Unsupervised Learning Style Classification for Learning Path Generation in Online Education Platforms
KDD '22: Proceedings of the 28th ACM SIGKDD Conference on Knowledge Discovery and Data Mining(2022)
摘要
Online education, which educates students that cannot be present at school, has become an important supplement to traditional education. Without the direct supervision and instruction of teachers, online education is always concerned with potential distractions and misunderstandings. Learning Style Classification (LSC) is proposed to analyze the learning behavior patterns of online learning users, based on which personalized learning paths are generated to help them learn and maintain their interests. Existing LSC studies rely on expert-labored labeling, which is infeasible in large-scale applications, so we resort to unsupervised classification techniques. However, current unsupervised classification methods are not applicable due to two important challenges: C1) the unawareness of the LSC problem formulation and pedagogy domain knowledge; C2) the absence of any supervision signals. In this paper, we give a formal definition of the unsupervised LSC problem and summarize the domain knowledge into problem-solving heuristics (which addresses C1). A rule-based approach is first designed to provide a tentative solution in a principled manner (which addresses C2). On top of that, a novel Deep Unsupervised Classifier with domain Knowledge (DUCK) is proposed to convert the discovered conclusions and domain knowledge into learnable model components (which addresses both C1 and C2), which significantly improves the effectiveness, efficiency, and robustness. Extensive offline experiments on both public and industrial datasets demonstrate the superiority of our proposed methods. Moreover, the proposed methods are now deployed in the Huawei Education Center, and the ongoing A/B testing results verify the effectiveness of the methods.
更多查看译文
关键词
learning path generation,online education platforms,classification
AI 理解论文
溯源树
样例
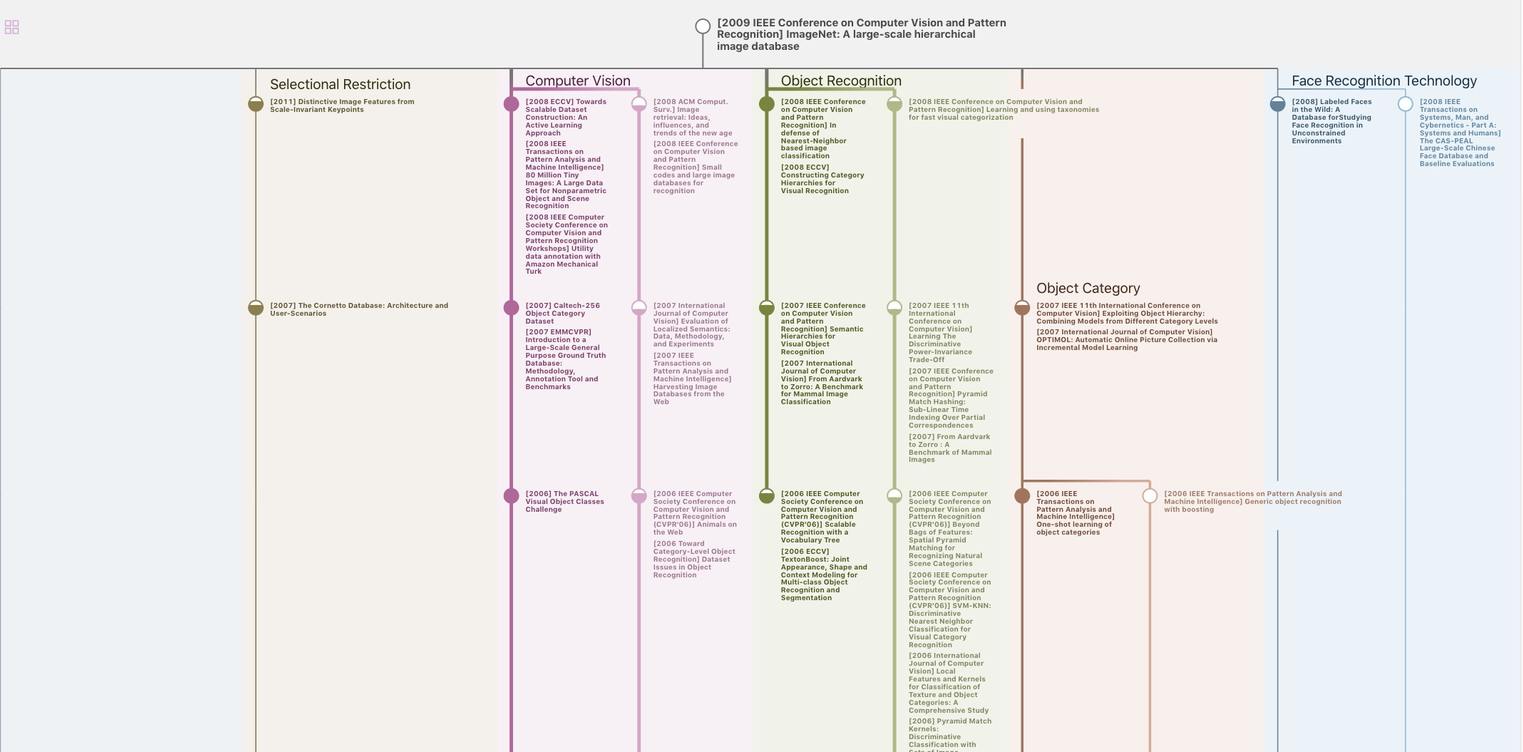
生成溯源树,研究论文发展脉络
Chat Paper
正在生成论文摘要