Crowdsourcing with Contextual Uncertainty
KDD '22: Proceedings of the 28th ACM SIGKDD Conference on Knowledge Discovery and Data Mining(2022)
摘要
We study a crowdsourcing setting where we need to infer the latent truth about a task given observed labels together with context in the form of a classifier score. We present Theodon, a hierarchical non-parametric Bayesian model, developed and deployed at Meta, that captures both the prevalence of label categories and the accuracy of labelers as functions of the classifier score. Theodon uses Gaussian processes to model the non-uniformity of mistakes over the range of classifier scores. For our experiments, we used data generated from integrity applications at Meta as well as public datasets. We showed that Theodon (1) obtains 1-4% improvement in AUC-PR predictions on items' true labels compared to state-of-the-art baselines for public datasets, (2) is effective as a calibration method, and (3) provides detailed insights on labelers' performances.
更多查看译文
关键词
uncertainty
AI 理解论文
溯源树
样例
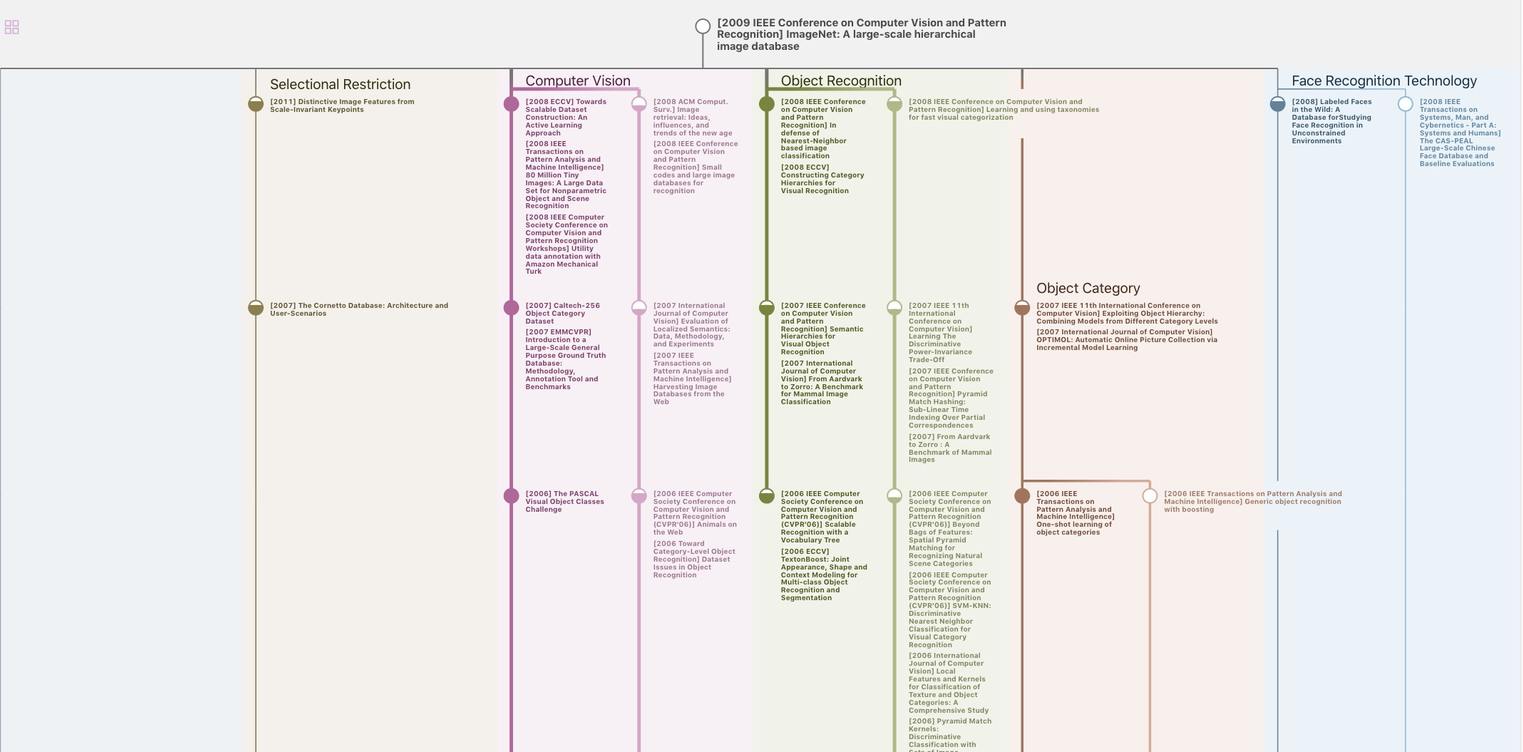
生成溯源树,研究论文发展脉络
Chat Paper
正在生成论文摘要