CausalInt: Causal Inspired Intervention for Multi-Scenario Recommendation
KDD '22: Proceedings of the 28th ACM SIGKDD Conference on Knowledge Discovery and Data Mining(2022)
摘要
Building appropriate scenarios to meet the personalized demands of different user groups is a common practice. Despite various scenario brings personalized service, it also leads to challenges for the recommendation on multiple scenarios, especially the scenarios with limited traffic. To give desirable recommendation service for all scenarios and reduce the cost of resource consumption, how to leverage the information from multiple scenarios to construct a unified model becomes critical. Unfortunately, the performance of existing multi-scenario recommendation approaches is poor since they introduce unnecessary information from other scenarios to target scenario. In this paper, we show it is possible to selectively utilize the information from different scenarios to construct the scenario-aware estimators in a unified model. Specifically, we first do analysis on multi-scenario modeling with causal graph from the perspective of users and modeling processes, and then propose the Causal Inspired Intervention (CausalInt) framework for multi-scenario recommendation. CausalInt consists of three modules: (1) Invariant Representation Modeling module to squeeze out the scenario-aware information through disentangled representation learning and obtain a scenario-invariant representation; (2) Negative Effects Mitigating module to resolve conflicts between different scenarios and conflicts between scenario-specific and scenario-invariant representations via gradient based orthogonal regularization and model-agnostic meta learning, respectively; (3) Inter-Scenario Transferring module designs a novel TransNet to simulate a counterfactual intervention and effectively fuse the information from other scenarios. Offline experiments over two real-world dataset and online A/B test are conducted to demonstrate the superiority of CausalInt.
更多查看译文
关键词
causalint,recommendation,intervention,multi-scenario
AI 理解论文
溯源树
样例
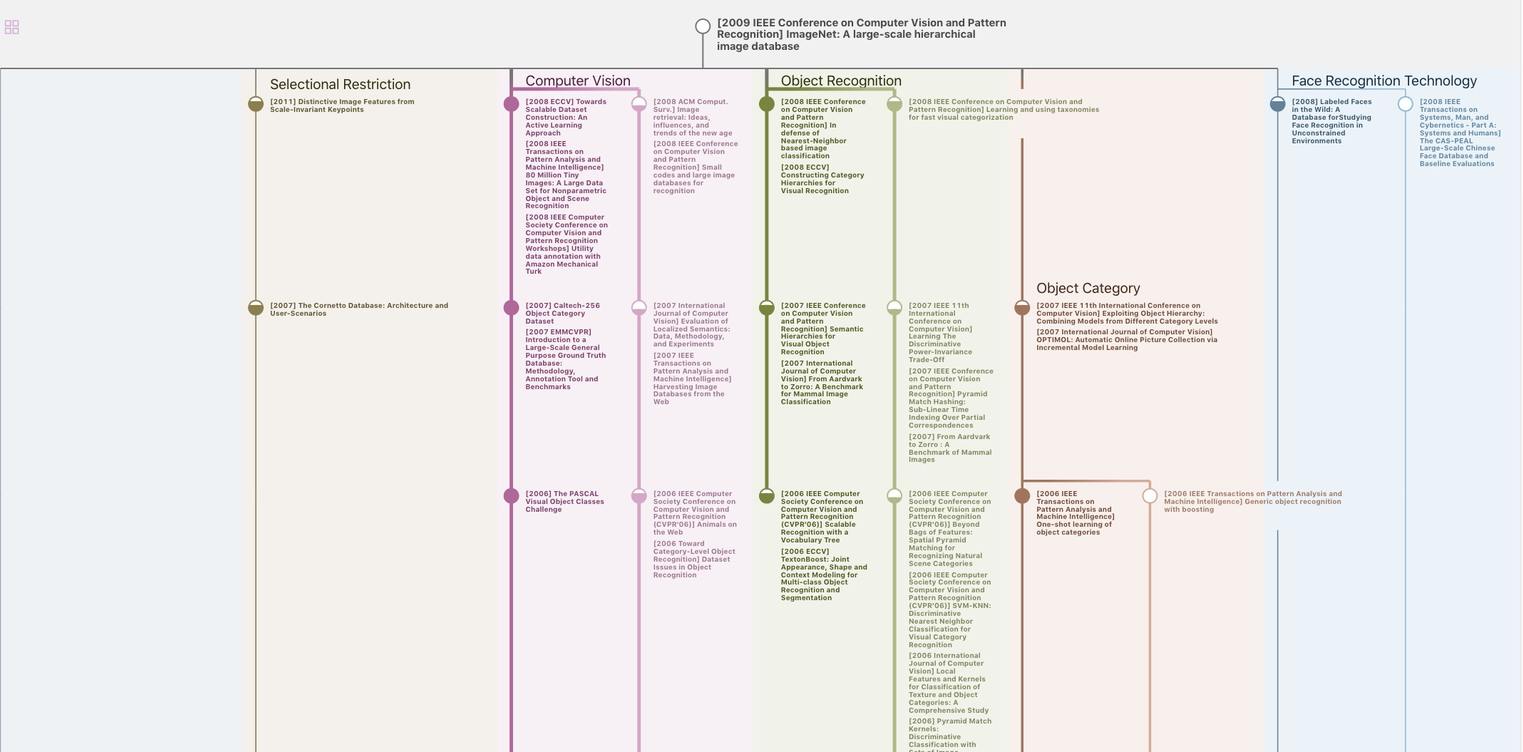
生成溯源树,研究论文发展脉络
Chat Paper
正在生成论文摘要