, we propose a Variational Graph Author Topic Model for documents to integrate both semantic interpretability and authorship and venue modeling into a unified VGAE framework. For authorship and venue modeling, we construct a hierarchical multi-layered document graph with both intra- and cross-layer topic propagation. For semantic interpretability, three word relations (contextual, syntactic, semantic) are modeled and constitute three word sub-layers in the document graph. We further propose three alternatives for variational divergence. Experiments verify the effectiveness of our model on supervised and unsupervised tasks.
更多查看译文
关键词
topic,author,graph
AI 理解论文
溯源树
样例
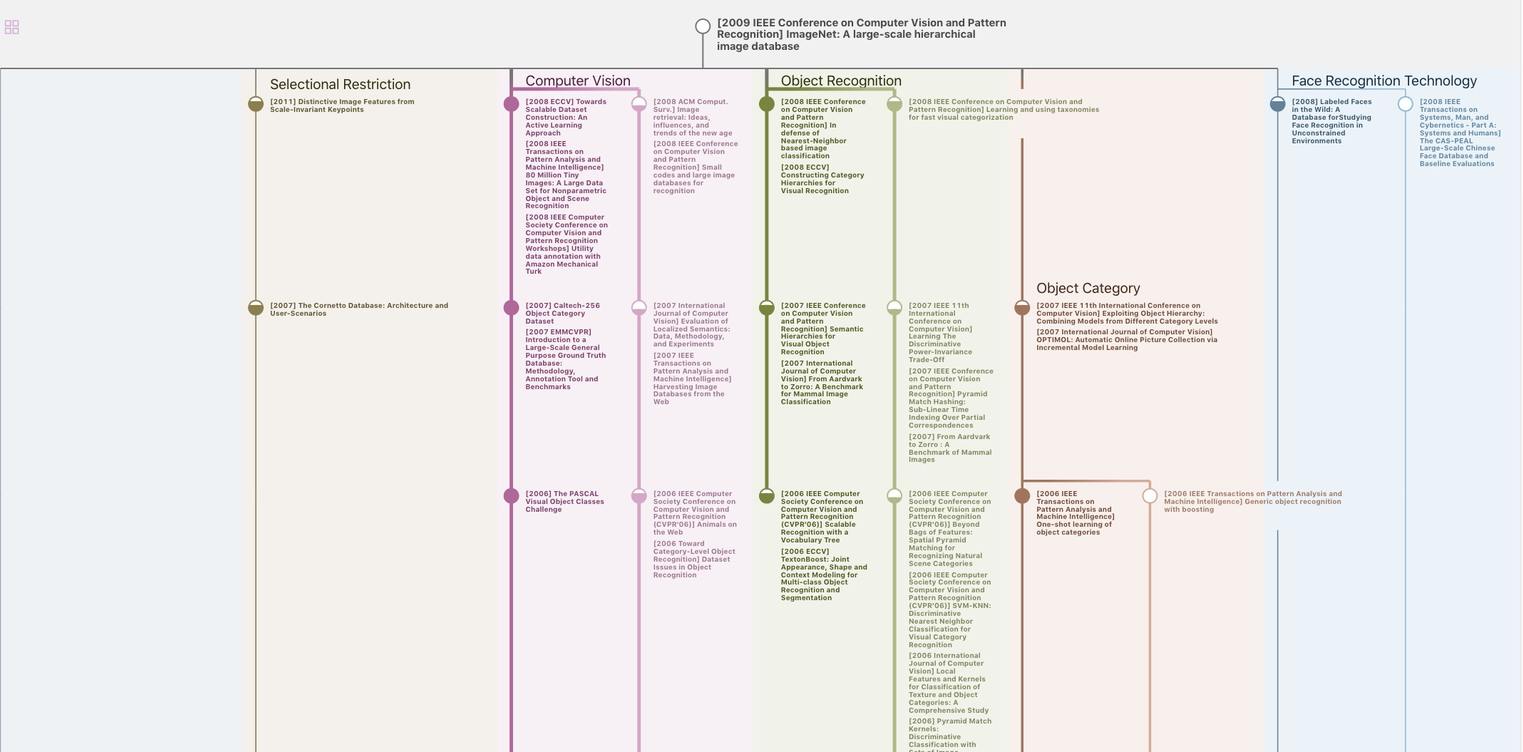
生成溯源树,研究论文发展脉络
Chat Paper
正在生成论文摘要