TARNet: Task-Aware Reconstruction for Time-Series Transformer
KDD '22: Proceedings of the 28th ACM SIGKDD Conference on Knowledge Discovery and Data Mining(2022)
摘要
Time-series data contains temporal order information that can guide representation learning for predictive end tasks (e.g., classification, regression). Recently, there are some attempts to leverage such order information to first pre-train time-series models by reconstructing time-series values of randomly masked time segments, followed by an end-task fine-tuning on the same dataset, demonstrating improved end-task performance. However, this learning paradigm decouples data reconstruction from the end task. We argue that the representations learnt in this way are not informed by the end task and may, therefore, be sub-optimal for the end-task performance. In fact, the importance of different timestamps can vary significantly in different end tasks. We believe that representations learnt by reconstructing important timestamps would be a better strategy for improving end-task performance. In this work, we propose TARNet, Task-Aware Reconstruction Network, a new model using Transformers to learn task-aware data reconstruction that augments end-task performance. Specifically, we design a data-driven masking strategy that uses self-attention score distribution from end-task training to sample timestamps deemed important by the end task. Then, we mask out data at those timestamps and reconstruct them, thereby making the reconstruction task-aware. This reconstruction task is trained alternately with the end task at every epoch, sharing parameters in a single model, allowing the representation learnt through reconstruction to improve end-task performance. Extensive experiments on tens of classification and regression datasets show that TARNet significantly outperforms state-of-the-art baseline models across all evaluation metrics.
更多查看译文
AI 理解论文
溯源树
样例
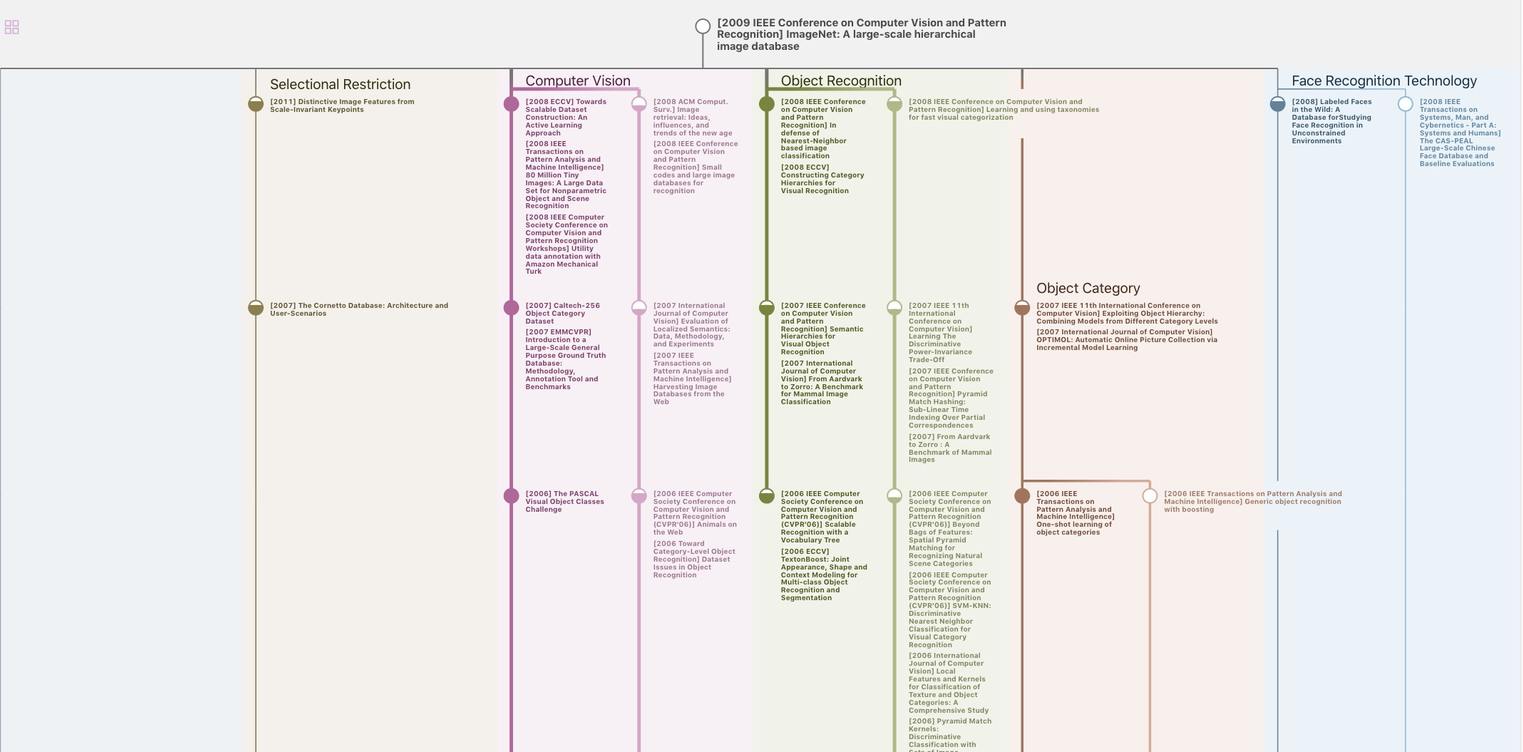
生成溯源树,研究论文发展脉络
Chat Paper
正在生成论文摘要